Treatment Heterogeneity for Survival Outcomes
arxiv(2022)
摘要
Estimation of conditional average treatment effects (CATEs) plays an essential role in modern medicine by informing treatment decision-making at a patient level. Several metalearners have been proposed recently to estimate CATEs in an effective and flexible way by re-purposing predictive machine learning models for causal estimation. In this chapter, we summarize the literature on metalearners and provide concrete guidance for their application for treatment heterogeneity estimation from randomized controlled trials' data with survival outcomes. The guidance we provide is supported by a comprehensive simulation study in which we vary the complexity of the underlying baseline risk and CATE functions, the magnitude of the heterogeneity in the treatment effect, the censoring mechanism, and the balance in treatment assignment. To demonstrate the applicability of our findings, we reanalyze the data from the Systolic Blood Pressure Intervention Trial (SPRINT) and the Action to Control Cardiovascular Risk in Diabetes (ACCORD) study. While recent literature reports the existence of heterogeneous effects of intensive blood pressure treatment with multiple treatment effect modifiers, our results suggest that many of these modifiers may be spurious discoveries. This chapter is accompanied by survlearners, an R package that provides well-documented implementations of the CATE estimation strategies described in this work, to allow easy use of our recommendations as well as the reproduction of our numerical study.
更多查看译文
关键词
outcomes,survival,treatment
AI 理解论文
溯源树
样例
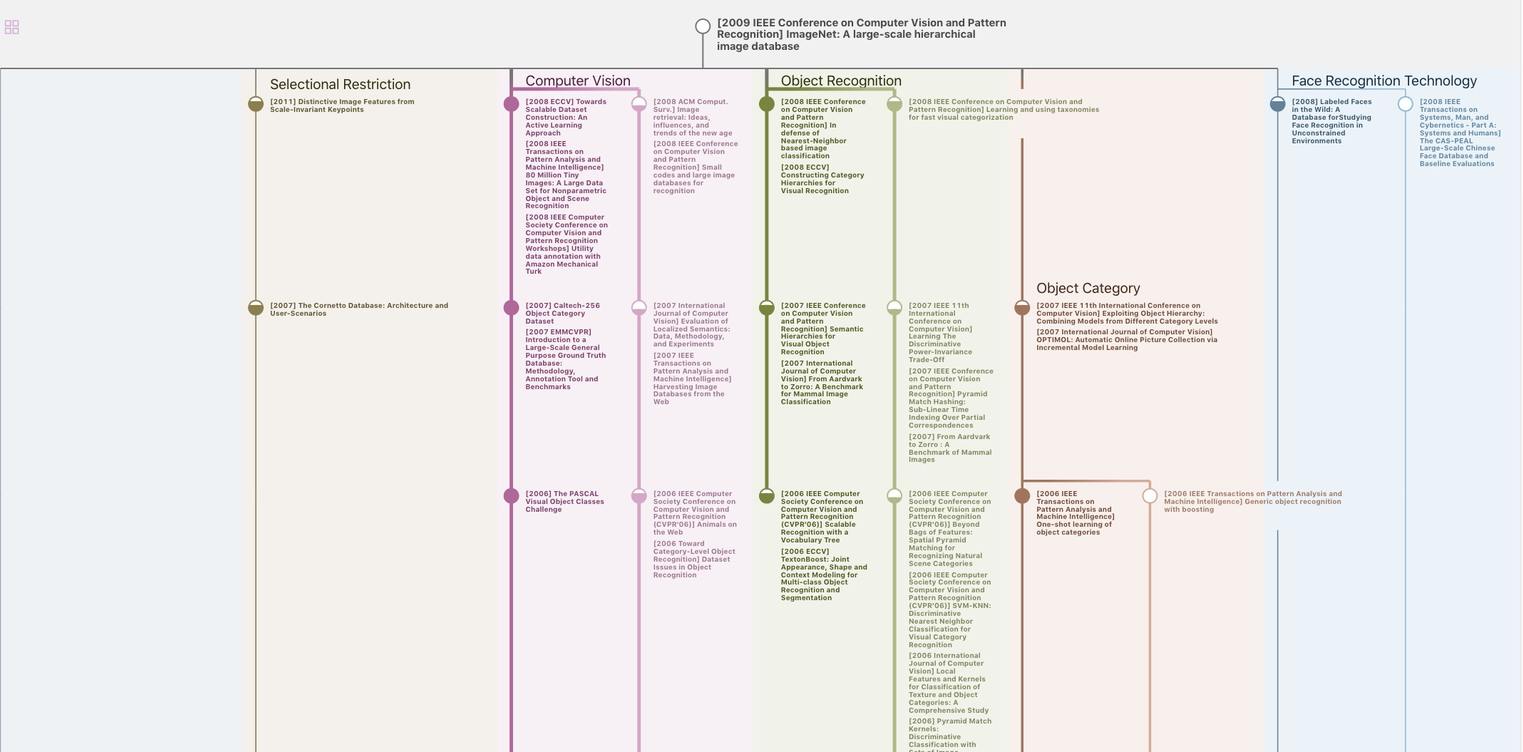
生成溯源树,研究论文发展脉络
Chat Paper
正在生成论文摘要