Online Prediction in Sub-linear Space.
SODA(2023)
摘要
We provide the first sub-linear space and sub-linear regret algorithm for online learning with expert advice (against an oblivious adversary), addressing an open question raised recently by Srinivas, Woodruff, Xu and Zhou (STOC 2022). We also demonstrate a separation between oblivious and (strong) adaptive adversaries by proving a linear memory lower bound of any sub-linear regret algorithm against an adaptive adversary. Our algorithm is based on a novel pool selection procedure that bypasses the traditional wisdom of leader selection for online learning, and a generic reduction that transforms any weakly sub-linear regret $o(T)$ algorithm to $T^{1-\alpha}$ regret algorithm, which may be of independent interest. Our lower bound utilizes the connection of no-regret learning and equilibrium computation in zero-sum games, leading to a proof of a strong lower bound against an adaptive adversary.
更多查看译文
关键词
prediction,sub-linear
AI 理解论文
溯源树
样例
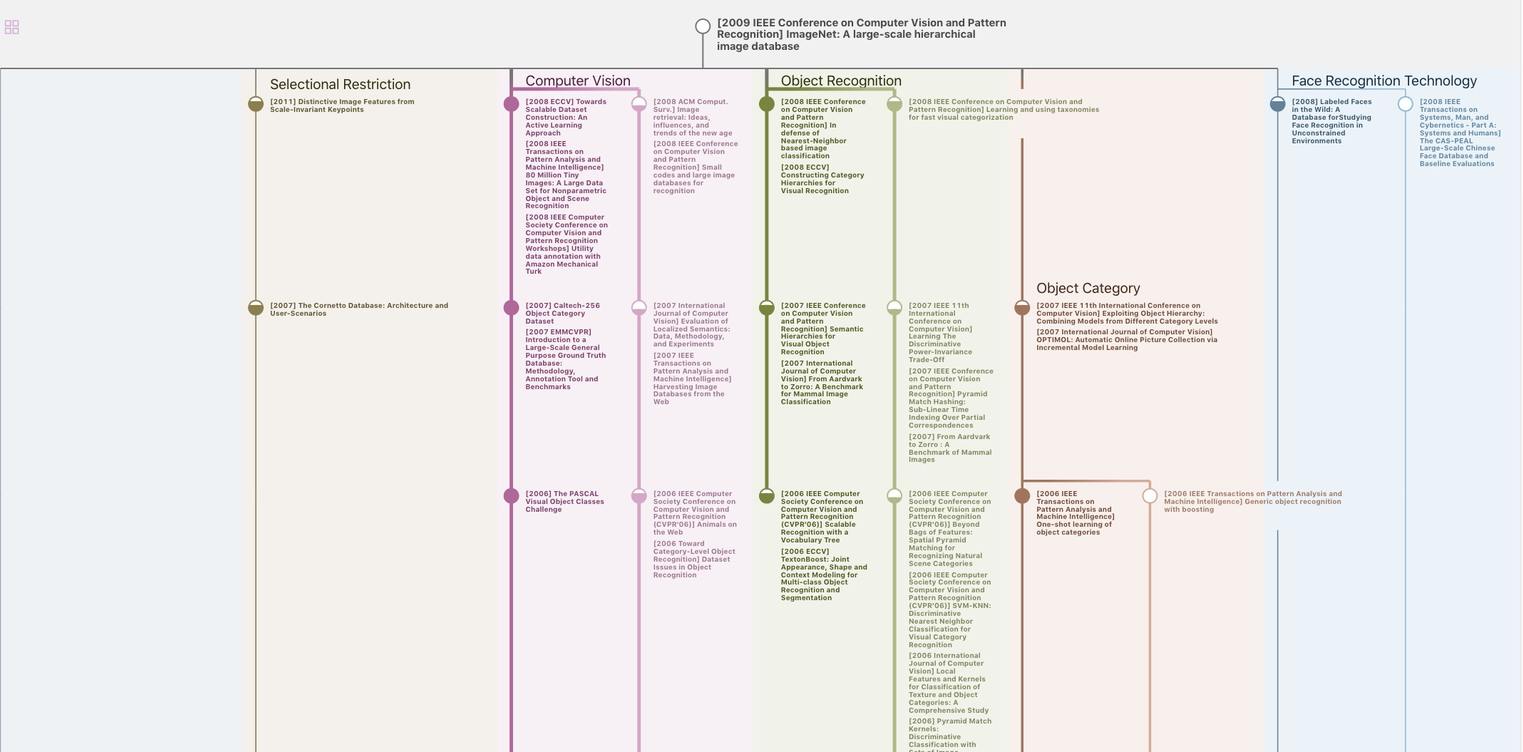
生成溯源树,研究论文发展脉络
Chat Paper
正在生成论文摘要