Area and Feature Guided Regularised Random Forest: a novel method for predictive modelling of binary phenomena. The case of illegal landfill in Canary Island
INTERNATIONAL JOURNAL OF GEOGRAPHICAL INFORMATION SCIENCE(2022)
摘要
This paper presents a novel method, Area and Feature Guided Regularised Random Forest (AFGRRF), applied for modelling binary geographic phenomenon (occurrence versus absence). AFGRRF is a wrapper feature-selection method based on a previous modification of Random Forest (RF), namely the Guided Regularised Random Forest (GRRF). AFGRRF produces maps that minimise the affected area without a significant difference in accuracy. For this, it tunes the GRRF hyper-parameters according to a trade of between True Positive Rate and the affected area (Success Rate). AFGRRF also addresses the 'Rashomon effect' or the multiplicity of good models. The proposed method was tested to model illegal landfills in Gran Canaria Island (Spain). AFGRRF performance was compared to that of other RF-based methods: (i) standard RF; (ii) Area Random Forest (ARF); (iii) Feature Random Forest (FRF); (iv) Area Feature Random Forest (AFRF) and (v) GRRF. AFGRRF predicted the smallest affected area, 19% of the island, at a similar True Positive Rate. This percentage is substantially smaller than the one predicted by RF (27.43%), ARF (26%), FRF (27.78%), AFRF (23%) and GRRF (29.67%).
更多查看译文
关键词
Random Forest, feature selection, predictive modelling, binary phenomena, success rate, illegal landfill
AI 理解论文
溯源树
样例
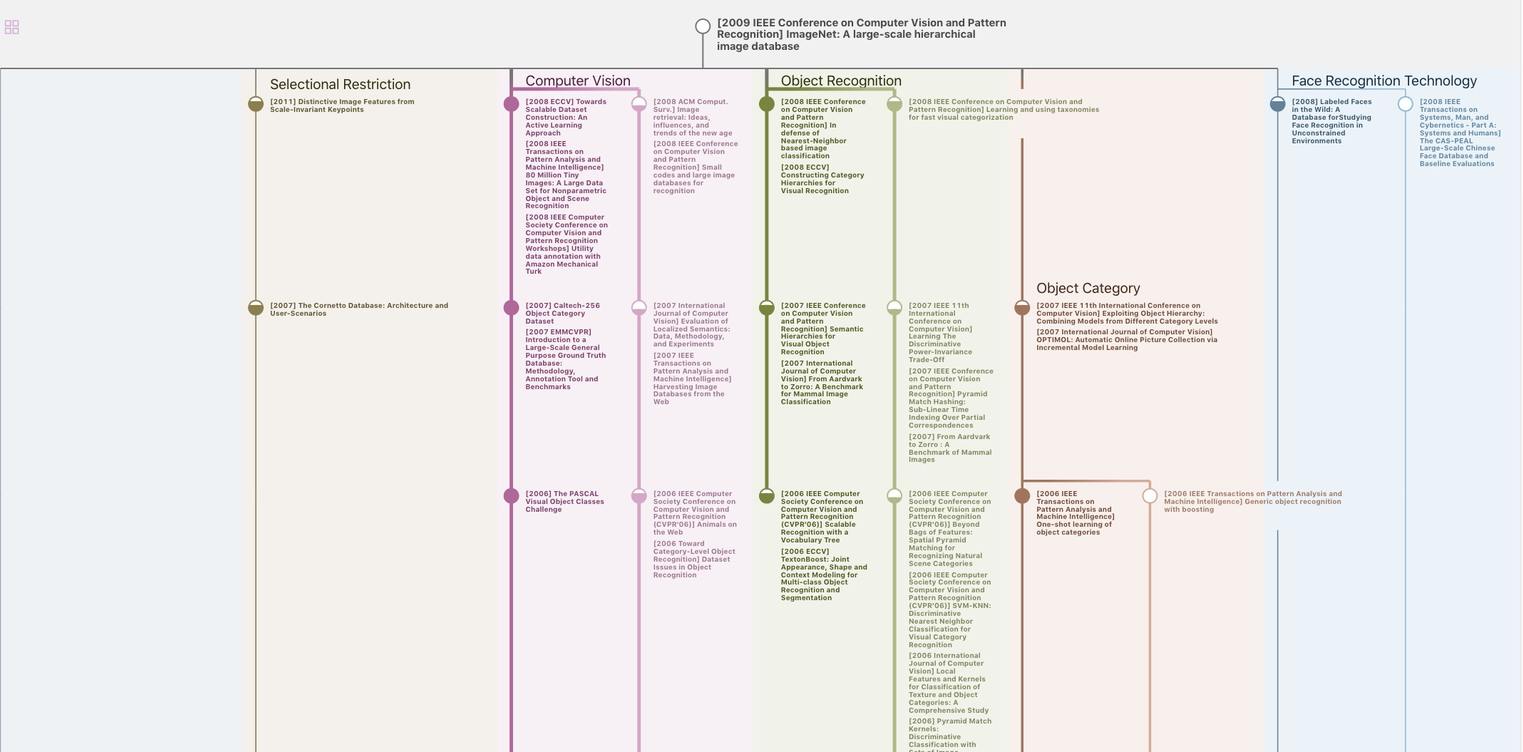
生成溯源树,研究论文发展脉络
Chat Paper
正在生成论文摘要