Including parameterized error covariance in local ensemble solvers: Experiments in a 1D model with balance constraints
QUARTERLY JOURNAL OF THE ROYAL METEOROLOGICAL SOCIETY(2022)
摘要
Lack of efficient ways to include parameterized error covariance in ensemble-based local volume solvers (e.g. the local ensemble-transform Kalman filter - the LETKF) remains an outstanding problem in data assimilation. Here, we describe two new algorithms: GETKF-OI and LETKF-OI. These algorithms are similar to the traditional optimal interpolation (OI) algorithm in that they use parameterized error covariance to update each of the local volume solutions. However, unlike the traditional OI that scales poorly as the number of observations increases, the new algorithms achieve linear scalability by using either the observational-space localization strategy of the traditional LETKF algorithm or the modulated ensembles of the gain-form (GETKF) algorithm. In our testing with a simple one-dimensional univariate system, we find that the GETKF-OI algorithm can recover the exact solution within the truncation bounds of the modulated ensemble and the LETKF-OI algorithm achieves a close approximation to the exact solution. We also demonstrate how to extend GETKF-OI algorithm to a toy multivariate system with balance constraints.
更多查看译文
关键词
tools and methods, data assimilation, optimal interpolation, LETKF
AI 理解论文
溯源树
样例
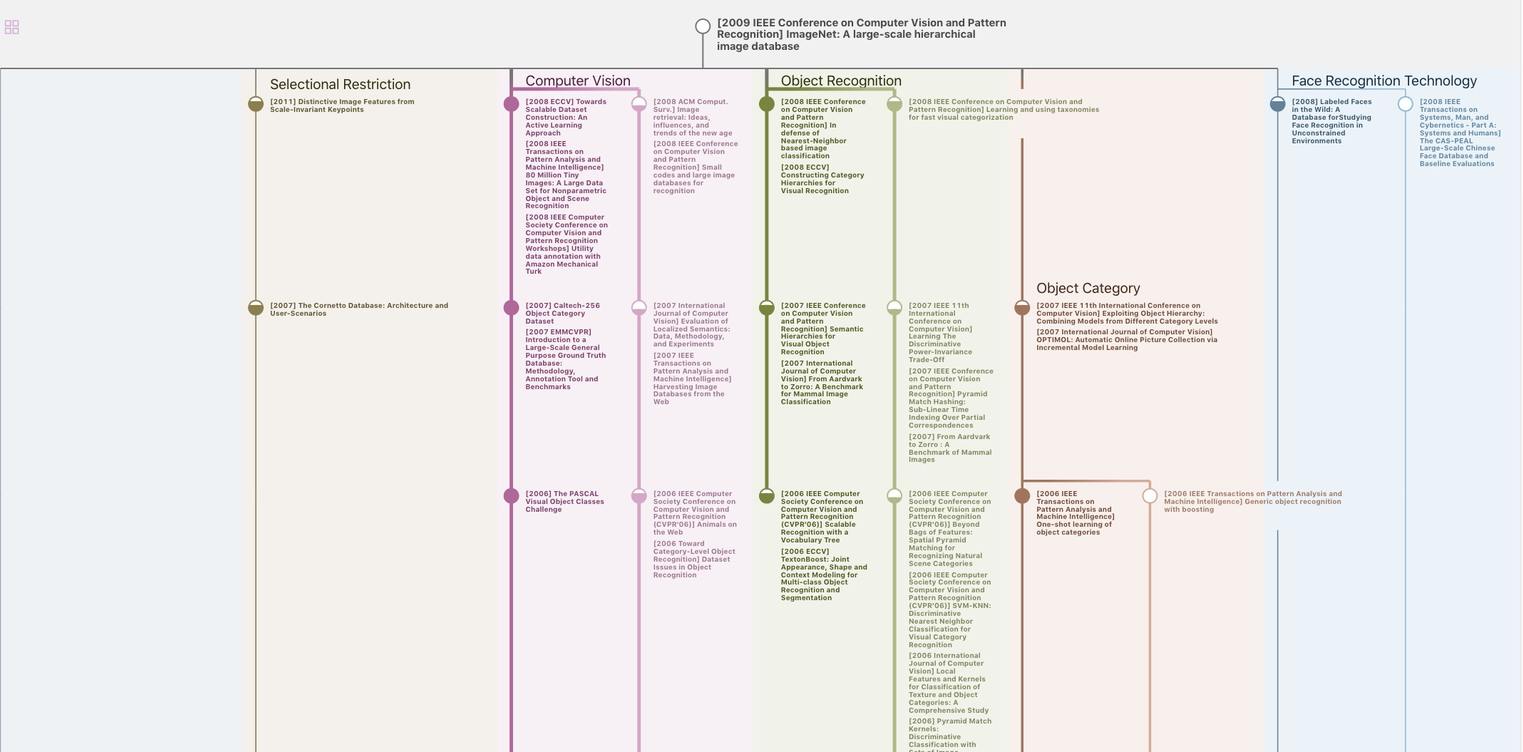
生成溯源树,研究论文发展脉络
Chat Paper
正在生成论文摘要