Machine learning and artificial neural networks to construct P2P lending credit-scoring model: A case using Lending Club data
QUANTITATIVE FINANCE AND ECONOMICS(2022)
摘要
In this study, we constructed the credit-scoring model of P2P loans by using several machine learning and artificial neural network (ANN) methods, including logistic regression (LR), a support vector machine, a decision tree, random forest, XGBoost, LightGBM and 2-layer neural networks. This study explores several hyperparameter settings for each method by performing a grid search and cross-validation to get the most suitable credit-scoring model in terms of training time and test performance. In this study, we get and clean the open P2P loan data from Lending Club with feature engineering concepts. In order to find significant default factors, we used an XGBoost method to pre-train all data and get the feature importance. The 16 selected features can provide economic implications for research about default prediction in P2P loans. Besides, the empirical result shows that gradient-boosting decision tree methods, including XGBoost and LightGBM, outperform ANN and LR methods, which are commonly used for traditional credit scoring. Among all of the methods, XGBoost performed the best.
更多查看译文
关键词
P2P lending, credit score, machine learning, artificial neural networks, feature engineering, Lending Club
AI 理解论文
溯源树
样例
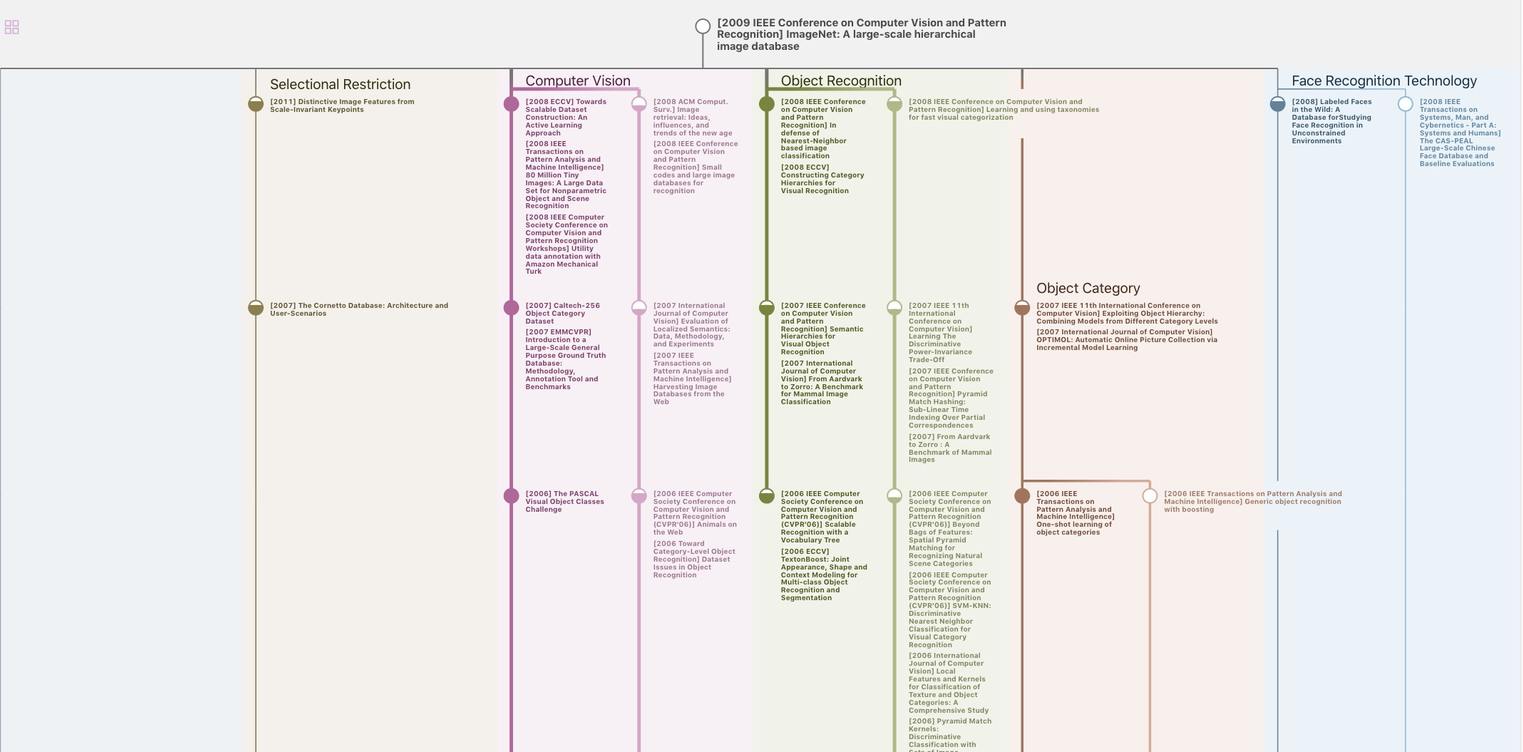
生成溯源树,研究论文发展脉络
Chat Paper
正在生成论文摘要