Two-stage method based on the you only look once framework and image segmentation for crack detection in concrete structures
Architecture, Structures and Construction(2023)
摘要
Detecting the presence of cracks and identifying their severity are crucial tasks for determining the structural health of a concrete building. In this study, we develop a two-stage automated method based on the You Only Look Once (YOLOv5) deep learning framework for the identification, localization, and quantification of cracks in the concrete structures. In the first stage, cracks are identified and localized using bounding boxes, while in the second stage, the length of cracks and, therefore, the damage severity are determined. The developed deep learning model is trained using 4500 annotated images from a total of 40000 images of size 227 × 227 pixel, which are obtained from an open-source dataset collected at various campus buildings of Middle East Technical University (METU). The concept of transfer learning (i.e., pre-trained weights) is used for the training, which drastically reduces the training time. The detection and localization accuracy of this model is measured in terms of the average precision, average recall, and F1-score. The YOLOv5 model achieves the mean average precision (mAP_0.5) of 95.02 % . A ResNet model is also developed just to capture the supremacy of the YOLOv5 model. The proposed method can help in identifying structural anomalies through real-time monitoring that must be urgently repaired and thus can be used in high-quality civil infrastructure monitoring systems.
更多查看译文
关键词
Deep learning, Structural health monitoring, Convolutional neural networks, Crack detection, Damage detection, Computer vision
AI 理解论文
溯源树
样例
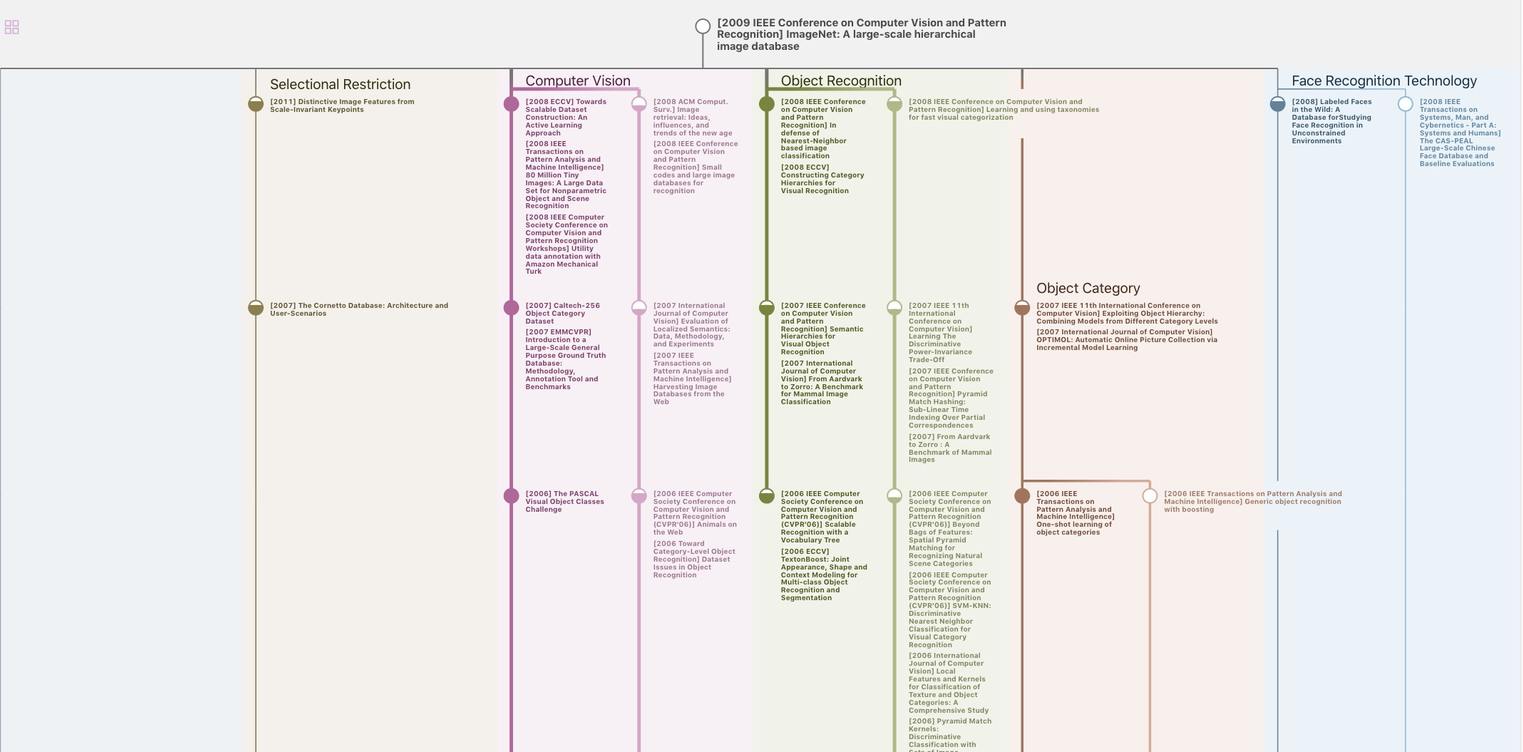
生成溯源树,研究论文发展脉络
Chat Paper
正在生成论文摘要