Video Sparse Transformer With Attention-Guided Memory for Video Object Detection
IEEE ACCESS(2022)
摘要
Detecting objects in a video, known as Video Object Detection (VOD), is challenging since appearance changes of objects over time may bring detection errors. Recent research has focused on aggregating features from adjacent frames to compensate for the deteriorated appearances of a frame. Moreover, using distant frames is also proposed to deal with deteriorated appearances over several frames. Since an object's position may change significantly at a distant frame, they only use features of object candidate regions, which do not depend on their position. However, such methods rely on object candidate regions' detection performance and are not practical for deteriorated appearances. In this paper, we enhance features element-wisely before the object candidate region detection, proposing Video Sparse Transformer with Attention-guided Memory (VSTAM). Furthermore, we propose aggregating element-wise features sparsely to reduce processing time and memory cost. In addition, we introduce an external memory update strategy based on the utilization of the aggregation to hold long-term information effectively. Our method achieved 8.3% and 11.1% accuracy gain from the baseline on ImageNet VID and UA-DETRAC datasets. Our method demonstrates superior performance against state-of-the-art results on widely used VOD datasets.
更多查看译文
关键词
Feature extraction, Transformers, Object detection, Refining, Spatiotemporal phenomena, Memory management, Computational efficiency, Video object detection, video analysis, object detection
AI 理解论文
溯源树
样例
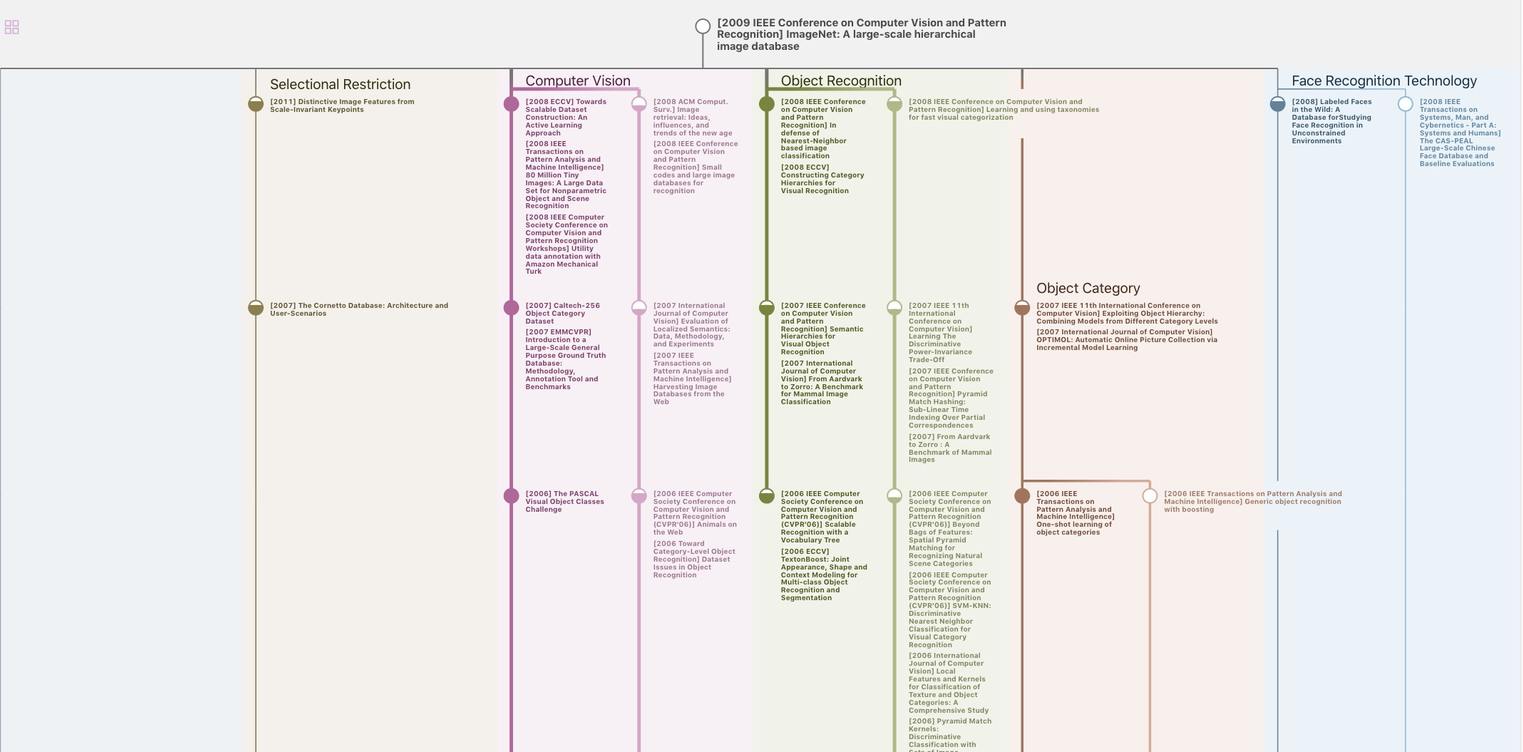
生成溯源树,研究论文发展脉络
Chat Paper
正在生成论文摘要