Power quality disturbance classification under noisy conditions using adaptive wavelet threshold and DBN-ELM hybrid model
ELECTRIC POWER SYSTEMS RESEARCH(2022)
摘要
To solve the problems of noise interference and artificial feature extraction in power quality disturbance (PQD) classification, a new method combining adaptive wavelet threshold denoising and deep belief network fusion extreme learning machine (DBN-ELM) is proposed. Firstly, the noise content of the layer is determined by calculating the energy ratio of the wavelet coefficients of each layer, and an adaptive wavelet threshold is constructed based on the energy ratio to denoise the PQD signals. Secondly, the feature extraction capability of DBN is used to extract the feature from the PQD signals after denoising. Finally, a novel PQD classifier called DBN-ELM is constructed by integrating an ELM into a DBN, which avoids global fine-tuning of DBN and improves PQD classification efficiency. The simulation result and experimental verification show that the proposed method can effectively suppress PQD noise and performs well on DBN-ELM classification.
更多查看译文
关键词
Power quality disturbance(PQD), Deep belief network(DBN), Extreme learning machine(ELM), Adaptive wavelet threshold, Restricted boltzmann machine(RBM)
AI 理解论文
溯源树
样例
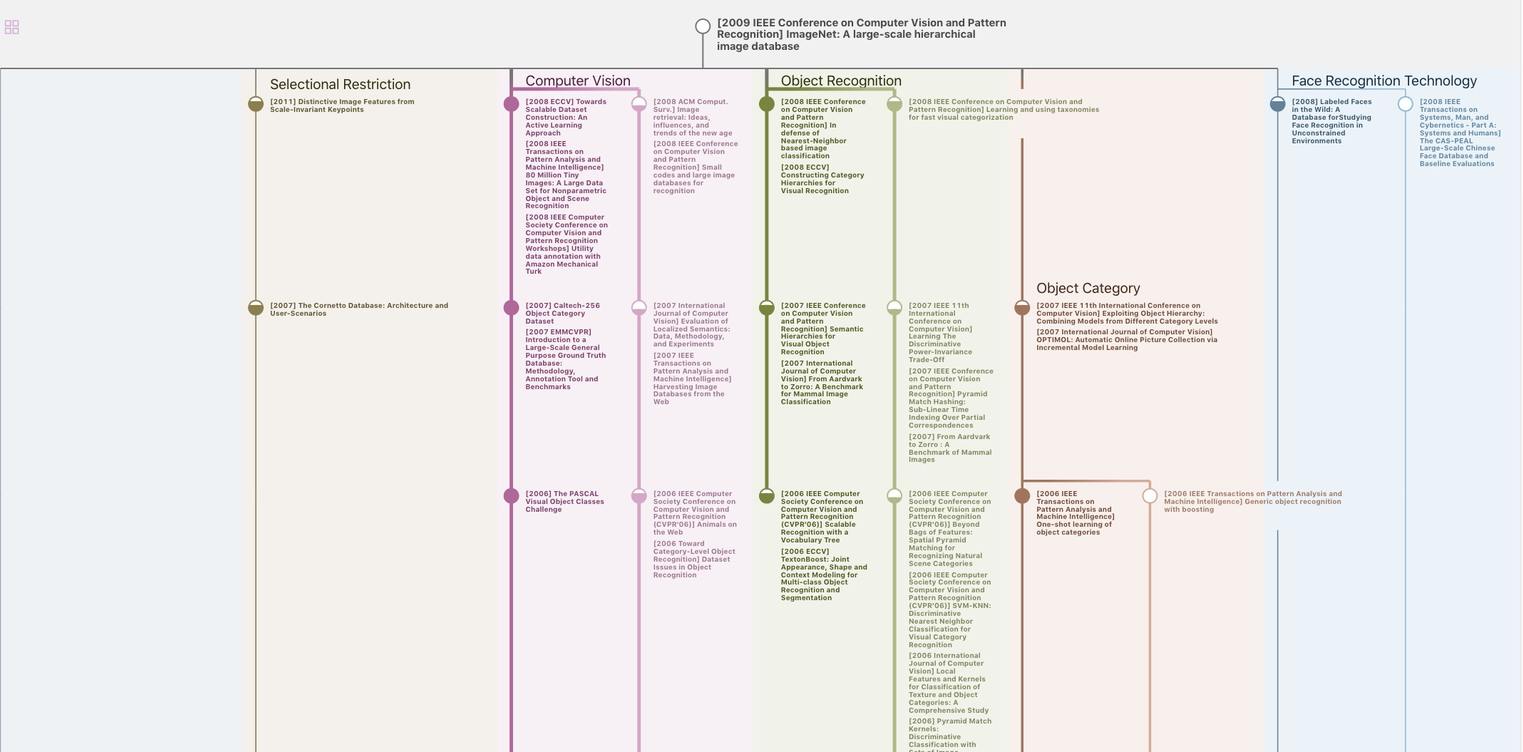
生成溯源树,研究论文发展脉络
Chat Paper
正在生成论文摘要