Using genetic algorithms to identify deleterious patterns of physiologic data for near real-time prediction of mortality in critically ill patients
Informatics in Medicine Unlocked(2021)
摘要
Objective: Contemporary predictive models of mortality for adult critically ill patients are not suitable for use at the bedside. Almost all have been developed and then tested using retrospective, cleansed data, which does not give an accurate assessment of how the models will function under actual situations. We previously reported on a promising predictive model called SIGNIPHY that used genetic algorithms to identify patterns that were precursors of patient decline in an intensive care unit (ICU). But it was based on retrospective data that had been post-processed. This study looked at how genetic algorithms could partition streaming physiologic data into patterns that translated into a near real-time, accurate, prediction of mortality in adult ICUs. Methods: Patients admitted to six ICUs had their vital signs data collected continuously and in near real-time for three months in 2020. A genetic algorithm partitioned the distribution of each vital sign into bins that conferred the greatest variation in mortality rate. “Letters” were assigned to each bin, which were then mapped to a patient's median value for each vital sign during a 10-min time segment. Concatenating letters from three time segments resulted in “words” that were proxies for unique physiologic trends over 30 min. Weights were assigned to these physiologic trends, as well as whether or not the patient was receiving mechanical ventilation, to give each patient a rolling score for every time period: rSIGNIPHY. A genetic algorithm optimized the randomly assigned weights into those that maximized the association between a patient's highest rSIGNIPHY score and risk of death. Results: 1810 patients had data collected and an rSIGNIPHY score computed at each 30-min time segment. A patient's highest rSIGNIPHY score was strongly associated with future mortality, with an R2 of 0.981 for a second-order polynomial model. We then partitioned the maximum rSIGNIPHY score into three “risk bins”, labelled low risk, moderate risk, and high risk. The respective morality rates for those three groups were 3.2%, 18.8%, and 33.3%. Conclusion: The use of genetic algorithms to identify specific physiologic patterns associated with mortality was tested using live, real-time data collected from critically ill patients. Although retrospectively analyzing the data, rSIGNIPHY proved successful in assigning patients to risk groups: low, moderate, and high. The high-risk group had a mortality rate that was 10.5-fold higher than that seen in the low-risk patient group, and increasing values of a patient's maximum rSIGNIPHY score were strongly associated with increasing mortality. Moving forward, implementation of rSIGNIPHY to prospectively assign mortality risk to patients in near real-time could lead to improved treatment of critically ill patients in a timely manner.
更多查看译文
关键词
Critical care,Intensive care units,Mortality prediction,Genetic algorithms,Optimization
AI 理解论文
溯源树
样例
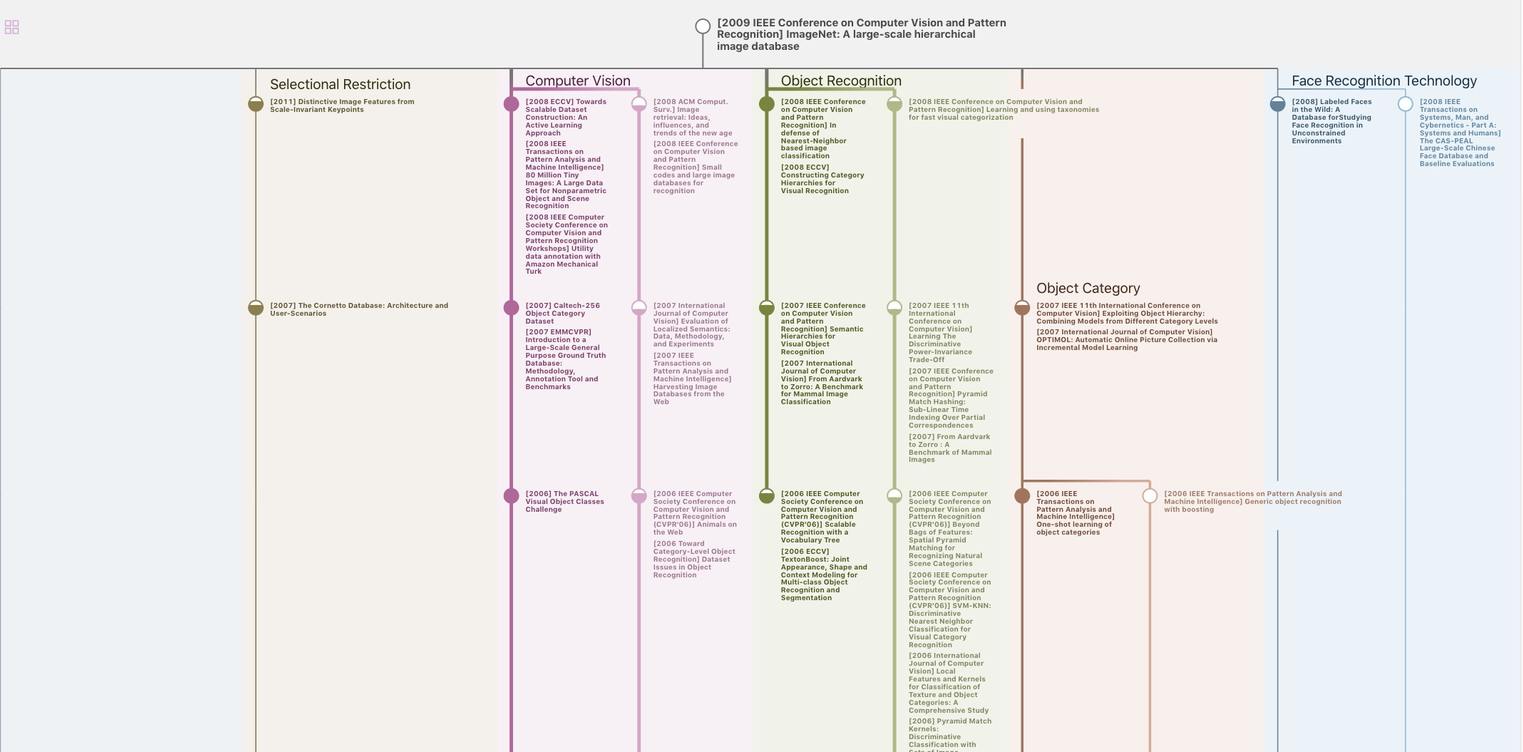
生成溯源树,研究论文发展脉络
Chat Paper
正在生成论文摘要