Comparison of Automatic Prostate Zones Segmentation Models in MRI Images Using U-net-like Architectures.
Mexican International Conference on Artificial Intelligence (MICAI)(2022)
摘要
Prostate cancer is the second-most frequently diagnosed cancer and the sixth leading cause of cancer death in males worldwide. The main problem that specialists face during the diagnosis of prostate cancer is the localization of Regions of Interest (ROI) containing a tumor tissue. Currently, the segmentation of this ROI in most cases is carried out manually by expert doctors, but the procedure is plagued with low detection rates (of about 27-44%) or overdiagnosis in some patients. Therefore, several research works have tackled the challenge of automatically segmenting and extracting features of the ROI from magnetic resonance images, as this process can greatly facilitate many diagnostic and therapeutic applications. However, the lack of clear prostate boundaries, the heterogeneity inherent to the prostate tissue, and the variety of prostate shapes makes this process very difficult to automate.In this work, six deep learning models were trained and analyzed with a dataset of MRI images obtained from the Centre Hospitalaire de Dijon and Universitat Politecnica de Catalunya. We carried out a comparison of multiple deep learning models (i.e. U-Net, Attention U-Net, Dense-UNet, Attention Dense-UNet, R2U-Net, and Attention R2U-Net) using categorical cross-entropy loss function. The analysis was performed using three metrics commonly used for image segmentation: Dice score, Jaccard index, and mean squared error. The model that give us the best result segmenting all the zones was R2U-Net, which achieved 0.869, 0.782, and 0.00013 for Dice, Jaccard and mean squared error, respectively.
更多查看译文
关键词
mri images,segmentation,prostate,u-net-like
AI 理解论文
溯源树
样例
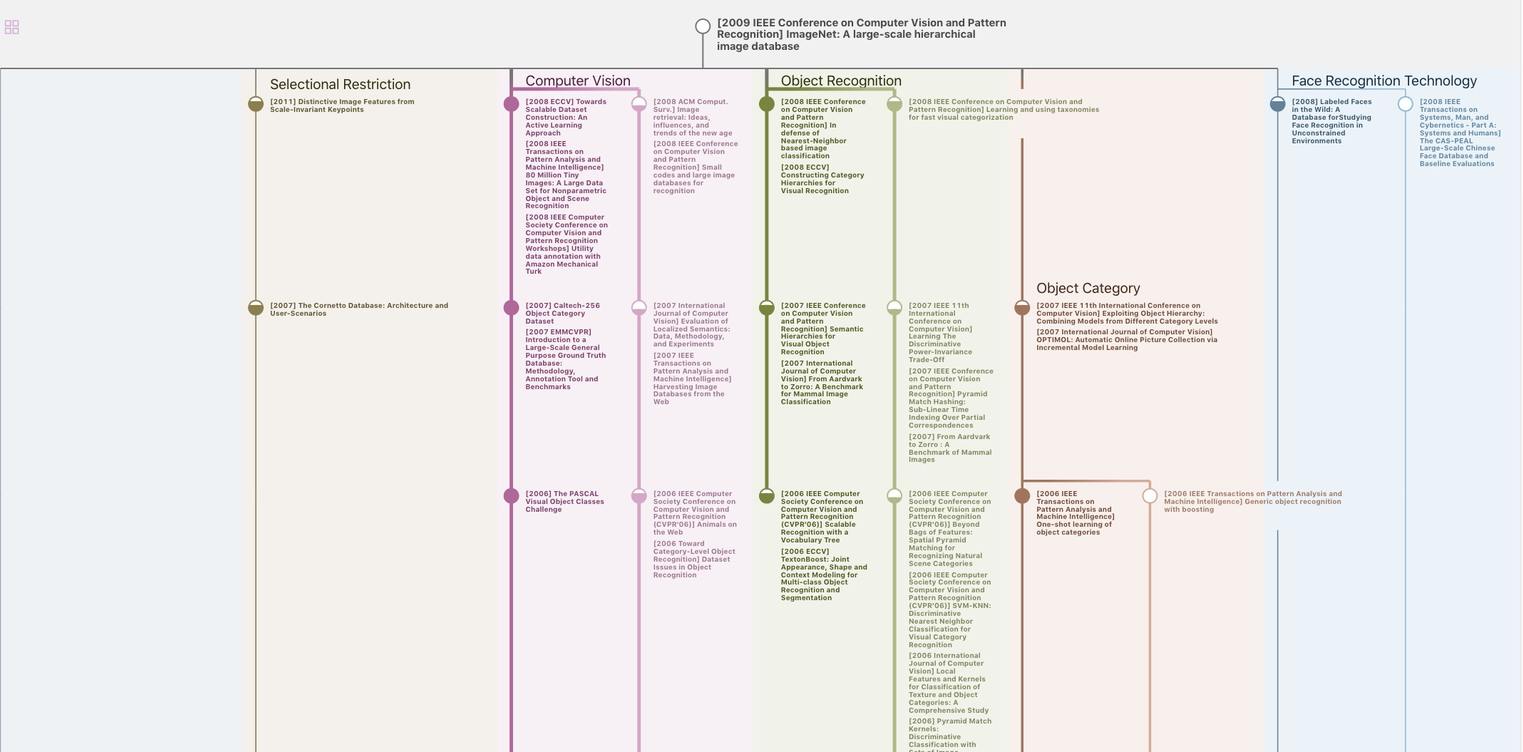
生成溯源树,研究论文发展脉络
Chat Paper
正在生成论文摘要