Active contour model of breast cancer DCE-MRI segmentation with an extreme learning machine and a fuzzy C-means cluster
IET IMAGE PROCESSING(2022)
摘要
Due to the low contrast, blurred boundary and intensity inhomogeneity of the images, accurate segmentation of breast cancer lesions with dynamic contrast-enhanced magnetic resonance imaging (DCE-MRI) still has great challenges. This paper proposed an improved active contour model (ACM) for segmenting breast cancer lesions in DCE-MRI images. First, based on the extreme learning machine (ELM) method, a robust function is proposed that combines image intensities and time-domain features to enhance the difference between the lesions and other tissues. Second, an edge-stop function (ESF) is introduced by combining the image intensity, time-domain feature, and Hessian shape index to detect the irregular and blurred boundaries. At the boundary of breast cancer lesions, the energy function of ACM is minimized and the evolution of the contour curve completes, so the accurate lesion region of breast cancer can be segmented. The mean Dice similar coefficient (DICE), Jaccard similarity (JC) and Hausdorff distance (HD) of the segmentation of the proposed model in 50 samples are 85.88 +/- 6.62%, 75.72 +/- 9.68% and 11.62 +/- 4.72 mm, respectively. The results segmented by the proposed ACM are more similar to the manual segmentation than the compared models.
更多查看译文
AI 理解论文
溯源树
样例
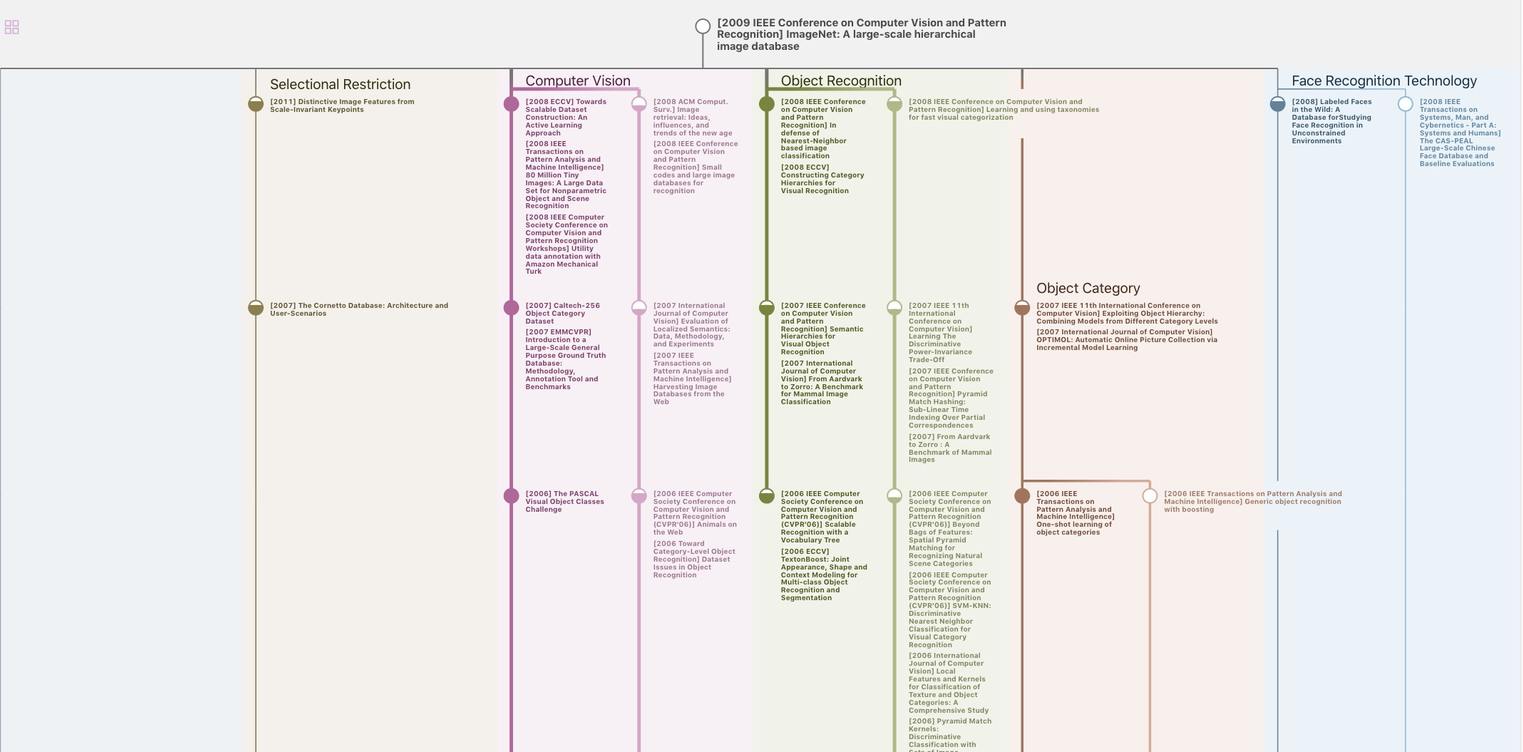
生成溯源树,研究论文发展脉络
Chat Paper
正在生成论文摘要