ILASR: Privacy-Preserving Incremental Learning for Automatic Speech Recognition at Production Scale
KDD '22: Proceedings of the 28th ACM SIGKDD Conference on Knowledge Discovery and Data Mining(2022)
摘要
Incremental learning is one paradigm to enable model building and updating at scale with streaming data. For end-to-end automatic speech recognition (ASR) tasks, the absence of human annotated labels along with the need for privacy preserving policies for model building makes it a daunting challenge. Motivated by these challenges, in this paper we use a cloud based framework for production systems to demonstrate insights from privacy preserving incremental learning for automatic speech recognition (ILASR). By privacy preserving, we mean, usage of ephemeral data which are not human annotated. This system is a step forward for production level ASR models for incremental/continual learning that offers near real-time test-bed for experimentation in the cloud for end-to-end ASR, while adhering to privacy-preserving policies. We show that the proposed system can improve the production models significantly ($3%$) over a new time period of six months even in the absence of human annotated labels with varying levels of weak supervision and large batch sizes in incremental learning. This improvement is $20%$ over test sets with new words and phrases in the new time period. We demonstrate the effectiveness of model building in a privacy-preserving incremental fashion for ASR while further exploring the utility of having an effective teacher model and use of large batch sizes.
更多查看译文
关键词
automatic speech recognition,learning,ilasr,privacy-preserving
AI 理解论文
溯源树
样例
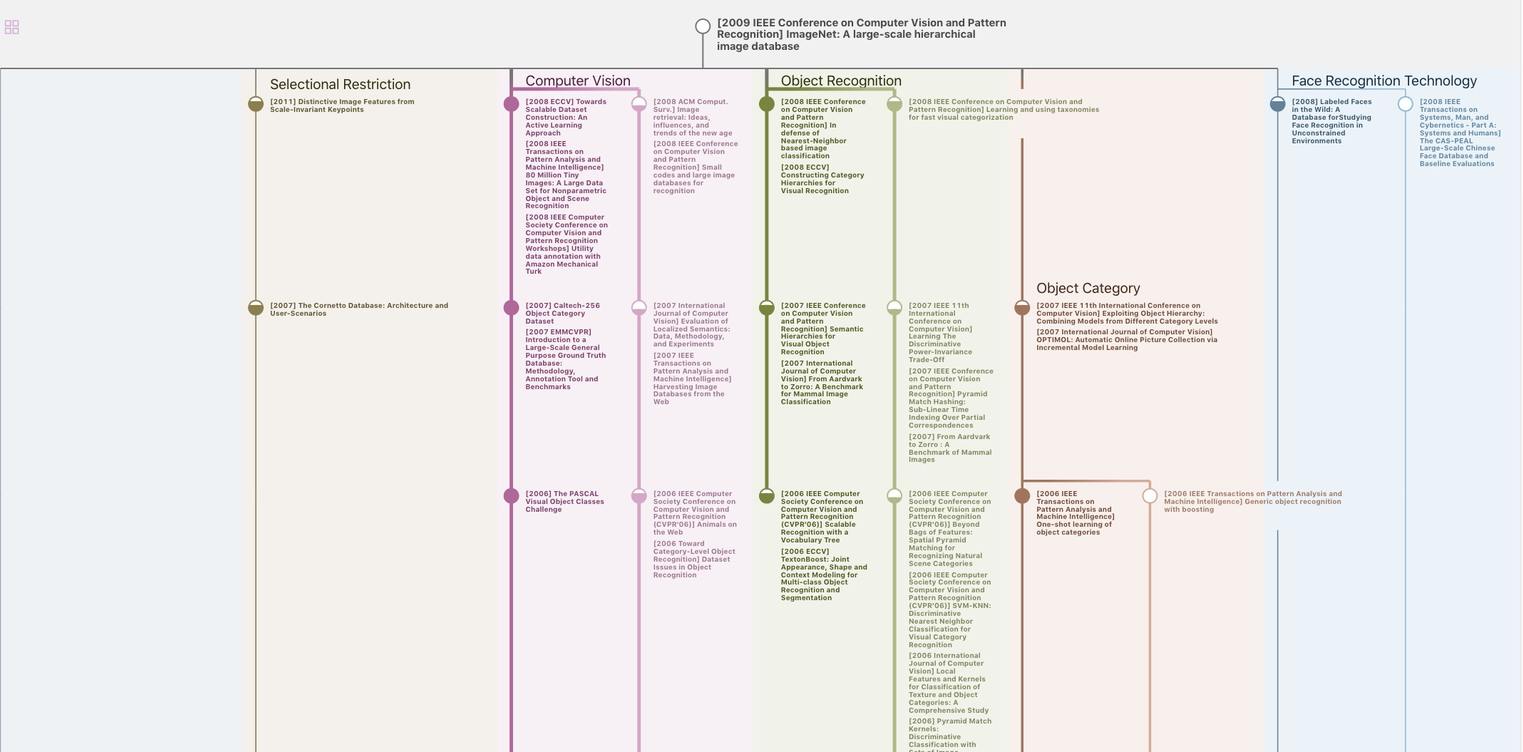
生成溯源树,研究论文发展脉络
Chat Paper
正在生成论文摘要