A machine learning-based procedure for leveraging clickstream data to investigate early predictability of failure on interactive tasks
BEHAVIOR RESEARCH METHODS(2022)
摘要
Early detection of risk of failure on interactive tasks comes with great potential for better understanding how examinees differ in their initial behavior as well as for adaptively tailoring interactive tasks to examinees’ competence levels. Drawing on procedures originating in shopper intent prediction on e-commerce platforms, we introduce and showcase a machine learning-based procedure that leverages early-window clickstream data for systematically investigating early predictability of behavioral outcomes on interactive tasks. We derive features related to the occurrence, frequency, sequentiality, and timing of performed actions from early-window clickstreams and use extreme gradient boosting for classification. Multiple measures are suggested to evaluate the quality and utility of early predictions. The procedure is outlined by investigating early predictability of failure on two PIAAC 2012 Problem Solving in Technology Rich Environments (PSTRE) tasks. We investigated early windows of varying size in terms of time and in terms of actions. We achieved good prediction performance at stages where examinees had, on average, at least two thirds of their solution process ahead of them, and the vast majority of examinees who failed could potentially be detected to be at risk before completing the task. In-depth analyses revealed different features to be indicative of success and failure at different stages of the solution process, thereby highlighting the potential of the applied procedure for gaining a finer-grained understanding of the trajectories of behavioral patterns on interactive tasks.
更多查看译文
关键词
Interactive tasks, Early prediction, Extreme gradient boosting, Time-stamped action sequences, Clickstreams, PIAAC
AI 理解论文
溯源树
样例
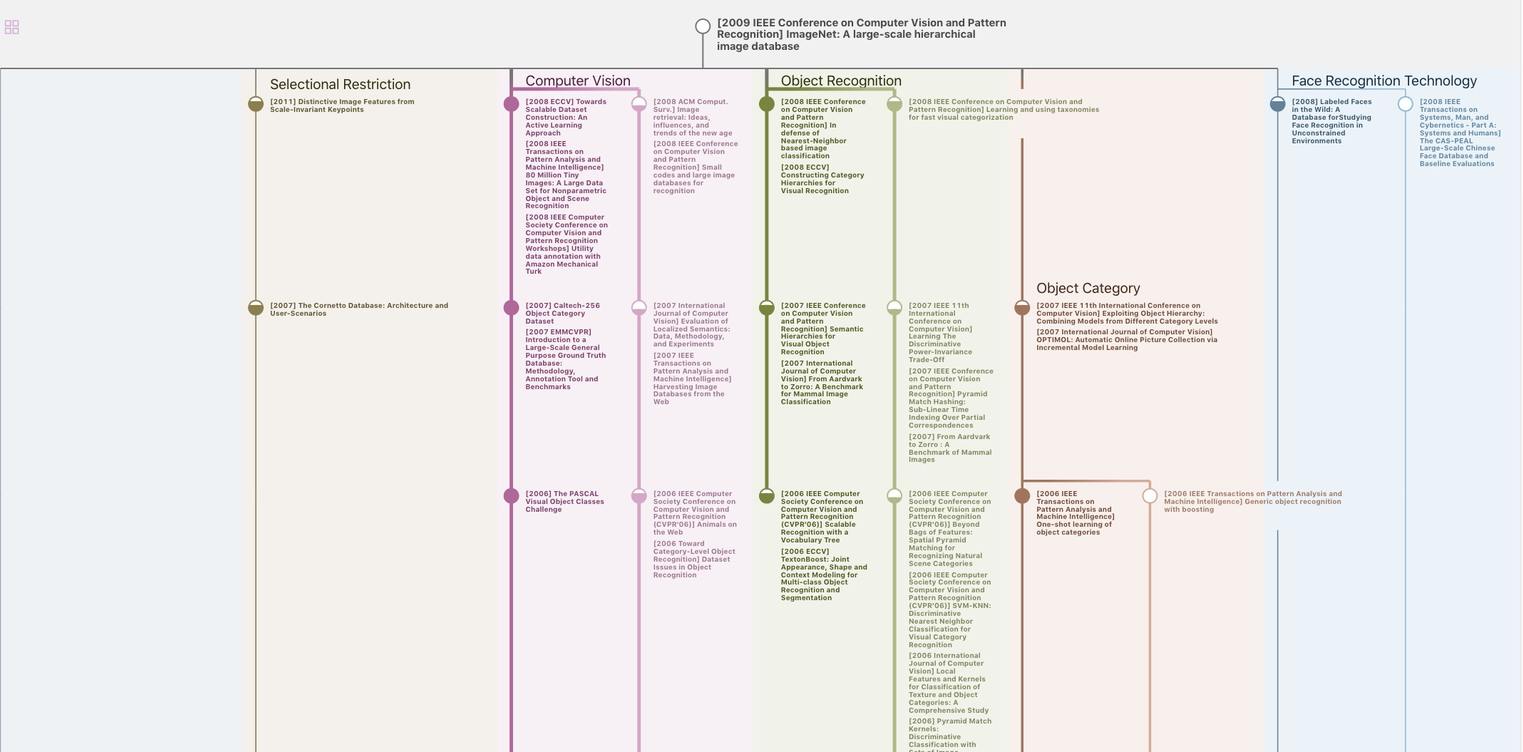
生成溯源树,研究论文发展脉络
Chat Paper
正在生成论文摘要