A Robotic Grasping State Perception Framework With Multi-Phase Tactile Information and Ensemble Learning
IEEE ROBOTICS AND AUTOMATION LETTERS(2022)
摘要
Recently, tactile sensing has attracted increasing attention for robotic manipulation. Predicting the grasping stability before lifting objects and detecting the ongoing/onset of slip after lifting objects are two critical and widely studied tasks in robotic tactile manipulation. Previous methods focus on proposing novel neural networks (NN) architectures towards one of the above two tasks and did not consider that the two tasks are employed in two interconnected action-phases, i.e. grasping and lifting. Therefore, we firstly explore the possibility of constructing a multi-phase, multi-output framework to combine the stability prediction before lifting and the slip detection after lifting. Moreover, to improve the prediction/detection accuracy, we also proposed to explicitly ensemble different NN architectures using various methods, including attention mechanisms. Our experiments are done with 6 state-of-art NN architectures on two datasets including more than 3000 robotic grasps over 80 objects in total. Furthermore our proposals are tested in a real-time robot experiment with unseen objects. Our experimental results show that the proposed multi-phase, multi-output model exhibits more reliable and flexible performance than a single phase model. We also show that using the ensemble of different NN architectures is a viable and practical choice to boost the overall performance.
更多查看译文
关键词
Deep learning in grasping and manipulation, grasping, perception for grasping and manipulation
AI 理解论文
溯源树
样例
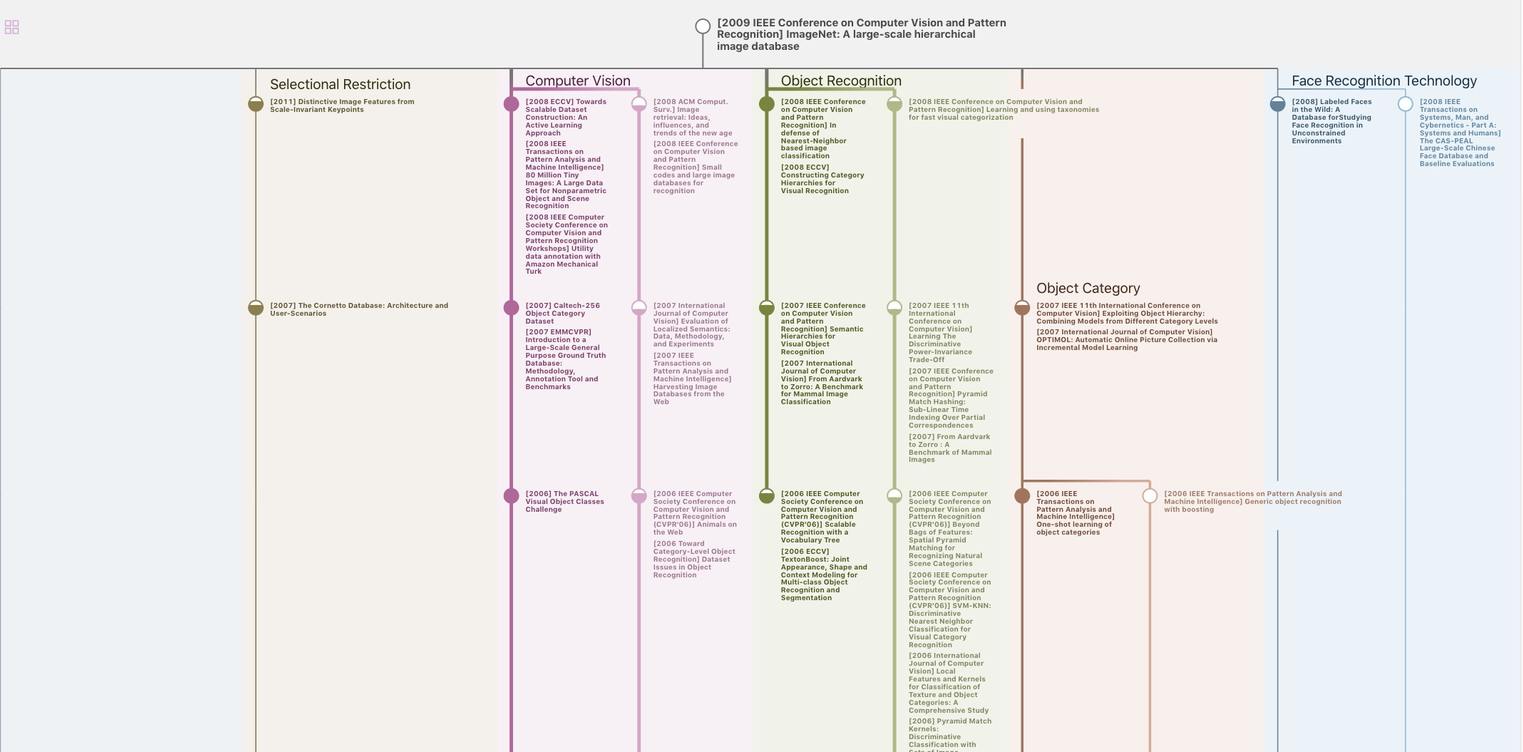
生成溯源树,研究论文发展脉络
Chat Paper
正在生成论文摘要