Bayesian calibration of strength model parameters from Taylor impact data
Computational Materials Science(2022)
摘要
Materials strength plays a key role in determining the mechanical response of engineered structures. As such, accurate strength models are crucial in simulations involving complex loading conditions, particularly when deformation in the plastic regime is deemed important. In this work, a Gaussian process based surrogate for a finite element simulation of a Taylor impact test is developed and used for Bayesian calibration of the Preston–Tonks–Wallace strength model. The surrogate model is shown to closely approximate the salient features of the Taylor cylinder deformation and is validated against simulation output before being used in the strength model calibration routine. The results show that Taylor impact test data can be used in the calibration of constitutive equations through the use of a combination of data science techniques, namely Gaussian processes and Bayesian inference.
更多查看译文
关键词
Materials strength,Surrogate models,Finite elements,Uncertainty quantification
AI 理解论文
溯源树
样例
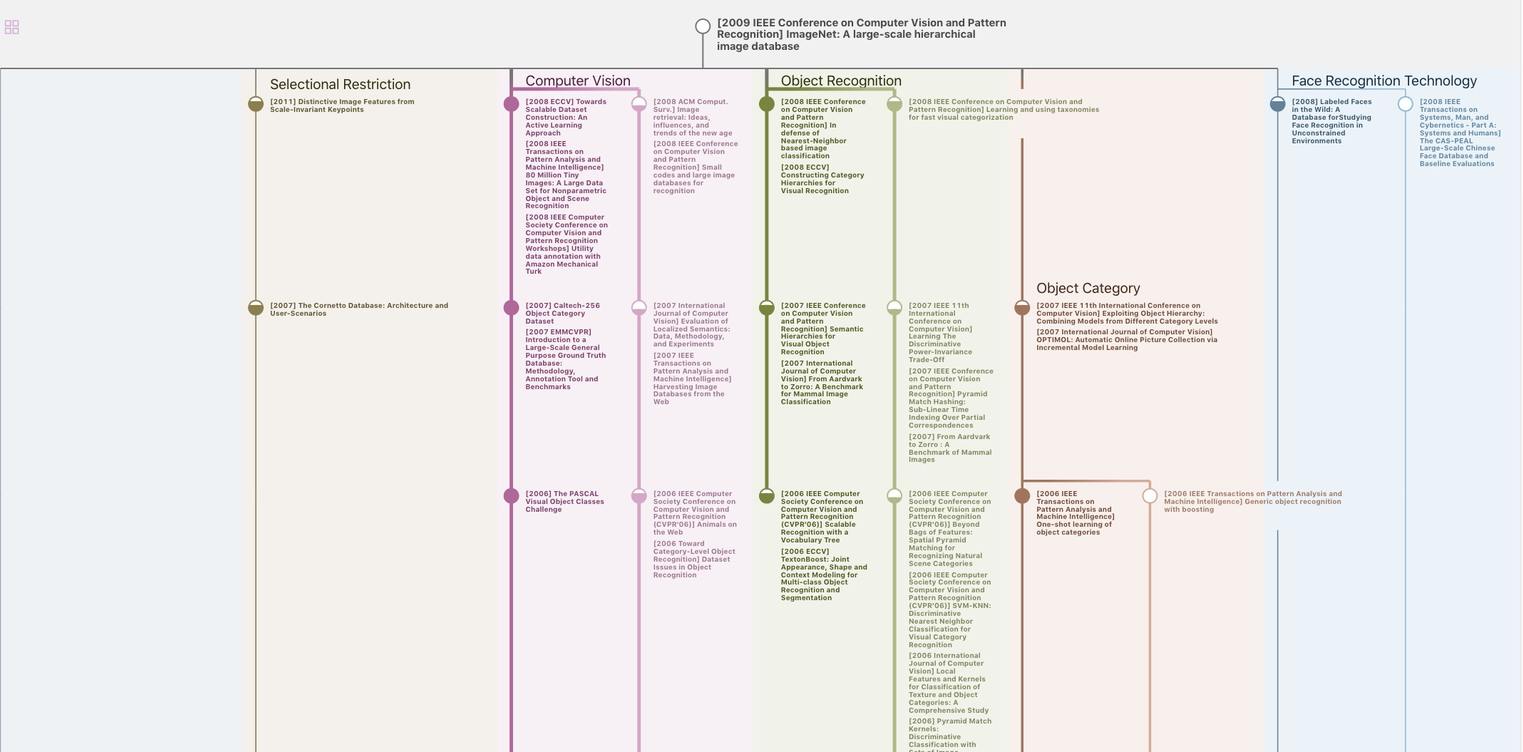
生成溯源树,研究论文发展脉络
Chat Paper
正在生成论文摘要