Algebraic Algorithms for Fractional Linear Matroid Parity via Non-commutative Rank.
SODA(2023)
摘要
Matrix representations are a powerful tool for designing efficient algorithms for combinatorial optimization problems such as matching, and linear matroid intersection and parity. In this paper, we initiate the study of matrix representations using the concept of non-commutative rank (nc-rank), which has recently attracted attention in the research of Edmonds' problem. We reveal that the nc-rank of the matrix representation of linear matroid parity corresponds to the optimal value of fractional linear matroid parity: a half-integral relaxation of linear matroid parity. Based on our representation, we present an algebraic algorithm for the fractional linear matroid parity problem by building a new technique to incorporate the search-to-decision reduction into the half-integral problem represented via the nc-rank. We further present a faster divide-and-conquer algorithm for finding a maximum fractional matroid matching and an algebraic algorithm for finding a dual optimal solution. They together lead to an algebraic algorithm for the weighted fractional linear matroid parity problem. Our algorithms are significantly simpler and faster than the existing algorithms.
更多查看译文
关键词
fractional linear matroid parity,algebraic,algorithms,rank,non-commutative
AI 理解论文
溯源树
样例
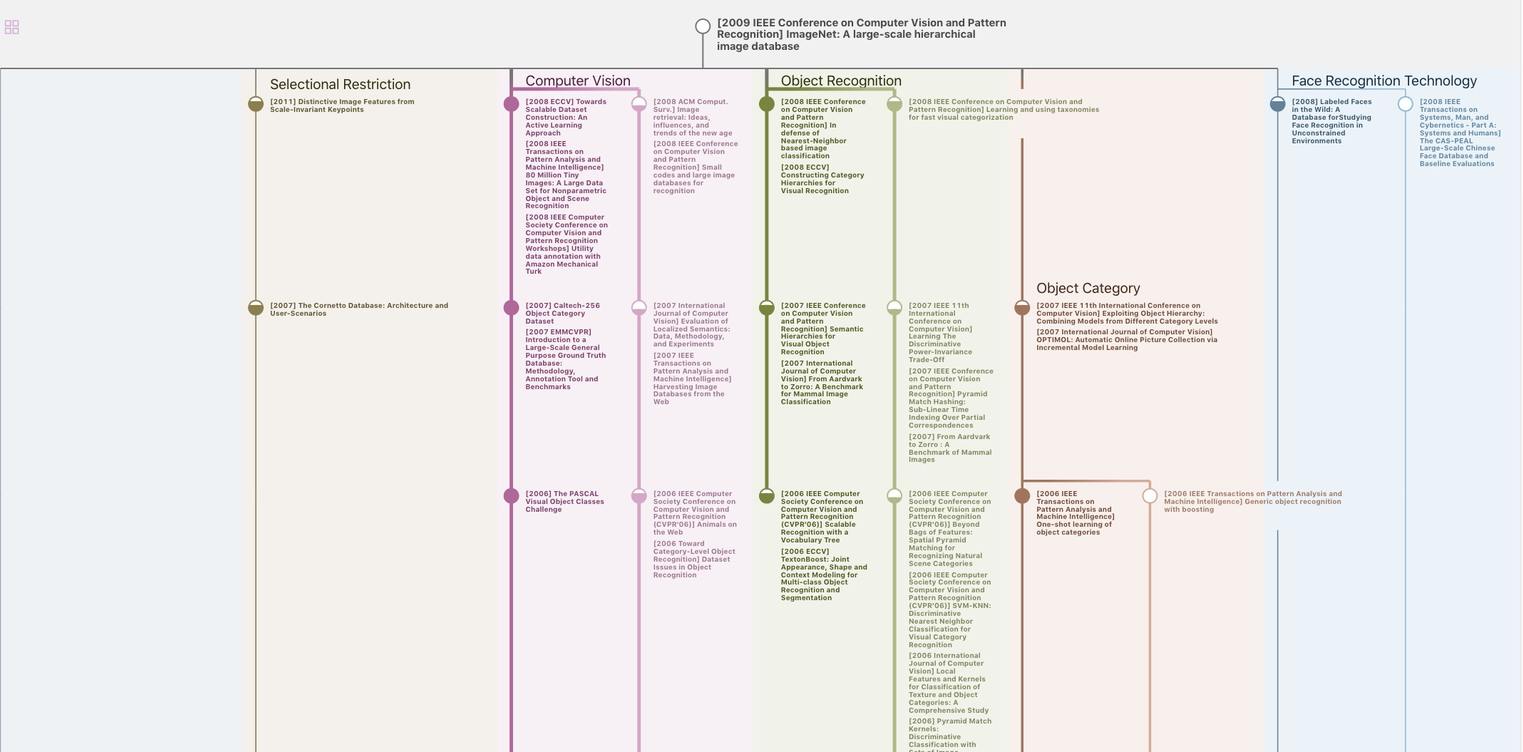
生成溯源树,研究论文发展脉络
Chat Paper
正在生成论文摘要