Automated Detection of Alzheimer's Disease and Mild Cognitive Impairment Using Whole Brain MRI
IEEE ACCESS(2022)
摘要
Early diagnosis is critical for the development and success of interventions, and neuroimaging is one of the most promising areas for early detection of Alzheimer's disease (AD). This study is aimed to develop a deep learning method to extract valuable AD biomarkers from structural magnetic resonance imaging (sMRI) and classify brain images into AD, mild cognitive impairment (MCI), and cognitively normal (CN) groups. In this work, we adapted and trained convolutional neural networks (CNNs) on sMRI images of the brain from ADNI datasets available in online databases. Our proposed mechanism was used to combine features from different layers to hierarchically transform the images from magnetic resonance imaging into more compact high-level features. The proposed method has a reduced number of parameters which reduces the computation complexity. The method is compared with the existing state-of-the-art works for AD classification, which show superior results for the widely used evaluation metrics, including accuracy, an area under the ROC curve, etc., suggesting that our proposed convolution operation is suitable for the AD diagnosis
更多查看译文
关键词
Feature extraction, Magnetic resonance imaging, Convolutional neural networks, Alzheimer's disease, Three-dimensional displays, Deep learning, Support vector machines, Alzheimer's disease (AD), magnetic resonance imaging (MRI), convolutional neural network (CNN), image classification
AI 理解论文
溯源树
样例
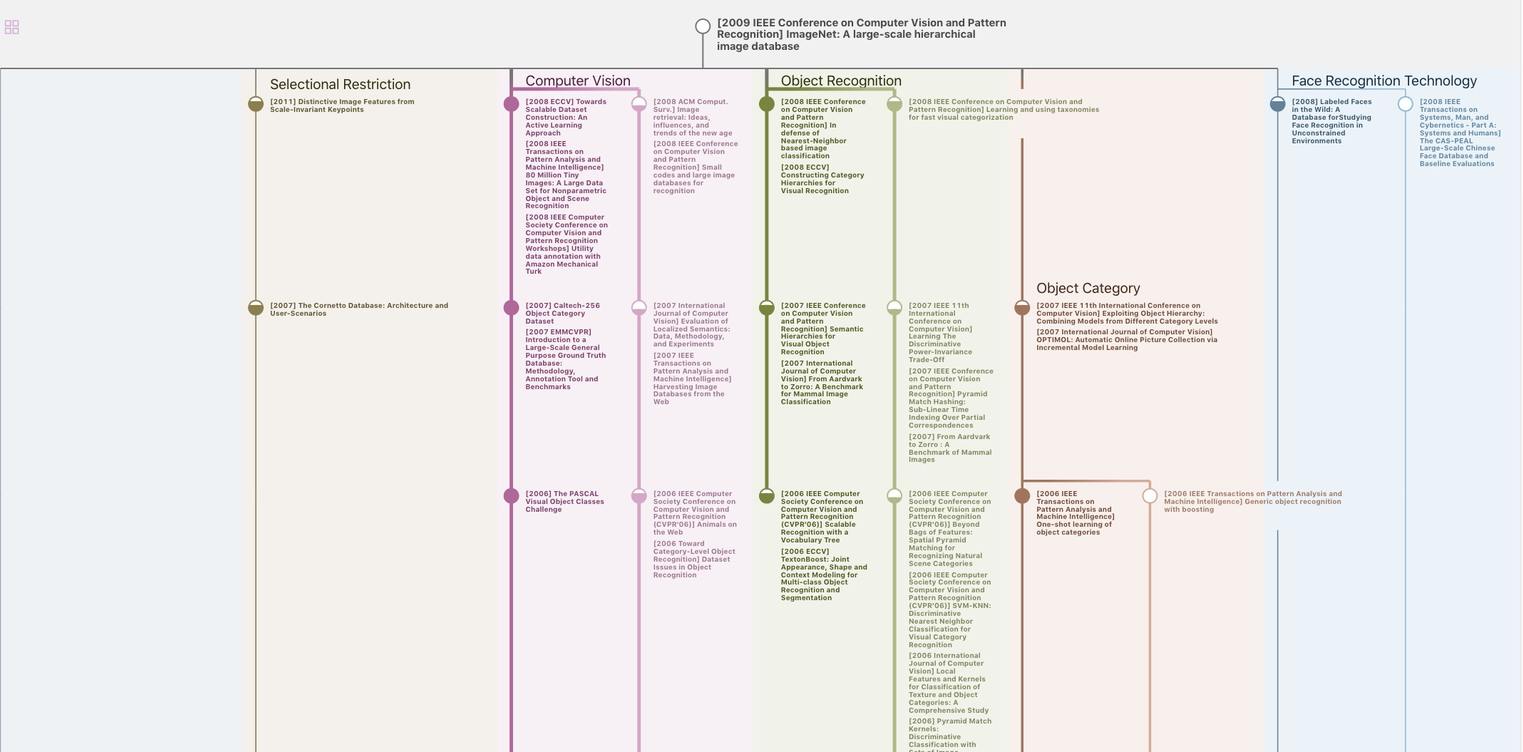
生成溯源树,研究论文发展脉络
Chat Paper
正在生成论文摘要