AUPOD: End-to-End Automatic Poster Design by Self-Supervision
IEEE ACCESS(2022)
摘要
The automatic design has become a popular topic in the application field of computer vision technologies. Previous methods for automatic design are mostly saliency-based, relying on an off-the-shelf model for saliency map detection and hand-crafted aesthetic rules for ranking on multiple proposals. We argue that the multi-stage generation and the excessive reliance on saliency map hindered the progress of pursuing better automatic design solutions. In this work, we explore the possibility of a saliency-free solution in a representative scenario, automatic poster design. We propose a novel end-to-end framework to solve the automatic poster design problem, which is divided into the layout prediction and attributes identification sub-tasks. We design a neural network based on multi-modality feature extraction to learn the two sub-tasks jointly. We train the deep neural network in our framework with automatically extracted supervision from semi-structured posters, bypassing a large amount of required manual labor. Both qualitative and quantitative results show the impressive performance of our end-to-end approach after discarding the explicit saliency detection module. Our system learned on self-supervision performs well on the automatic design by learning aesthetic constraints implicitly in the neural networks.
更多查看译文
关键词
Layout, Feature extraction, Training, Pipelines, Task analysis, Neural networks, Visualization, Design automation, design aesthetic, artificial intelligence, neural networks, machine learning
AI 理解论文
溯源树
样例
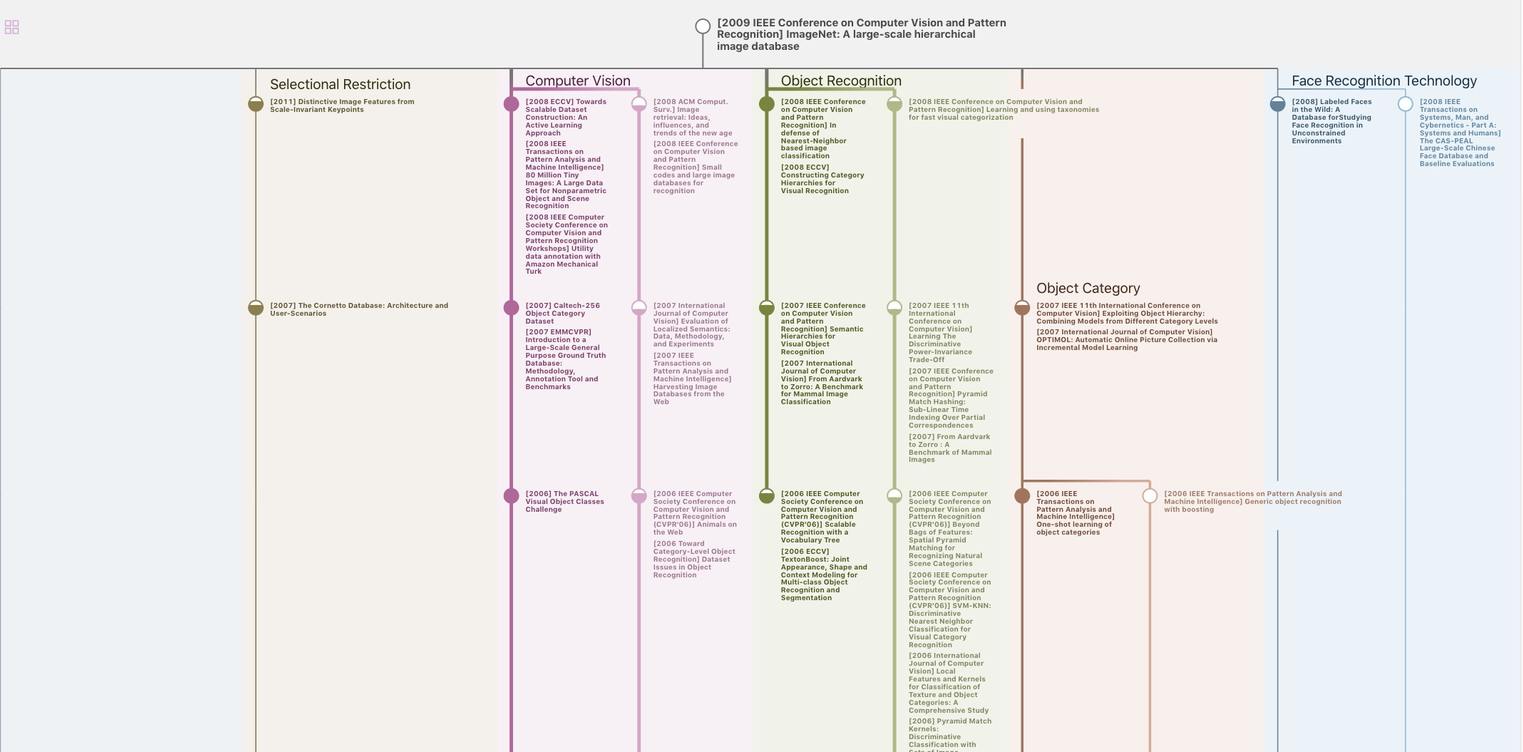
生成溯源树,研究论文发展脉络
Chat Paper
正在生成论文摘要