MINIMAX ESTIMATION OF SMOOTH DENSITIES IN WASSERSTEIN DISTANCE
ANNALS OF STATISTICS(2022)
摘要
We study nonparametric density estimation problems where error is measured in the Wasserstein distance, a metric on probability distributions popular in many areas of statistics and machine learning. We give the first minimax-optimal rates for this problem for general Wasserstein distances for two classes of densities: smooth probability densities on [0, 1](d) bounded away from 0, and sub-Gaussian densities lying in the Holder class C-s, s is an element of (0, 1). Unlike classical nonparametric density estimation, these rates depend on whether the densities in question are bounded below, even in the compactly supported case. Motivated by variational problems involving the Wasserstein distance, we also show how to construct discretely supported measures, suitable for computational purposes, which achieve the minimax rates. Our main technical tool is an inequality giving a nearly tight dual characterization of the Wasserstein distances in terms of Besov norms.
更多查看译文
关键词
Wasserstein distance, optimal transport, high-dimensional statistics, density estimation
AI 理解论文
溯源树
样例
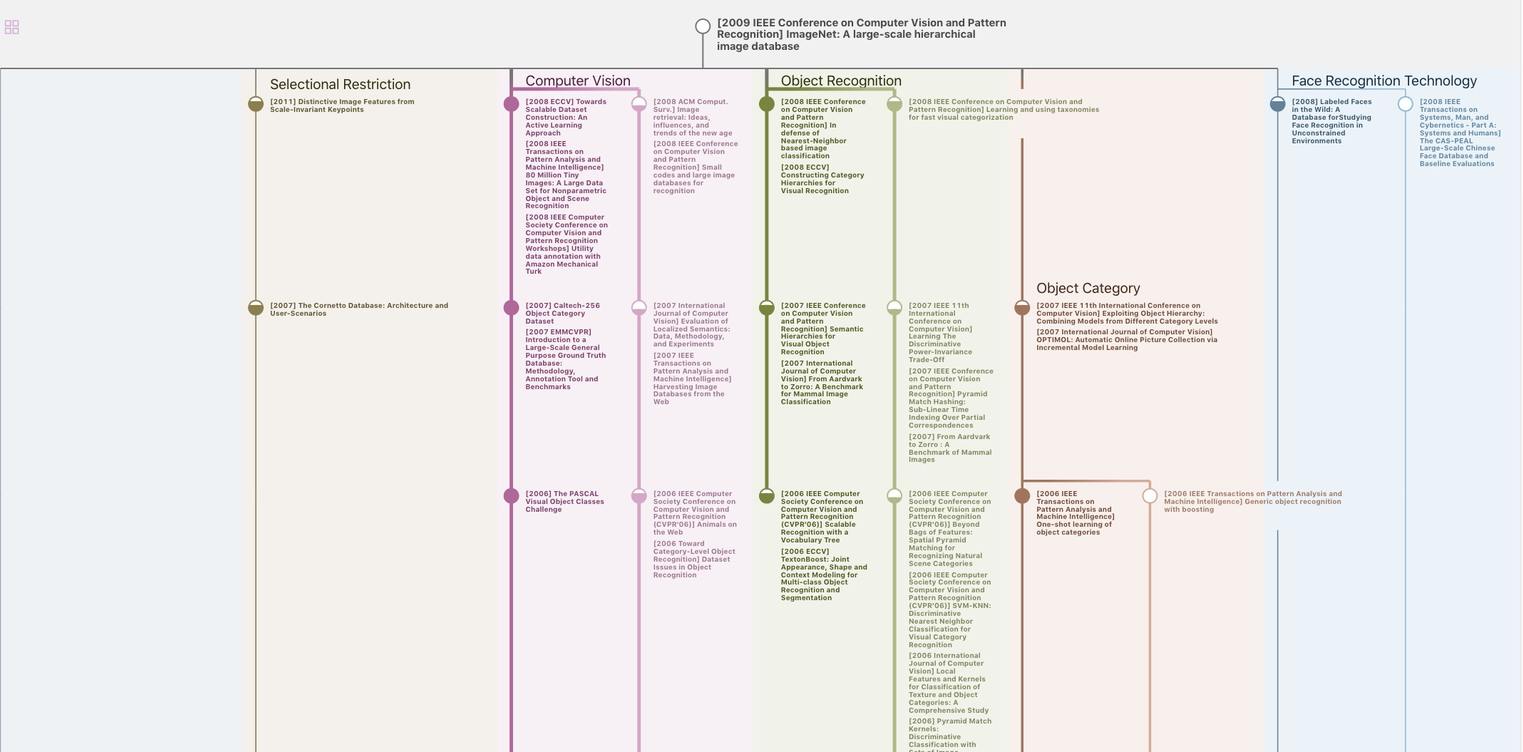
生成溯源树,研究论文发展脉络
Chat Paper
正在生成论文摘要