Convolutional Shrinkage Neural Networks Based Model-Agnostic Meta-Learning for Few-Shot Learning
NEURAL PROCESSING LETTERS(2022)
摘要
Meta Learning (ML) has the ability to quickly learn from a small number of samples, and has become an important research field after reinforcement learning. However, the complexity of sample features severely reduces the performance of few-shot learning, and proper feature selection plays a vital role in the performance of neural networks. To address this problem, this article draws up a new type of convolutional neural network with an attention mechanism, namely, convolutional shrinkage neural networks (CSNNs), using the characteristics of negligible noise to obtain a good optimization parameter model. Moreover, soft thresholding is inserted into the network architectures as nonlinear transformation layers to eliminate nonessential features. In addition, considering that it is difficult to set appropriate values for the thresholds, the developed convolutional shrinkage neural networks integrates some specialized neural networks into trainable modules to automatically set the thresholds. To illustrate the effectiveness of the proposed method, the model-agnostic meta-learning method is considered for testing. The results show that the improved method can significantly improve the accuracy of few-shot images classification and enhance the generalization performance.
更多查看译文
关键词
Meta learning, Few-shot learning, Residual networks, Soft thresholding
AI 理解论文
溯源树
样例
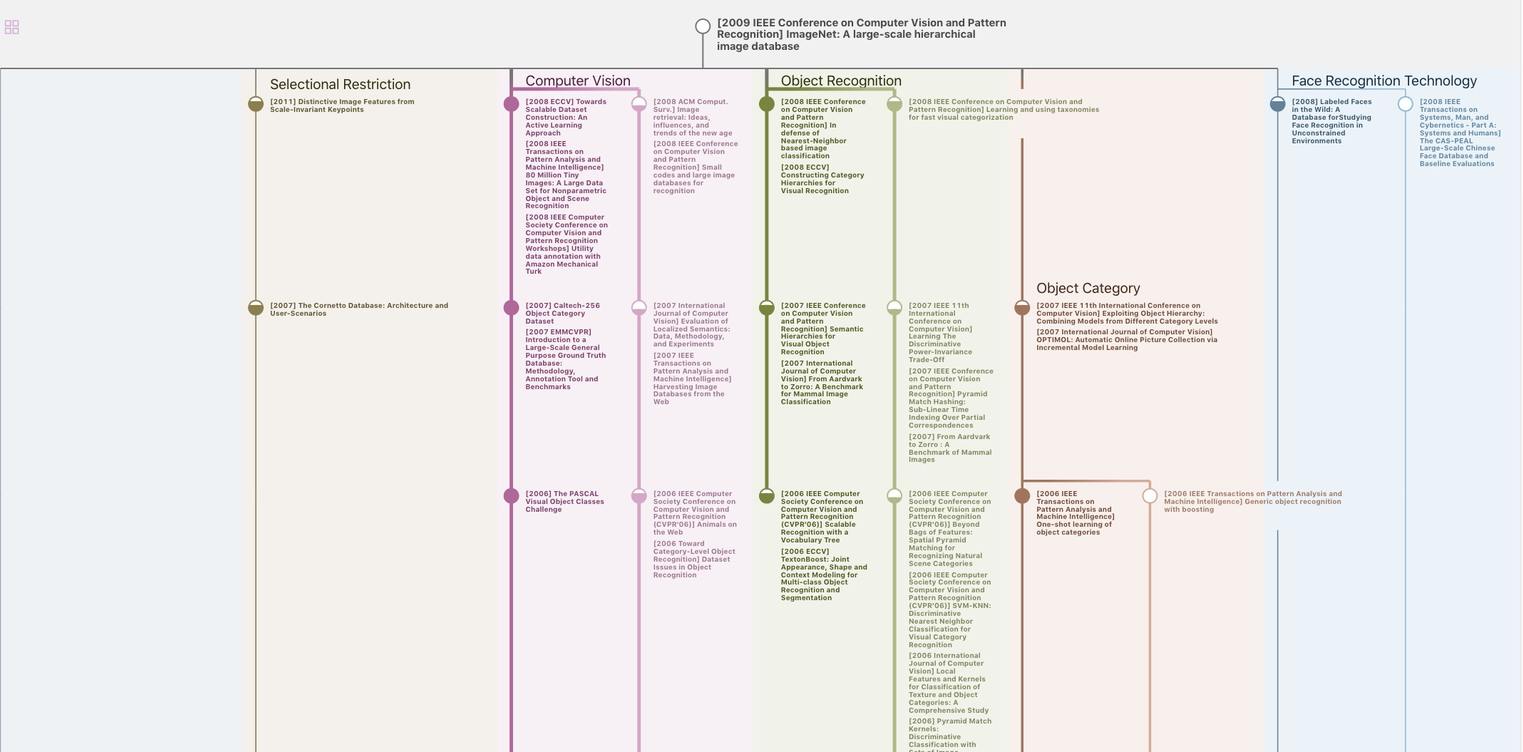
生成溯源树,研究论文发展脉络
Chat Paper
正在生成论文摘要