Statistical Process Monitoring Based on Collaboration Preserving Embedding
IEEE TRANSACTIONS ON INSTRUMENTATION AND MEASUREMENT(2022)
摘要
The applications of dimensionality reduction method in statistical process monitoring have been widely researched in recent years. Considering the incompleteness and one-sidedness of the most existing methods on preserving the timing dynamic characteristics and spatial structure, a new dimensionality reduction method called collaborative preserving embedding (CPE) is proposed and applied for process monitoring in this article. It focuses on preserving intrinsic low-dimensional manifold structure from time series and spatial scale at the same time. In space scale, the local spatial geometric structure information and the global spatial geometric structure information of the data are preserved. In time series, the short-time serial dependence and long-time serial relation of the data are analyzed. Combining the above information constructively, the intrinsic spatial structure and dynamic characteristics are entirely preserved. Simulations on a numerical example, the Tennessee Eastman process (TEP) and a real industrial production process have validated the effectiveness and the advantages of the proposed method.
更多查看译文
关键词
Process monitoring, Dimensionality reduction, Time series analysis, Production, Principal component analysis, Linear programming, Collaboration, Collaborative, dimensionality reduction, process monitoring, spatial and time
AI 理解论文
溯源树
样例
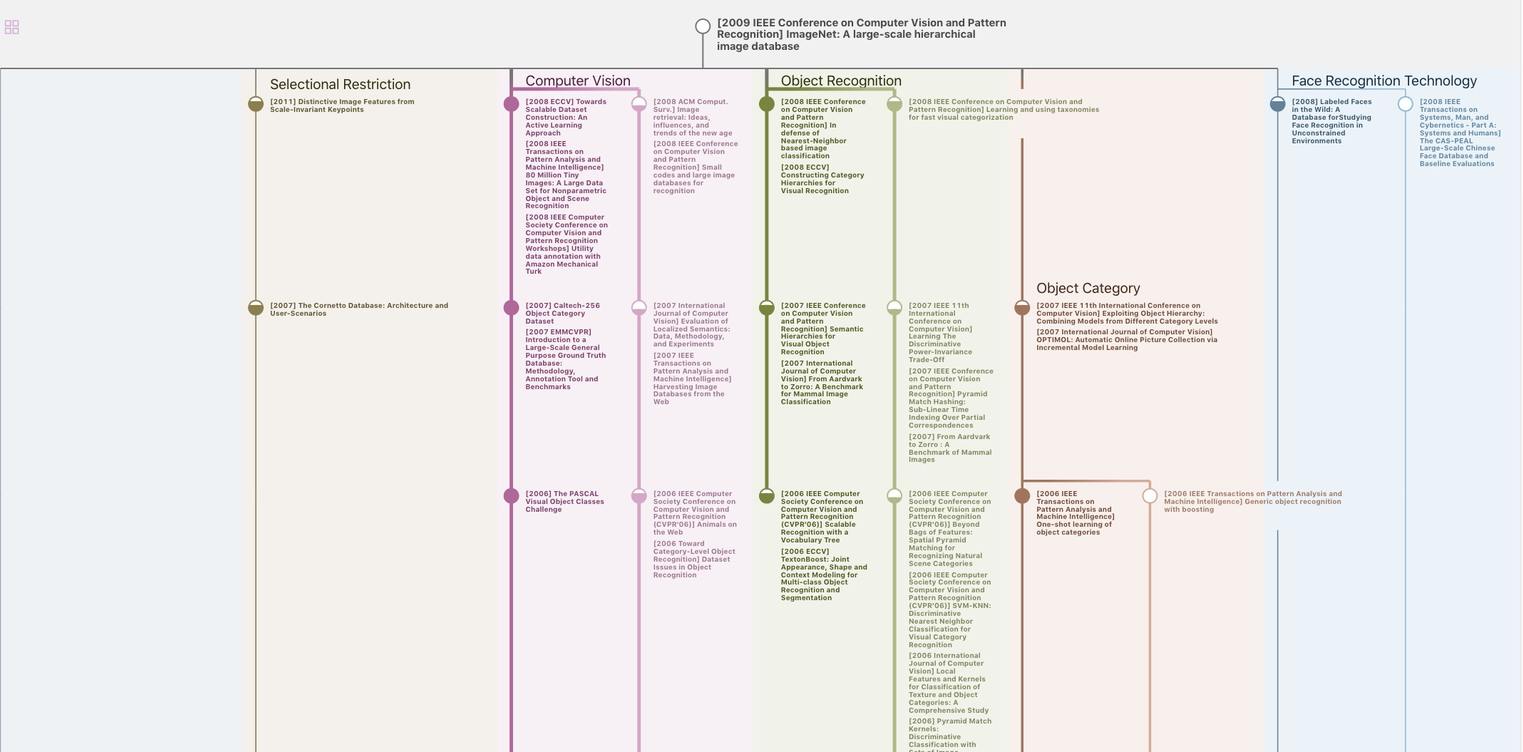
生成溯源树,研究论文发展脉络
Chat Paper
正在生成论文摘要