Partial Disentanglement via Mechanism Sparsity
arxiv(2022)
摘要
Disentanglement via mechanism sparsity was introduced recently as a principled approach to extract latent factors without supervision when the causal graph relating them in time is sparse, and/or when actions are observed and affect them sparsely. However, this theory applies only to ground-truth graphs satisfying a specific criterion. In this work, we introduce a generalization of this theory which applies to any ground-truth graph and specifies qualitatively how disentangled the learned representation is expected to be, via a new equivalence relation over models we call consistency. This equivalence captures which factors are expected to remain entangled and which are not based on the specific form of the ground-truth graph. We call this weaker form of identifiability partial disentanglement. The graphical criterion that allows complete disentanglement, proposed in an earlier work, can be derived as a special case of our theory. Finally, we enforce graph sparsity with constrained optimization and illustrate our theory and algorithm in simulations.
更多查看译文
关键词
partial disentanglement,mechanism sparsity
AI 理解论文
溯源树
样例
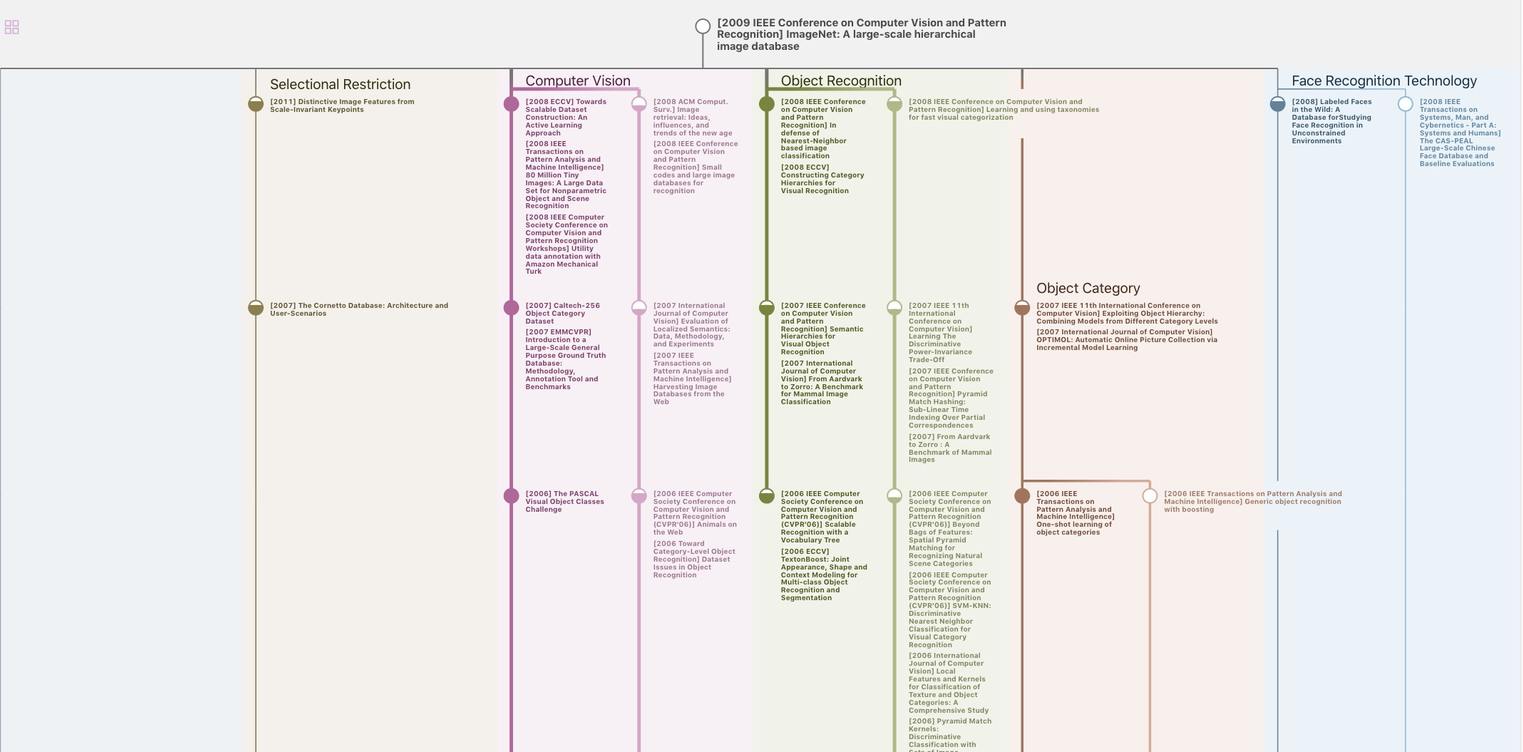
生成溯源树,研究论文发展脉络
Chat Paper
正在生成论文摘要