Stochastic configuration networks for self-blast state recognition of glass insulators with adaptive depth and multi-scale representation
Information Sciences(2022)
摘要
The operating state of insulators is directly related to the stability of power transmission line. The existing methods for insulator state recognition cannot achieve satisfactory performance. In this paper, the self-blast state recognition of glass insulators is investigated by using an adaptive learning representation. To increase the adaptability of the network to different scales, we propose a solution based on multi-scale information throughout the entire process, beginning from a low-scale to high-scale subnetworks. The multi-scale information is aggregated in parallel way to take advantage of rich information representation. Then, an imitation of the human thinking pattern is employed. Utilizing entropy-based cost function, we update the parameters of the learner model in real-time. Based on the constraint of the evaluation index, adaptive depth representation for training glass insulators that are unsatisfied with the reliability evaluation is constructed to realize the self-optimizing regulation of feature space. Correspondingly, a stochastic configuration networks (SCNs) classifier is re-constructed to fit for the update multi-hierarchies knowledge space to carry out the re-recognition process. Finally, fuzzy integration is employed to ensemble multi-hierarchies network to improve the model’s generalization. The recognition results on aerial dataset of insulators images demonstrate the effectiveness of our proposed approach.
更多查看译文
关键词
Self-blast state,Adaptive depth and multi-scale representation,Ensemble learning,Stochastic configuration networks,Feedback mechanism
AI 理解论文
溯源树
样例
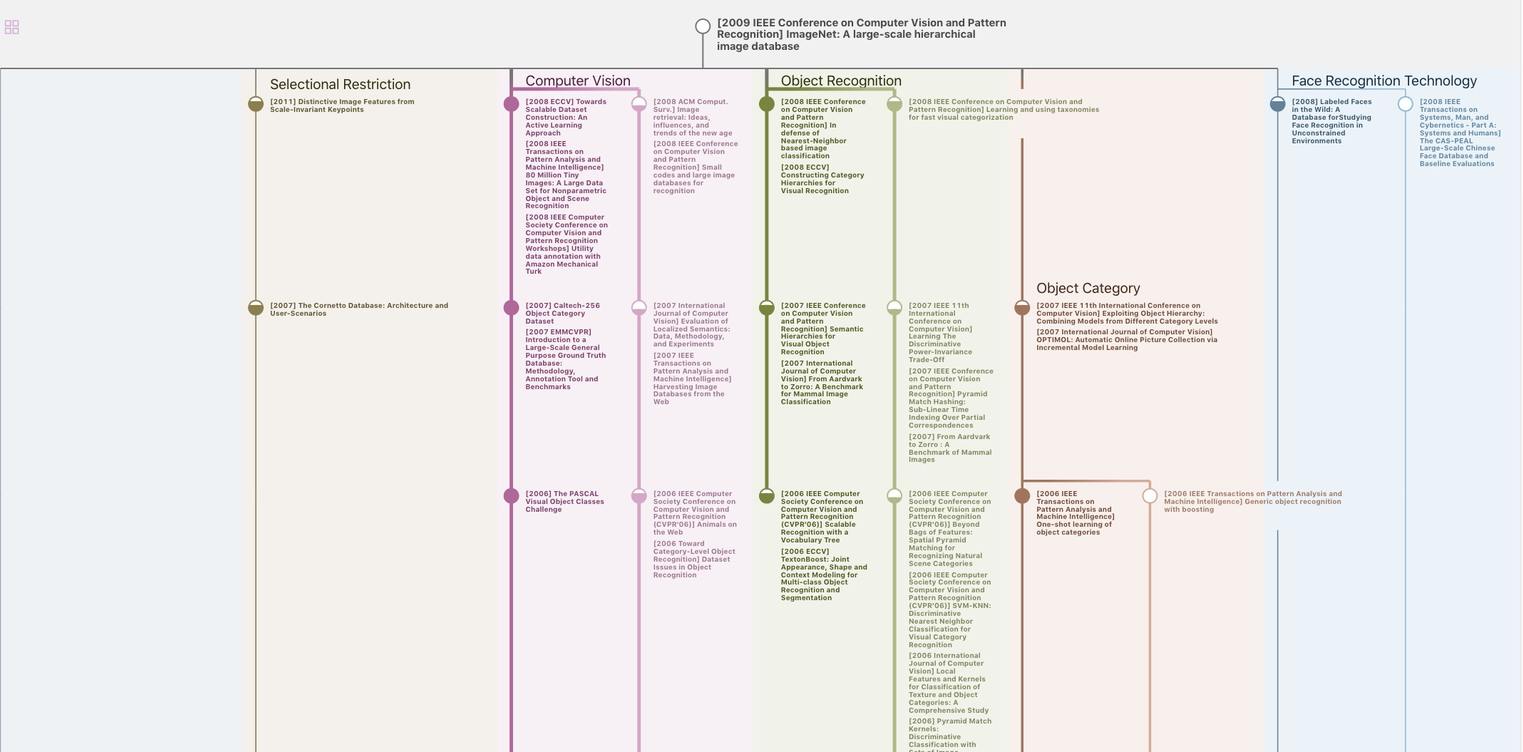
生成溯源树,研究论文发展脉络
Chat Paper
正在生成论文摘要