A Deep Attention Model for Environmental Sound Classification from Multi-Feature Data
APPLIED SCIENCES-BASEL(2022)
摘要
Automated environmental sound recognition has clear engineering benefits; it allows audio to be sorted, curated, and searched. Unlike music and language, environmental sound is loaded with noise and lacks the rhythm and melody of music or the semantic sequence of language, making it difficult to find common features representative enough of various environmental sound signals. To improve the accuracy of environmental sound recognition, this paper proposes a recognition method based on multi-feature parameters and time-frequency attention module. It begins with a pretreatment that relies on multi-feature parameters to extract the sound, which supplements the phase information lost by the Log-Mel spectrogram in the current mainstream methods, and enhances the expressive ability of input features. A time-frequency attention module with multiple convolutions is designed to extract the attention weight of the input feature spectrogram and reduce the interference coming from the background noise and irrelevant frequency bands in the audio. Comparative experiments were conducted on three general datasets: environmental sound classification datasets (ESC-10, ESC-50) and an UrbanSound8K dataset. Experiments demonstrated that the proposed method performs better.
更多查看译文
关键词
environmental sound recognition, multi-feature parameters, attention mechanism
AI 理解论文
溯源树
样例
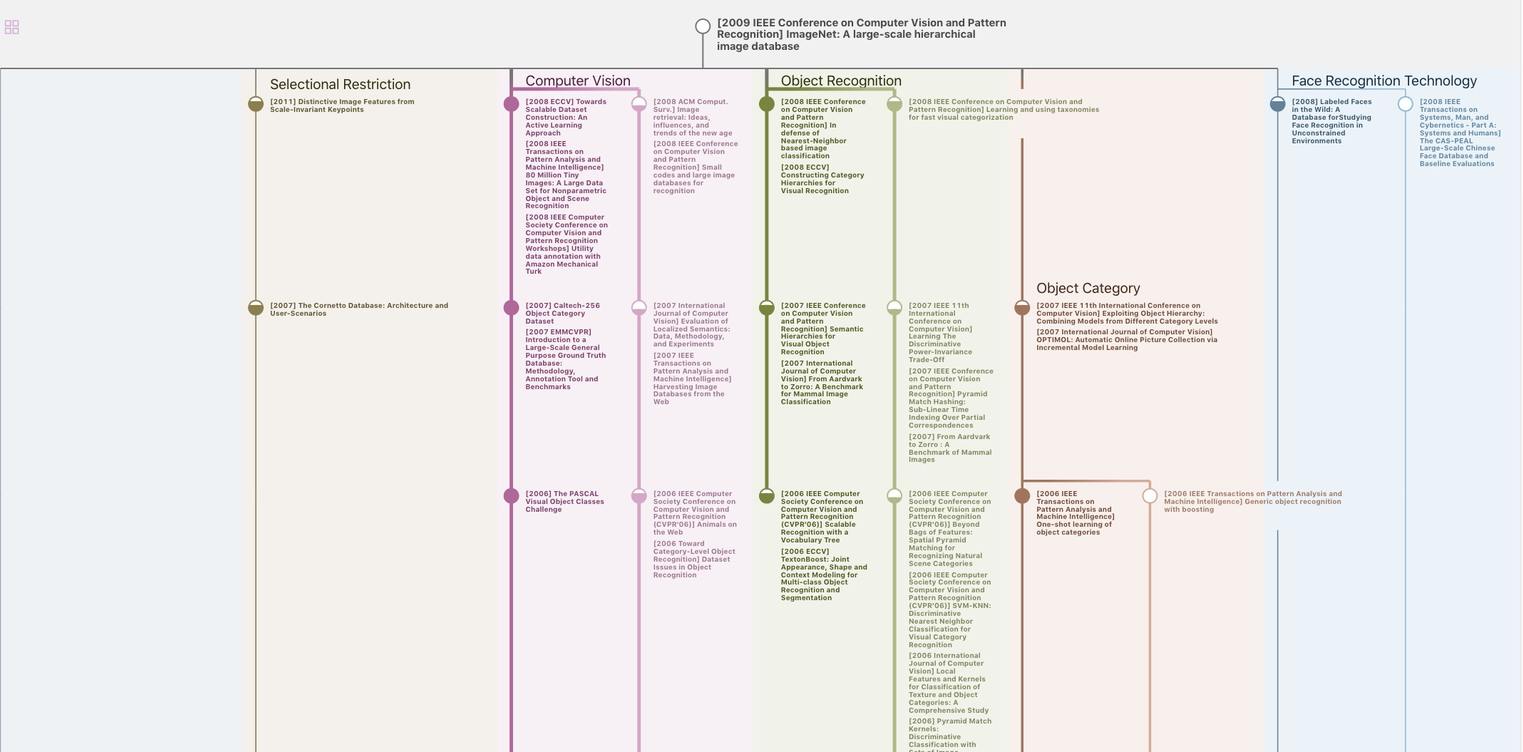
生成溯源树,研究论文发展脉络
Chat Paper
正在生成论文摘要