Utilizing Skipped Frames in Action Repeats for Improving Sample Efficiency in Reinforcement Learning
IEEE ACCESS(2022)
摘要
Action repeat has become the de-facto mechanism in deep reinforcement learning (RL) for stabilizing training and enhancing exploration. Here, the action is taken at the action-decision point and is executed repeatedly for a designated number of times until the next decision point. Although showing several advantages, in this mechanism, the intermediate states which stem from repeated actions are discarded in training agents, causing sample inefficiency. To utilize the discarded states as training data is nontrivial as the action, which causes the transition between these states, is unavailable. This paper proposes to infer the action at the intermediate states via an inverse dynamic model. The proposed method is simple and easily incorporated into the existing off-policy RL algorithms - integrating the proposed method with SAC shows consistent improvement across various tasks.
更多查看译文
关键词
Task analysis, Training, Heuristic algorithms, Benchmark testing, Data models, Training data, Robots, Action repeat mechanism, off-policy reinforcement learning, reinforcement learning, sample efficiency
AI 理解论文
溯源树
样例
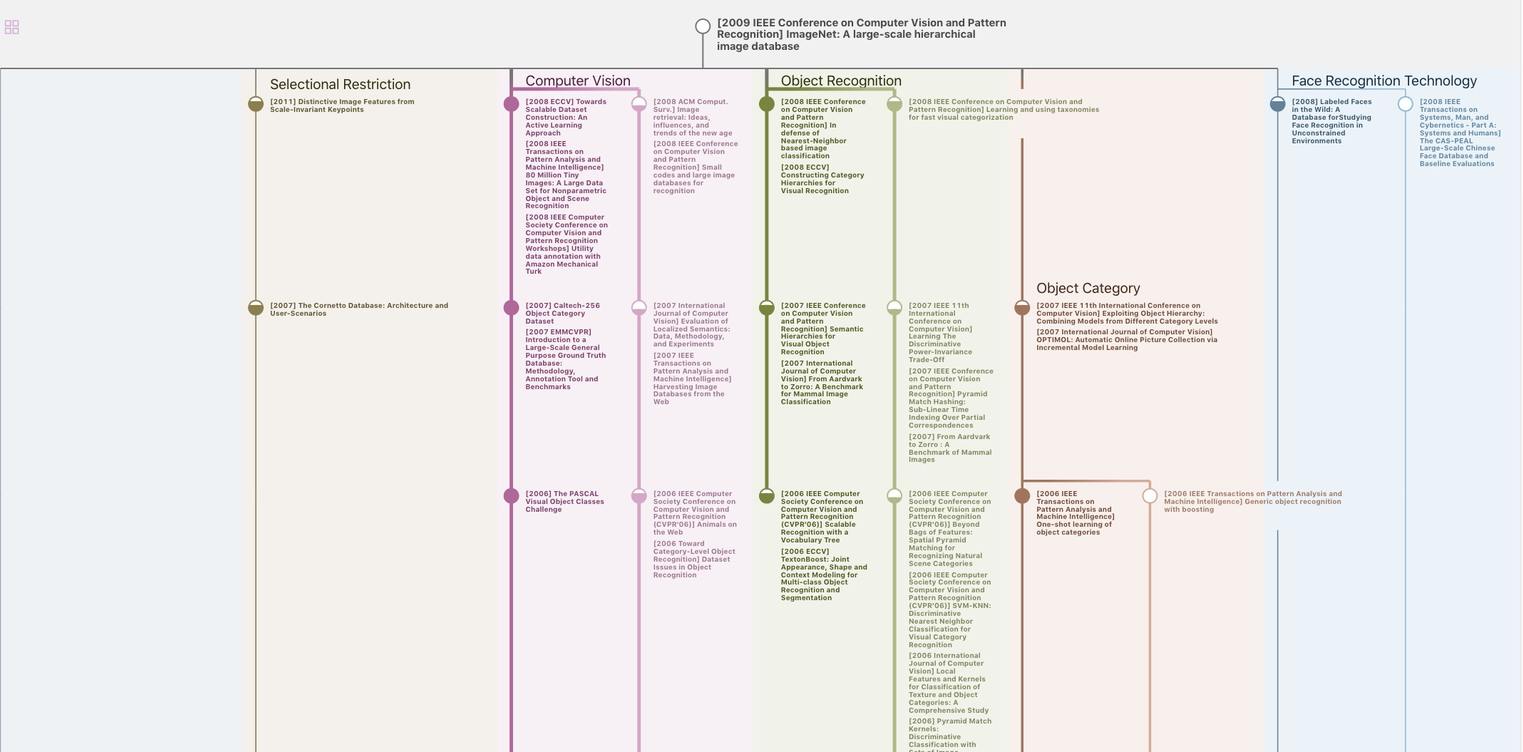
生成溯源树,研究论文发展脉络
Chat Paper
正在生成论文摘要