Fixed-time neuroadaptive practical tracking control based on extended state/disturbance observer for a QUAV with external disturbances and time-varying parameters
Journal of the Franklin Institute(2022)
摘要
This paper investigates the fixed-time neural network adaptive (FNNA) tracking control of a quadrotor unmanned aerial vehicle (QUAV) to achieve flight safety and high efficiency. By combining radial basis function neural network (RBFNN) with fixed time adaptive sliding mode algorithm, a novel radial basis function neural network adaptive law is proposed. In addition, an extended state/disturbance observer (ESDO) is proposed to solve the problem of unmeasurable state and external interference, which can obtain reliable state feedback and interference input. Unlike most other ESO applications, this paper does not set the uncertainty model and external disturbances as total disturbances. Instead, the external disturbances are observed by extending the states and the observed states are fed back to the controller to cancel the disturbances. In view of the time-varying resistance coefficient and inertia torque in the QUAV model, the neural network is introduced so that the controller does not need to consider these nonlinear uncertainties. Finally, a numerical example is given to verify the effectiveness of the coupled non-simplified QUAV model.
更多查看译文
AI 理解论文
溯源树
样例
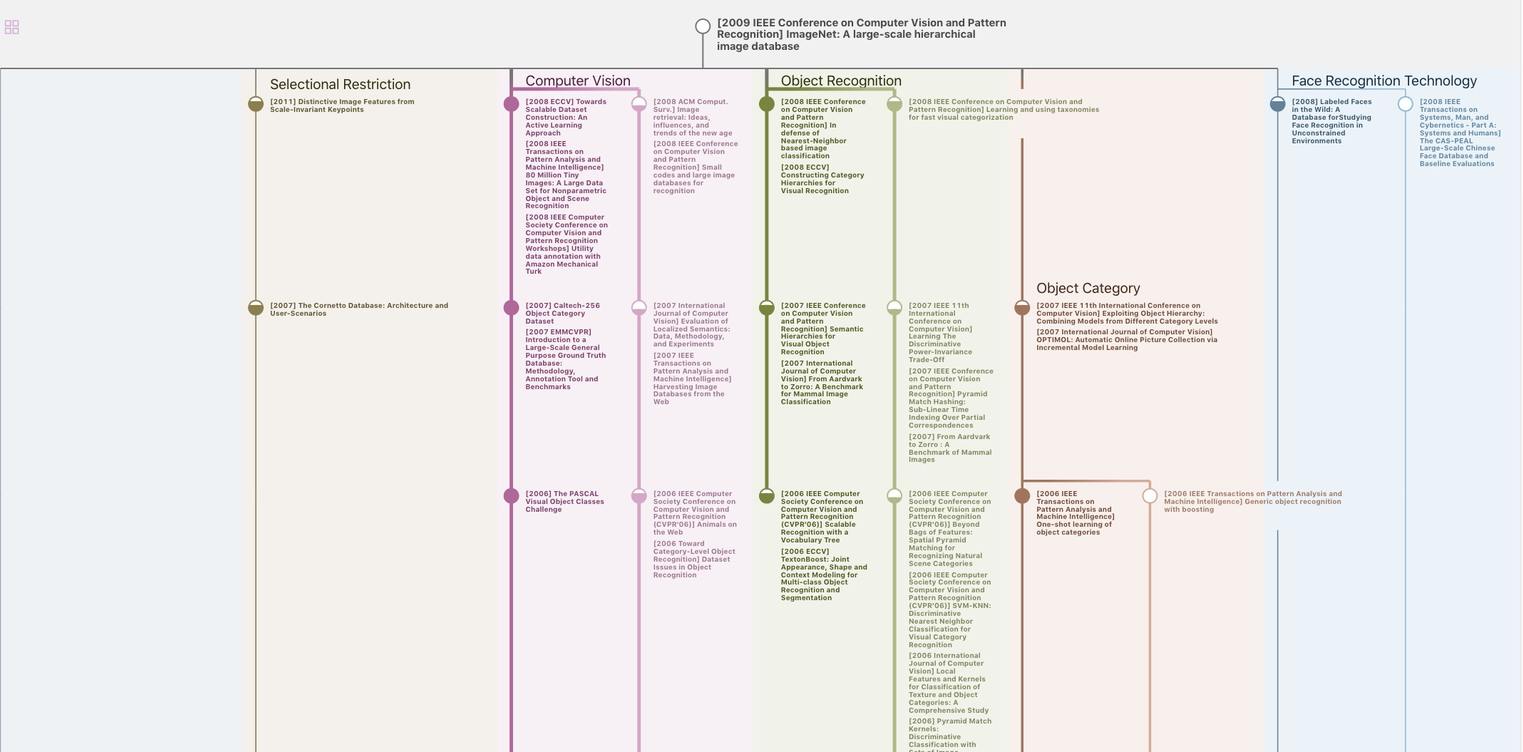
生成溯源树,研究论文发展脉络
Chat Paper
正在生成论文摘要