Bayesian Optimization for Macro Placement
arxiv(2022)
摘要
Macro placement is the problem of placing memory blocks on a chip canvas. It can be formulated as a combinatorial optimization problem over sequence pairs, a representation which describes the relative positions of macros. Solving this problem is particularly challenging since the objective function is expensive to evaluate. In this paper, we develop a novel approach to macro placement using Bayesian optimization (BO) over sequence pairs. BO is a machine learning technique that uses a probabilistic surrogate model and an acquisition function that balances exploration and exploitation to efficiently optimize a black-box objective function. BO is more sample-efficient than reinforcement learning and therefore can be used with more realistic objectives. Additionally, the ability to learn from data and adapt the algorithm to the objective function makes BO an appealing alternative to other black-box optimization methods such as simulated annealing, which relies on problem-dependent heuristics and parameter-tuning. We benchmark our algorithm on the fixed-outline macro placement problem with the half-perimeter wire length objective and demonstrate competitive performance.
更多查看译文
关键词
bayesian optimization,placement,macro
AI 理解论文
溯源树
样例
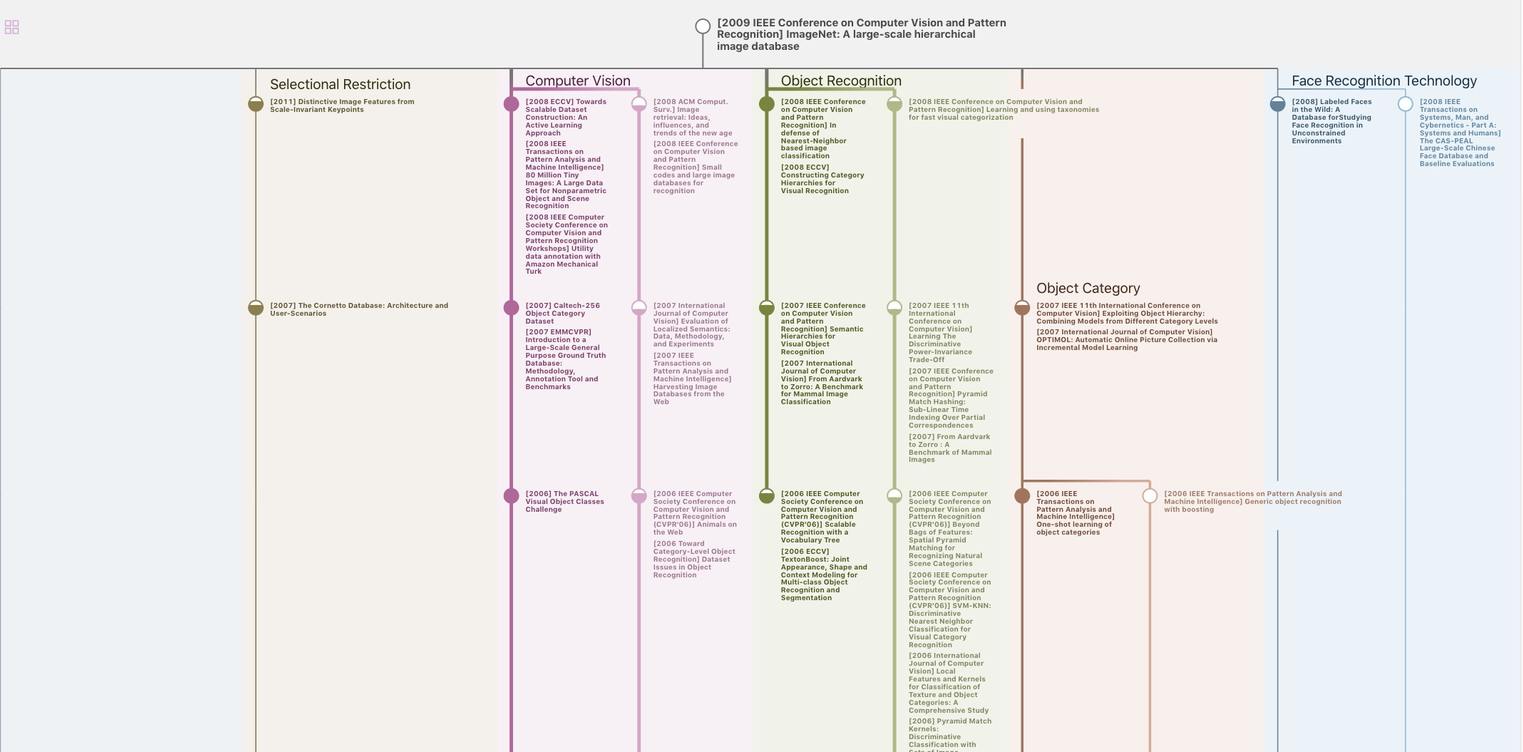
生成溯源树,研究论文发展脉络
Chat Paper
正在生成论文摘要