Estimation of the Connectivity of Random Graphs Through Q-Learning Techniques
IEEE Journal of Radio Frequency Identification(2022)
摘要
Motivated by its applications to real-world sensor networks, the problem of connectivity estimation of random graphs is investigated. Random graphs are utilized here for representing networks with probabilistic node-to-node communication links. In this context, the unknown probability matrix of the network characterizes the existence of graph edges. This publication presents two novel adaptive algorithms based on the Q-learning technique for estimating said random graphs probability matrix. Those algorithms exploit different methods for computing moving averages. Afterwards, an estimation of the generalized algebraic connectivity is obtained from the estimated probability matrix. The effectiveness of the proposed algorithms is verified by simulation for graphs mimicking underwater sensor networks. Compared to previous work, the authors introduce an estimation scheme tailored to time-varying conditions, a simplification of the upgrade function, and new performance metrics prior to discussing their usefulness. In most scenarios, the proposed procedures outperform the previously proposed approach due to their adaptive nature.
更多查看译文
关键词
Network,learning,connectivity
AI 理解论文
溯源树
样例
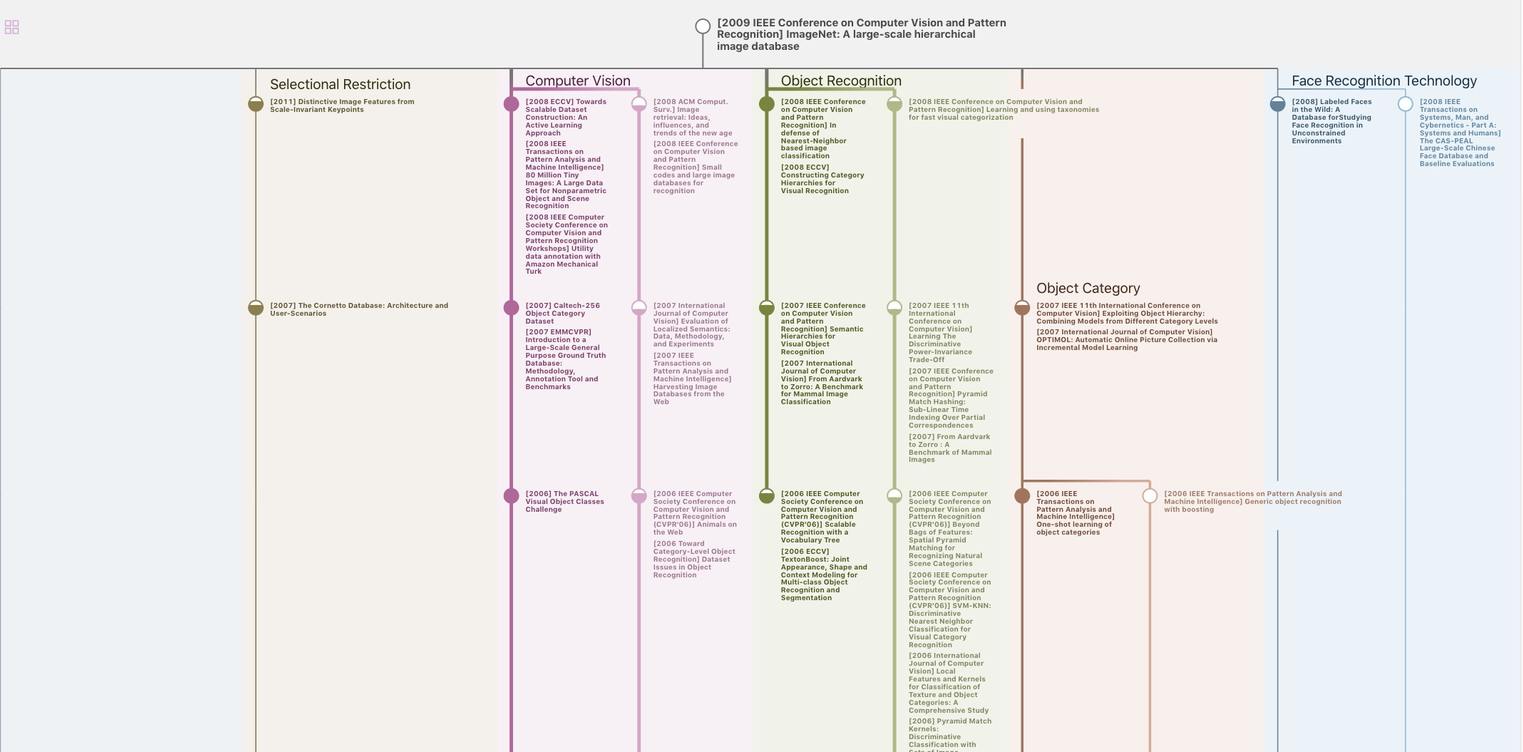
生成溯源树,研究论文发展脉络
Chat Paper
正在生成论文摘要