The benefits of adversarial defense in generalization
Neurocomputing(2022)
摘要
Recent research has shown that models induced by machine learning, and in particular by deep learning, can be easily fooled by an adversary who carefully crafts imperceptible, at least from the human perspective, or physically plausible modifications of the input data. This discovery gave birth to a new field of research, the adversarial machine learning, where new methods of attacks and defense are developed continuously, mimicking what is happening from a long time in cybersecurity. In this paper we will show that the drawbacks of inducing models from data less prone to be misled can actually provide some benefits when it comes to assessing their generalization abilities. We will show these benefits both from a theoretical perspective, using state-of-the-art statistical learning theory, and both with practical examples.
更多查看译文
关键词
Adversarial machine learning,Evasion attacks,Adversarial defense,Statistical learning theory,Generalization,(Local) Vapnik–Chervonenkis theory,(Local) Rademacher complexity theory
AI 理解论文
溯源树
样例
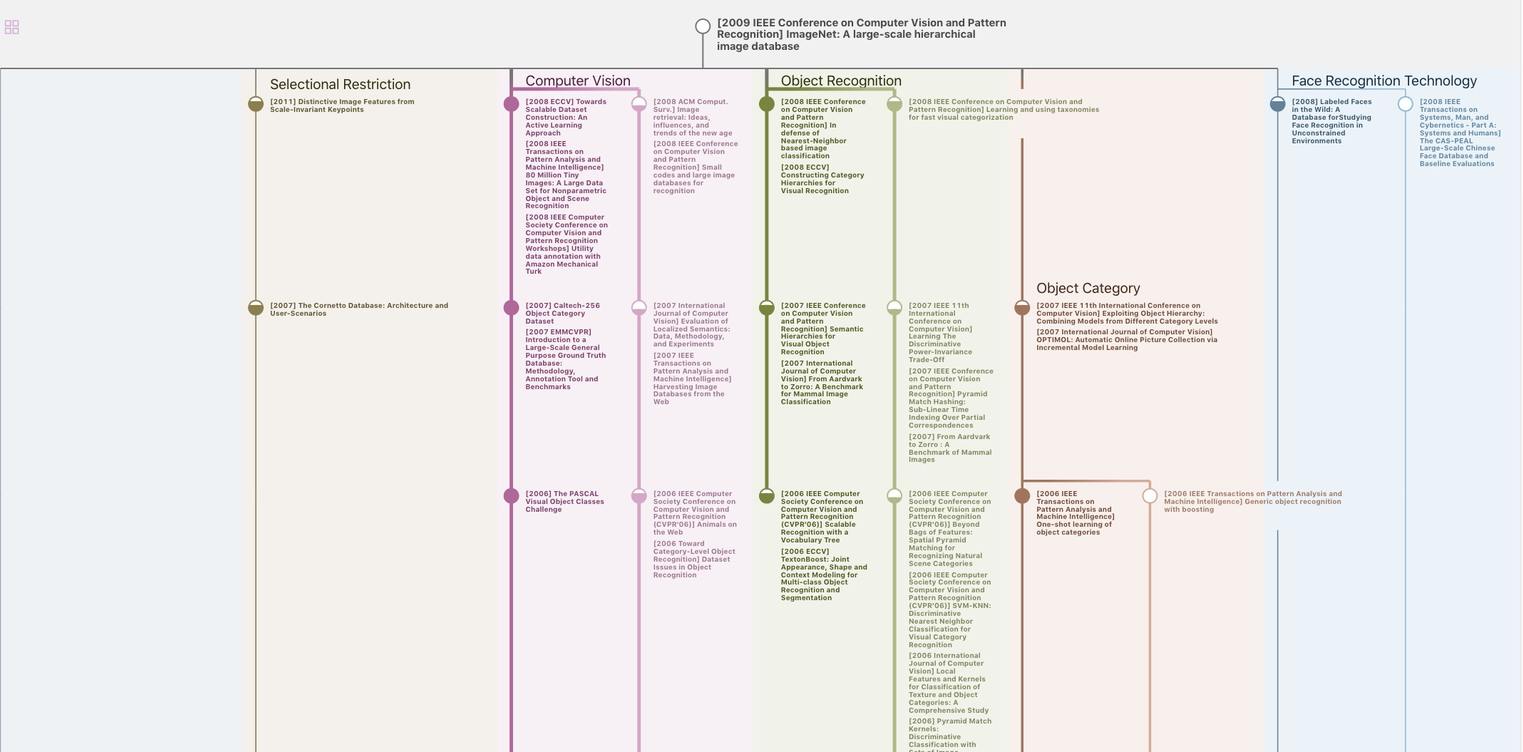
生成溯源树,研究论文发展脉络
Chat Paper
正在生成论文摘要