Image-Goal Navigation in Complex Environments via Modular Learning
IEEE ROBOTICS AND AUTOMATION LETTERS(2022)
摘要
We present a novel approach for image-goal navigation, where an agent navigates with a goal image rather than accurate target information, which is more challenging. Our goal is to decouple the learning of navigation goal planning, collision avoidance, and navigation ending prediction, which enables more concentrated learning of each part. This is realized by four different modules. The first module maintains an obstacle map during robot navigation. The second predicts a long-term goal on the real-time map periodically, which can thus convert an image-goal navigation task to several point-goal navigation tasks. To achieve these point-goal navigation tasks, the third module plans collision-free command sets for navigating to these long-term goals. The final module stops the robot properly near the goal image. The four modules are designed or maintained separately, which helps cut down the search time during navigation and improve the generalization to previously unseen real scenes. We evaluate the method in both a simulator and in the real world with a mobile robot. The results in real complex environments show that our method attains at least a 17% increase in navigation success rate and a 23% decrease in navigation collision rate over some state-of-the-art models.
更多查看译文
关键词
Modular learning, motion and path planning, reactive and sensor-based planning, vision-based navigation
AI 理解论文
溯源树
样例
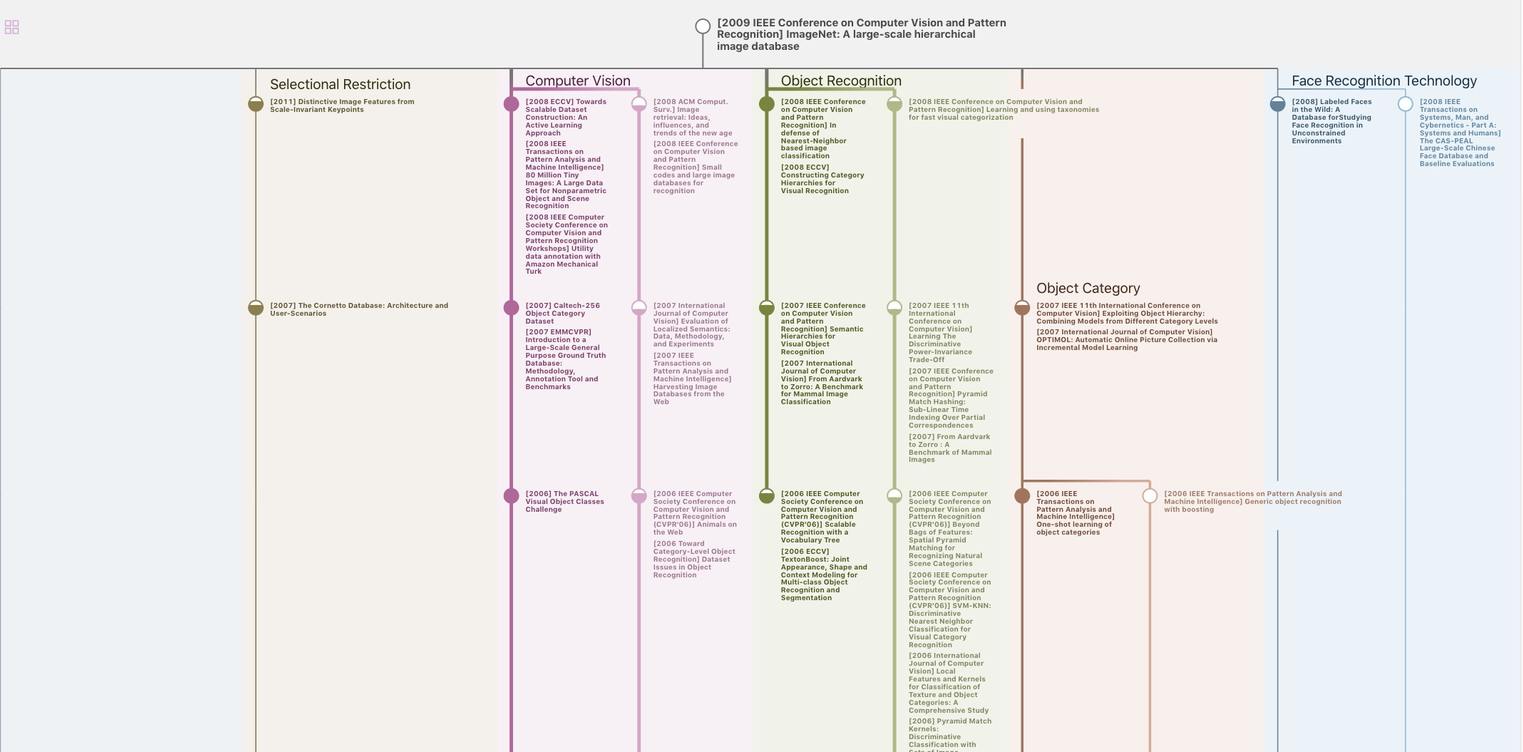
生成溯源树,研究论文发展脉络
Chat Paper
正在生成论文摘要