Learning Correspondency in Frequency Domain by a Latent-Space Similarity Loss for Multispectral Pansharpening
arxiv(2022)
摘要
The process of fusing a high spatial resolution (HR) panchromatic (PAN) image and a low spatial resolution (LR) multispectral (MS) image to obtain an HRMS image is known as pansharpening. With the development of convolutional neural networks, the performance of pansharpening methods has been improved, however, the blurry effects and the spectral distortion still exist in their fusion results due to the insufficiency in details learning and the mismatch between the high-frequency (HF) and low-frequency (LF) components. Therefore, the improvements of spatial details at the premise of reducing spectral distortion is still a challenge. In this paper, we propose a frequency-aware network (FAN) together with a novel latent-space similarity loss to address above mentioned problems. FAN is composed of three modules, where the frequency feature extraction module aims to extract features in the frequency domain with the help of discrete wavelet transform (DWT) layers, and the inverse DWT (IDWT) layers are then utilized in the frequency feature fusion module to reconstruct the features. Finally, the fusion results are obtained through the reconstruction module. In order to learn the correspondency, we also propose a latent-space similarity loss to constrain the LF features derived from PAN and MS branches, so that HF features of PAN can reasonably be used to supplement that of MS. Experimental results on three datasets at both reduced- and full-resolution demonstrate the superiority of the proposed method compared with several state-of-the-art pansharpening models, especially for the fusion at full resolution.
更多查看译文
关键词
multispectral pansharpening,learning,frequency domain,similarity
AI 理解论文
溯源树
样例
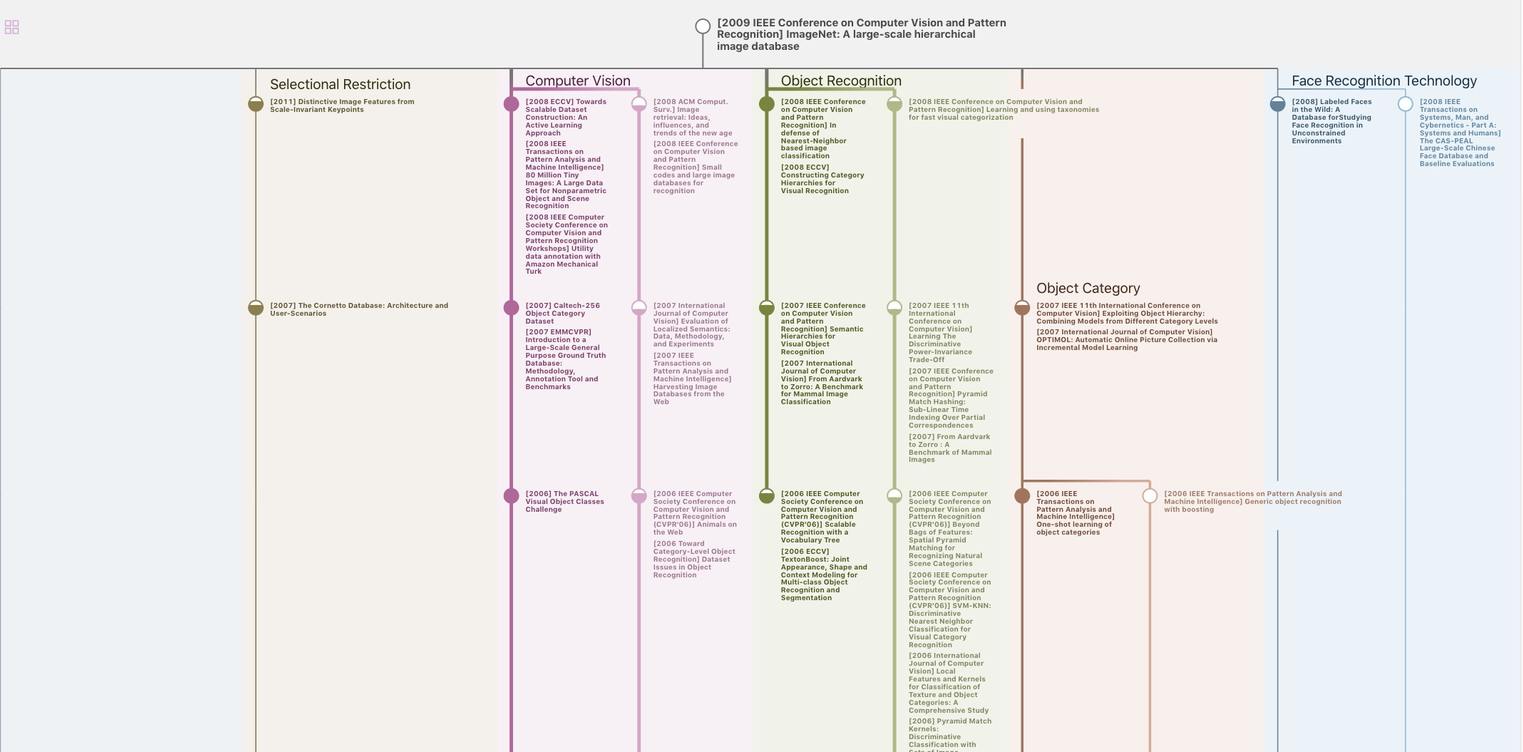
生成溯源树,研究论文发展脉络
Chat Paper
正在生成论文摘要