eCDT: Event Clustering for Simultaneous Feature Detection and Tracking.
IEEE/RJS International Conference on Intelligent RObots and Systems (IROS)(2022)
摘要
Contrary to other standard cameras, event cameras interpret the world in an entirely different manner; as a collection of asynchronous events. Despite event camera's unique data output, many event feature detection and tracking algorithms have shown significant progress by making detours to frame-based data representations. This paper questions the need to do so and proposes a novel event data-friendly method that achieve simultaneous feature detection and tracking, called event Clustering-based Detection and Tracking (eCDT). Our method employs a novel clustering method, named as k-NN Classifier-based Spatial Clustering and Applications with Noise (KCSCAN), to cluster adjacent polarity events to retrieve event trajectories.With the aid of a Head and Tail Descriptor Matching process, event clusters that reappear in a different polarity are continually tracked, elongating the feature tracks. Thanks to our clustering approach in spatio-temporal space, our method automatically solves feature detection and feature tracking simultaneously. Also, eCDT can extract feature tracks at any frequency with an adjustable time window, which does not corrupt the high temporal resolution of the original event data. Our method achieves 30% better feature tracking ages compared with the state-of-the-art approach while also having a low error approximately equal to it.
更多查看译文
关键词
simultaneous feature detection,tracking,clustering,event
AI 理解论文
溯源树
样例
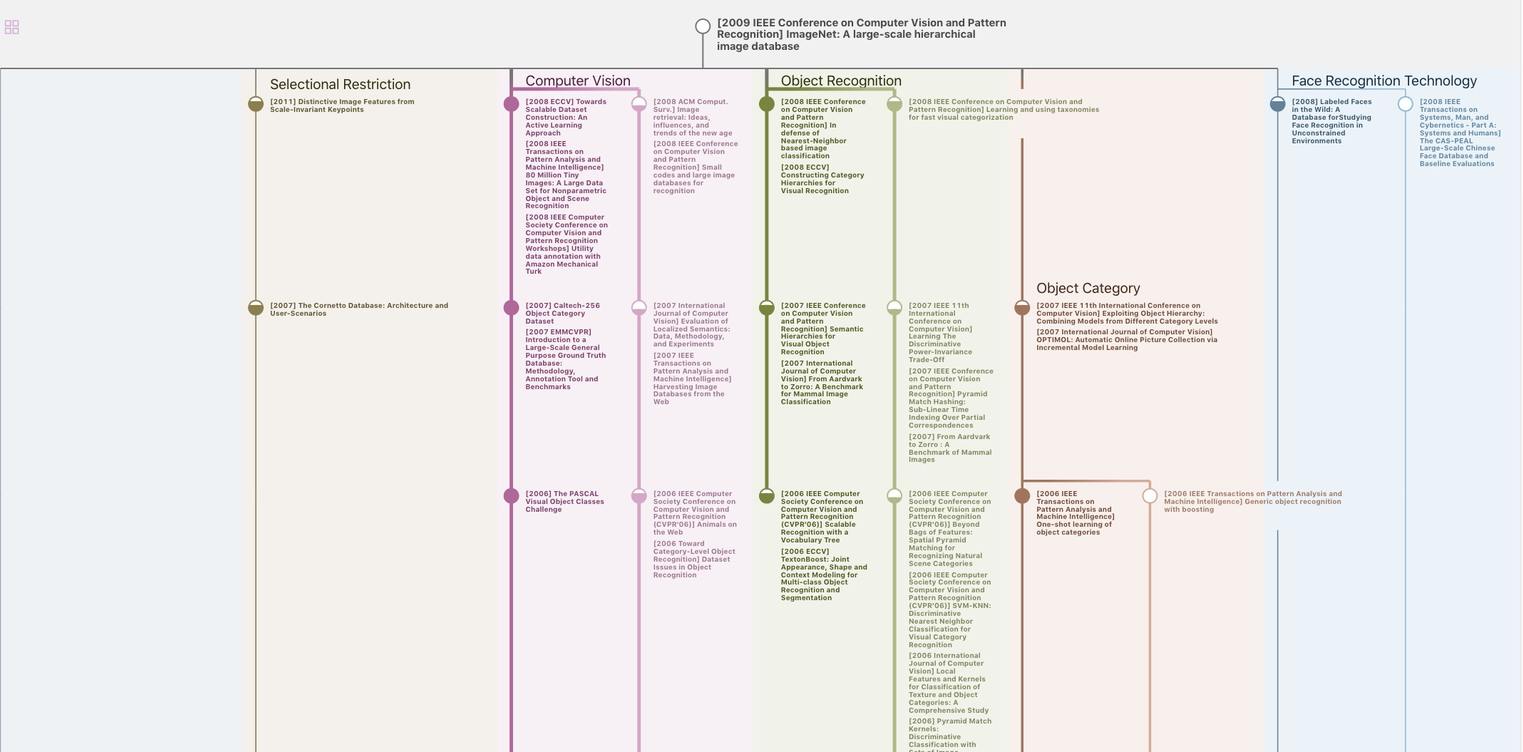
生成溯源树,研究论文发展脉络
Chat Paper
正在生成论文摘要