Assessing the Nonlinear Effect of Atmospheric Variables on Primary and Oxygenated Organic Aerosol Concentration Using Machine Learning
ACS EARTH AND SPACE CHEMISTRY(2022)
摘要
Organic aerosol (OA) accounts for a significant fraction of atmosphericparticulate matter. The OA concentration in the atmosphere is of high variability anddepends on factors such as emission, the atmospheric oxidation process, meteorology,and transport. Due to the complex interactions among the numerous factors, accurateestimation of the effects of target variables on OA concentration is often challenging.Herein, a random forest machine learning algorithm successfully predicted theconcentrations of primary and oxygenated organic aerosol (POA and OOA) at urbanand rural sites in Hong Kong. The random forest model explained more than 80% ofthe observed traffic-POA, cooking-POA, and OOA. In contrast, a multiple linearregression model only explained 30-50% of these OA concentrations. In the randomforest model training process, NOxwas also the most important variable for traffic-POA and cooking-POA. For OOA, multiple parameters were equally crucial in themodel prediction, including NOx,O3, and relative humidity (RH). The dependence ofOA concentrations on atmospheric conditions (e.g., various NOxand O3concentrations and meteorological conditions) wascalculated via the partial dependence algorithm. The results suggested that the dependence of OA concentrations on atmosphericconditions was nonlinear and depended on different condition regimes. The partial dependence algorithm provides insights into thePOA source and OOA formation mechanisms under a complex environment.
更多查看译文
关键词
partial dependence, machine learning, organic aerosol, nonlinear effect, atmospheric variables
AI 理解论文
溯源树
样例
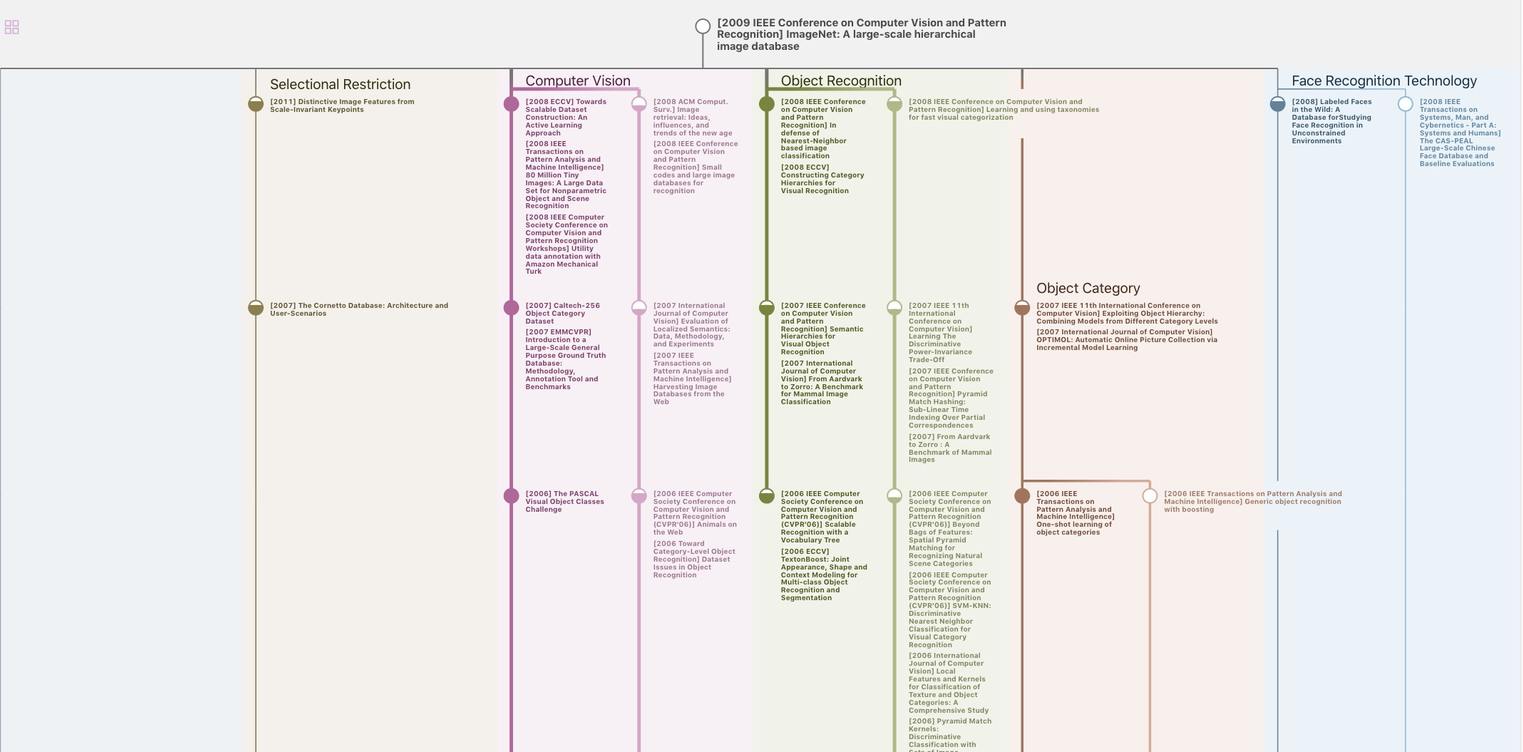
生成溯源树,研究论文发展脉络
Chat Paper
正在生成论文摘要