Boosting Video Super Resolution with Patch-Based Temporal Redundancy Optimization
ARTIFICIAL NEURAL NETWORKS AND MACHINE LEARNING, ICANN 2023, PT VII(2023)
摘要
The success of existing video super-resolution (VSR) algorithms stems mainly exploiting the temporal information from the neighboring frames. However, none of these methods have discussed the influence of the temporal redundancy in the patches with stationary objects and background and usually use all the information in the adjacent frames without any discrimination. In this paper, we observe that the temporal redundancy will bring adverse effect to the information propagation, which limits the performance of the most existing VSR methods and causes the severe generalization problem. Motivated by this observation, we aim to improve existing VSR algorithms by handling the temporal redundancy patches in an optimized manner. We develop two simple yet effective plug-and-play methods to improve the performance and the generalization ability of existing local and non-local propagation-based VSR algorithms on widely-used public videos. For more comprehensive evaluating the robustness and performance of existing VSR algorithms, we also collect a new dataset which contains a variety of public videos as testing set. Extensive evaluations show that the proposed methods can significantly improve the performance and the generalization ability of existing VSR methods on the collected videos from wild scenarios while maintain their performance on existing commonly used datasets.
更多查看译文
关键词
video super-resolution,temporal redundancy,plug and play methods
AI 理解论文
溯源树
样例
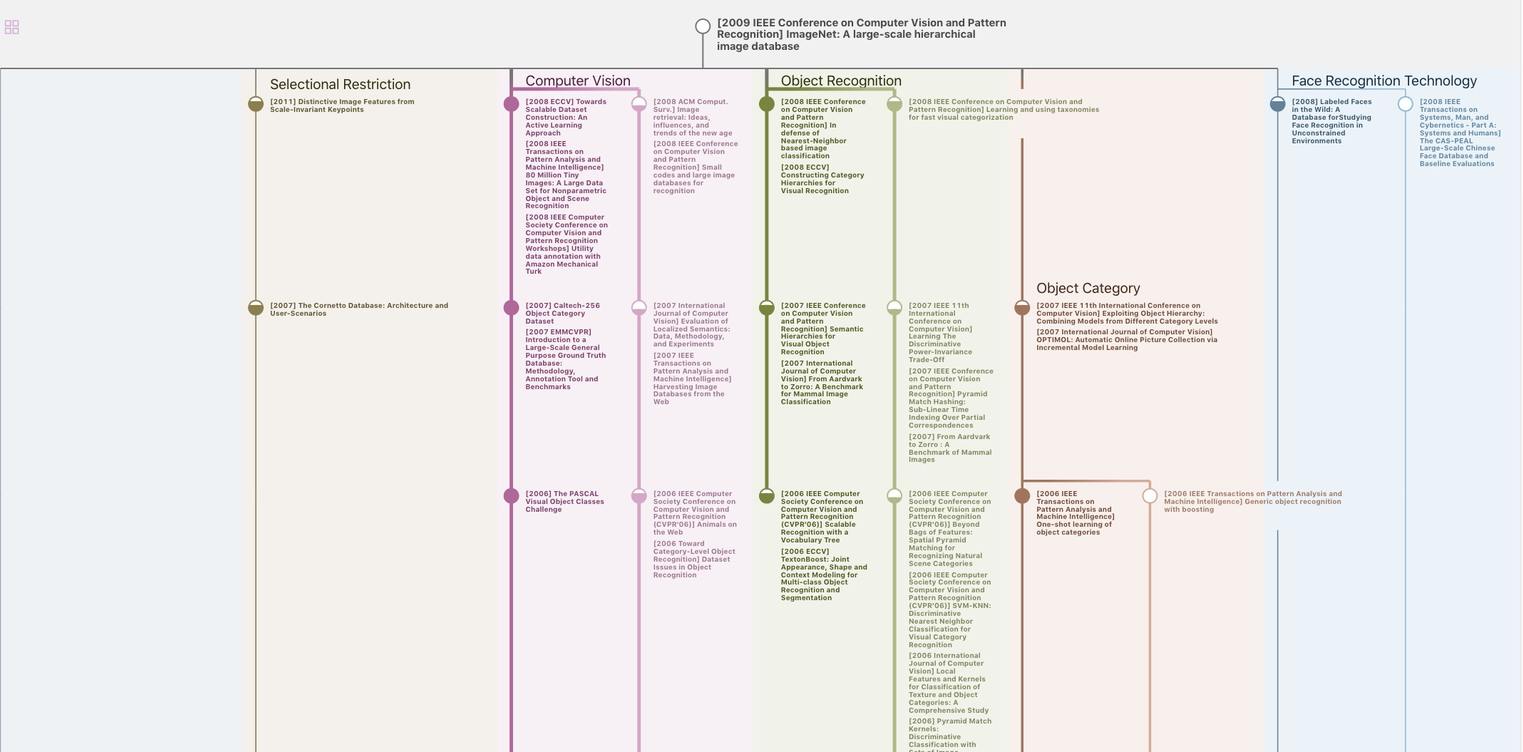
生成溯源树,研究论文发展脉络
Chat Paper
正在生成论文摘要