A soft-computing based approach to overlapped cells analysis in histopathology images with genetic algorithm
Applied Soft Computing(2022)
摘要
Deep learning methods have shown great significance for medical image processing in recent years, including radiography, magnetic resonance imaging, nuclear medicine, and histopathology. Learning-based methods help to detect the target patterns when the network is well-trained. However, the gap between detected patterns and clinical decisions is still enormous, and soft-computing methods could help to improve the decisions based on the specific features of the problem. In this paper, the target is to detect the immunohistochemistry (IHC) positive cells in lymphoma histopathology images. We use a deep learning network to detect the positive cells in pixel level, and results show the proposed residual U-Net structure outperforms the baseline models. In the failure cases, they mainly occur when two cells are overlapped in predicted masks, which is the drawback of learning-based methods. Hence, a soft-computing based method is proposed to use ellipse formulation to represent a cellular pattern, and use genetic algorithm (GA) to determine an optimal decision of overlapped ellipses. We use two datasets: 36 images with ground truth from pathologists’ annotation, and 43 images with overlapped cells (cropped from failed predictions). A series of experiments show that the proposed deep learning model could detect most of IHC-positive cells in the proposed dataset, and our soft-computing method could detect 86.04% of the overlapped cells and the proposed GA outperforms the baseline methods.
更多查看译文
关键词
Lymphoma histopathology images,Cell detection,Overlapped cells,Genetic algorithm,Deep learning
AI 理解论文
溯源树
样例
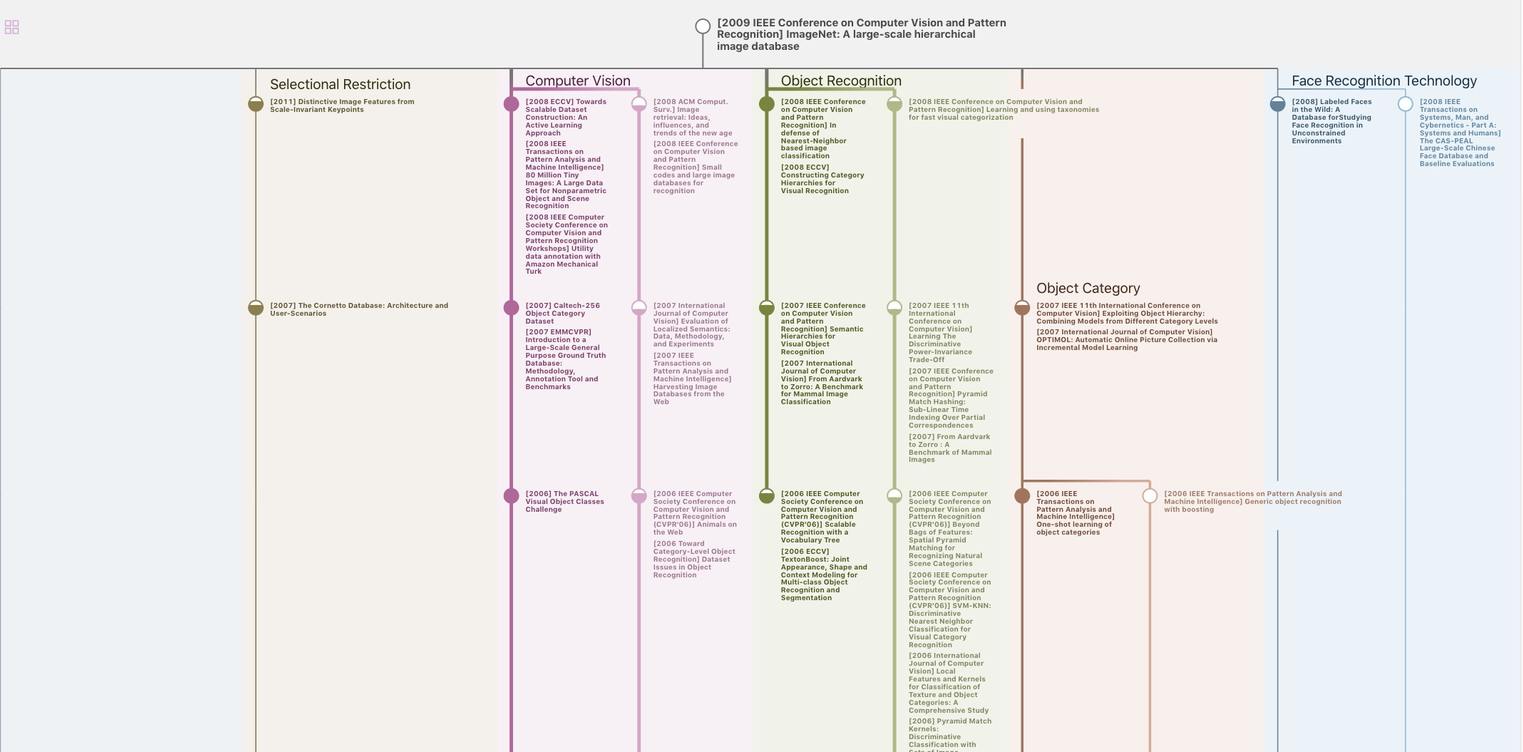
生成溯源树,研究论文发展脉络
Chat Paper
正在生成论文摘要