Deep learning approach for early detection of sub-surface bruises in fruits using single snapshot spatial frequency domain imaging
JOURNAL OF FOOD MEASUREMENT AND CHARACTERIZATION(2022)
摘要
Spatial frequency domain imaging (SFDI) technique shows enhanced ability to detect sub-surface bruises compared to planar illumination. However, conventional three-phase-shifting SFDI is limited by the speed of image acquisition, and the single snapshot methods using filtering windows suffer from low signal-to-noise ratio and image degradation, which has a negative impact on the detection accuracy. In this study, conditional Generative Adversarial Networks (cGANs) were adopted to do the image-to-image translation for single snapshot images. For training and testing of the cGANs, 750 single snapshot images of ‘Red Fuji’ apples were used as input, paired with the alternating component images demodulated by conventional SFDI as ground truth. Results show that at spatial frequencies of 100 m −1 and 200 m −1 , the cGAN-predicted images present higher values of peak signal-to-noise-ratio (PSNR) and structural similarity index (SSIM) than images processed by filtering. Moreover, the trained cGANs can be used for ‘Crystal Fuji’ apples and ‘Crown’ pears with performance robustness. This cGAN-based method would have potential applications for real-time, wild-field, sub-surface defects detection and quality assessment of horticultural and agricultural products.
更多查看译文
关键词
Bruise, Single snapshot, Spatial frequency domain imaging, Deep learning
AI 理解论文
溯源树
样例
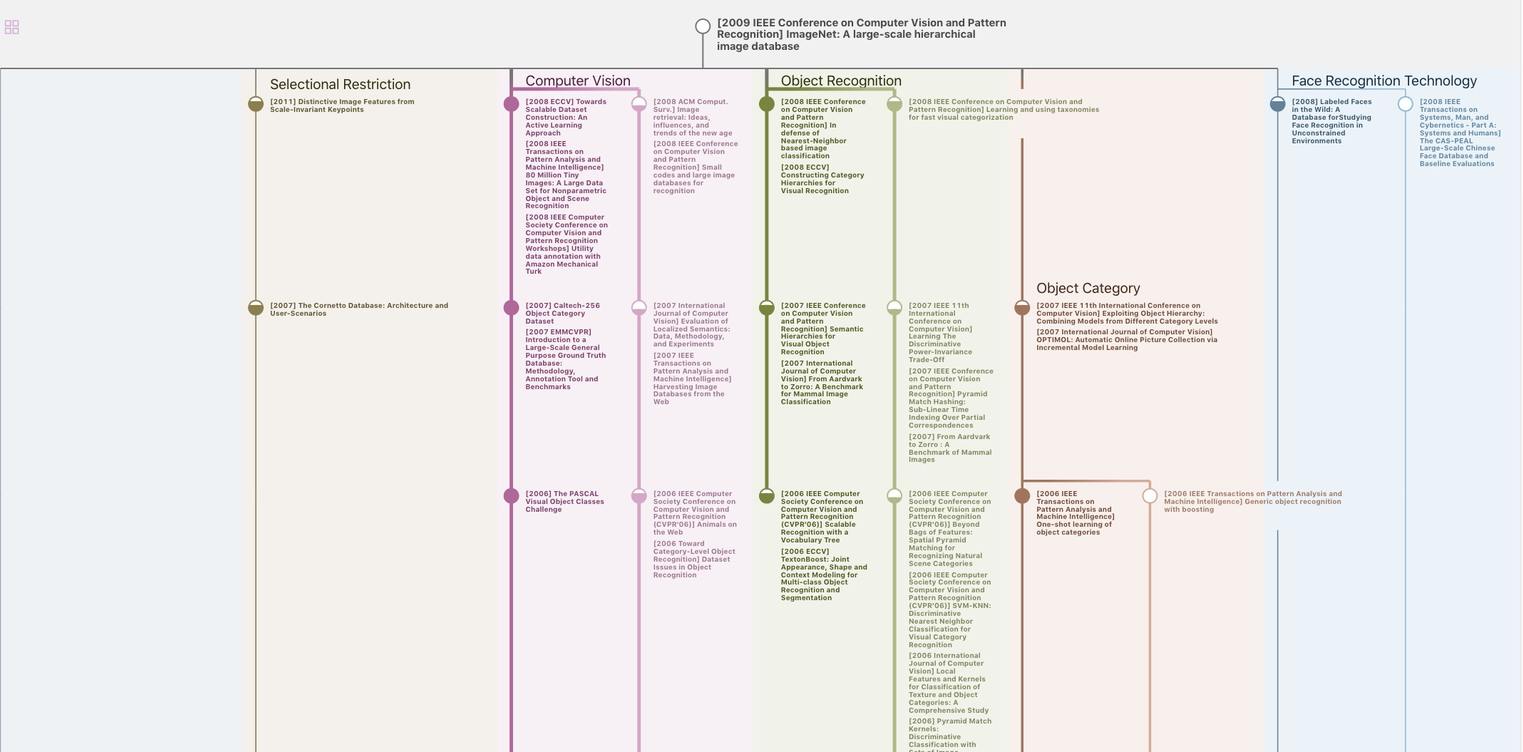
生成溯源树,研究论文发展脉络
Chat Paper
正在生成论文摘要