A New Body Weight Lifelog Outliers Generation Method: Reflecting Characteristics of Body Weight Data
APPLIED SCIENCES-BASEL(2022)
摘要
Lifelogs are generated in our daily lives and contain useful information for health monitoring. Nowadays, one can easily obtain various lifelogs from a wearable device such as a smartwatch. These lifelogs could include noise and outliers. In general, the amount of noise and outliers is significantly smaller than that of normal data, resulting in class imbalance. To achieve good analytic accuracy, the noise and outliers should be filtered. Lifelogs have specific characteristics: low volatility and periodicity. It is very important to continuously analyze and manage them within a specific time. To solve the class imbalance problem of outliers in weight lifelog data, we propose a new outlier generation method that reflects the characteristics of body weight. This study compared the proposed method with the SMOTE-based data augmentation and the GAN-based data augmentation methods. Our results confirm that our proposed method for outlier detection was better than the SVM, XGBOOST, and CATBOOST algorithms. Through them, we can reduce the data imbalance level, improve data quality, and improve analytics accuracy.
更多查看译文
关键词
data generation, data imbalance, machine learning, outlier generation, wearable device, smartwatch, body weight data, lifelog
AI 理解论文
溯源树
样例
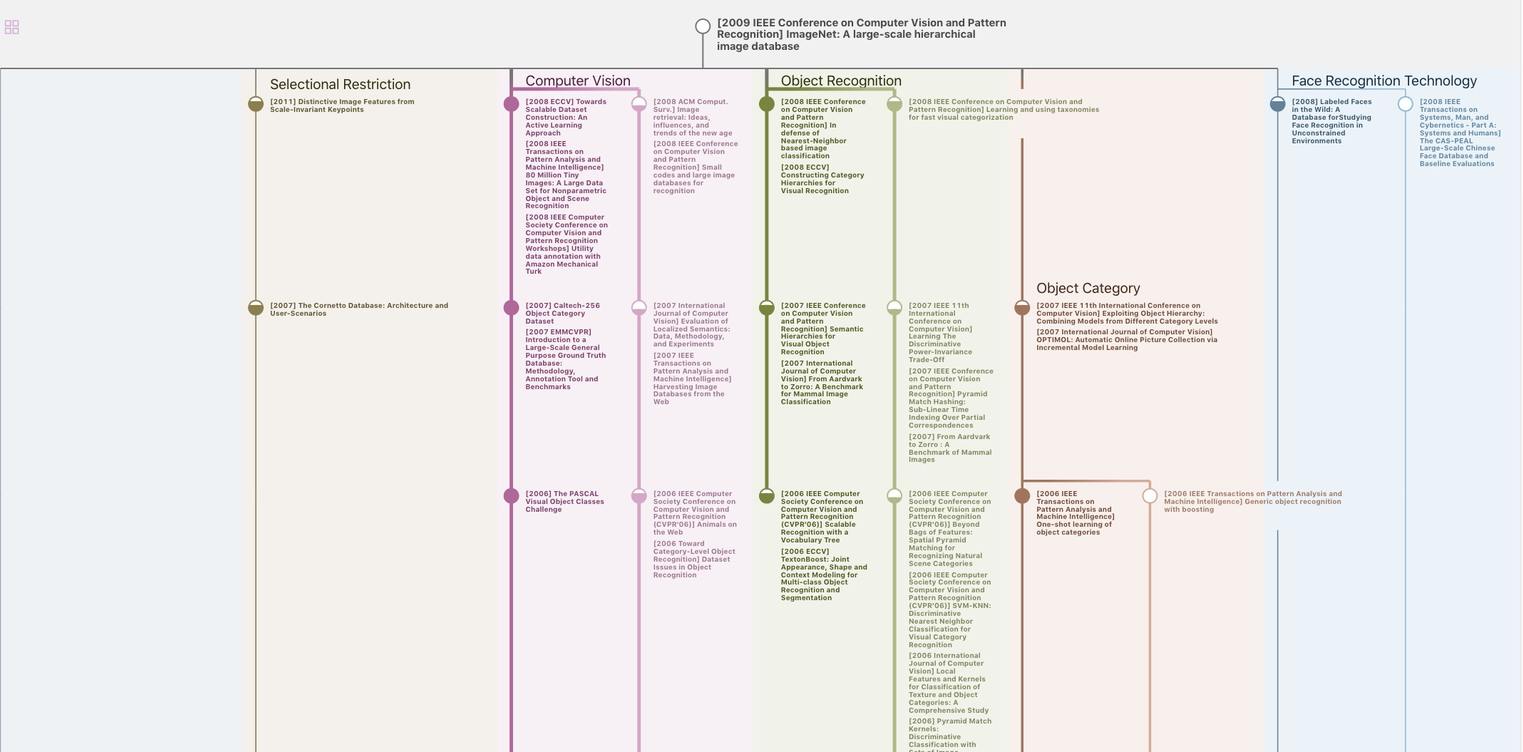
生成溯源树,研究论文发展脉络
Chat Paper
正在生成论文摘要