Learning label-specific features with global and local label correlation for multi-label classification
APPLIED INTELLIGENCE(2022)
摘要
Multi-label algorithms often use an identical feature space to build classification models for all labels. However, labels generally express different semantic information and should have their own characteristics. A few algorithms have been proposed to find label-specific features to construct discriminative classification models. Some use global label correlation to make the reconstructed features more discriminative, but they usually neglect the local correlation between labels. To solve this problem, we propose a new algorithm, named learning Label-specific Features with Global and Local label Correlation (LFGLC). The algorithm integrates both global and local label correlation to extract label-specific features for each label. Specifically, global label correlation is calculated by the label co-occurrence frequency between label pairs, and local label correlation is learned from the neighborhood of each instance. Comprehensive experiments on 12 multi-label data sets clearly manifest that the proposed algorithm performs competitively in feature selection and multi-label classification.
更多查看译文
关键词
Multi-label classification,Label-specific features,Global label correlation,Local label correlation,Feature selection
AI 理解论文
溯源树
样例
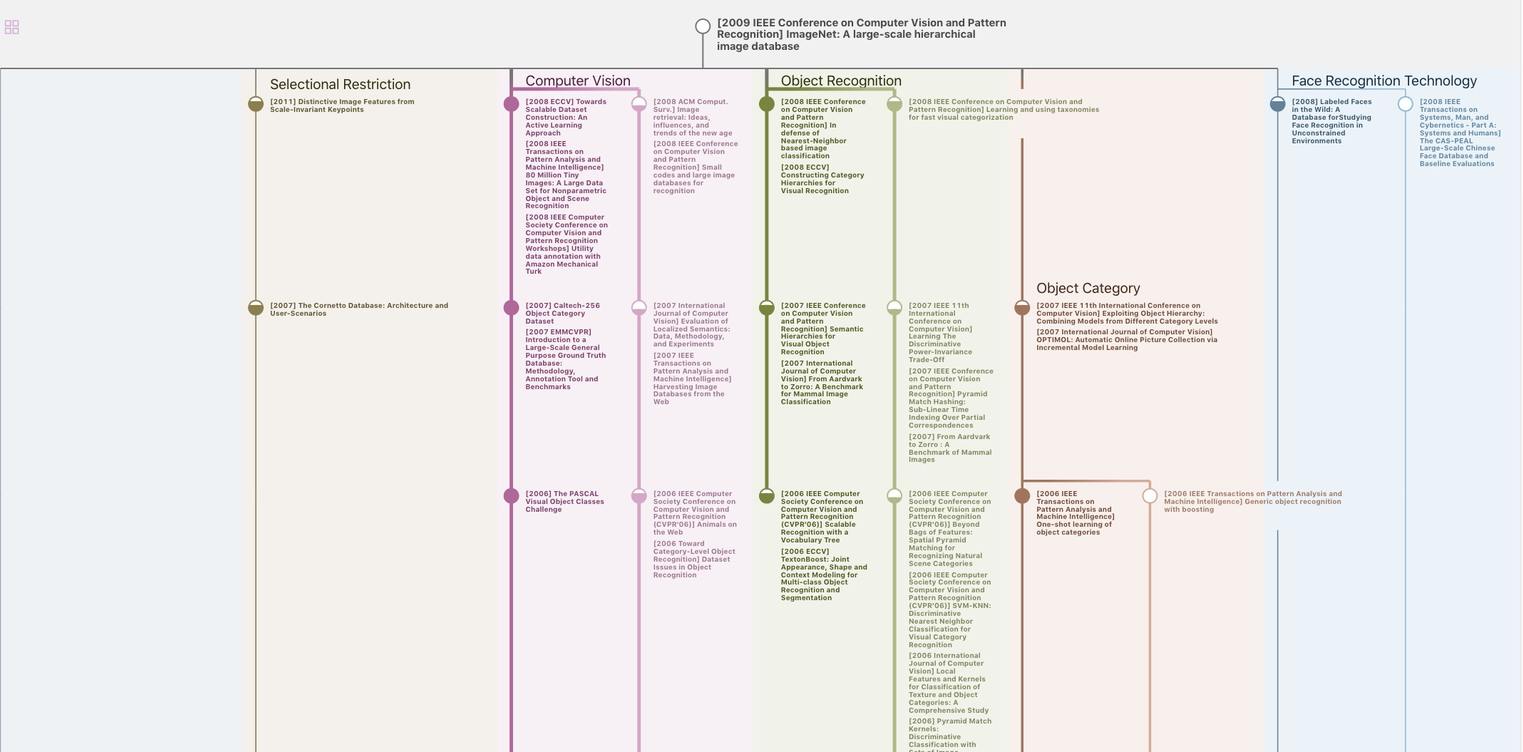
生成溯源树,研究论文发展脉络
Chat Paper
正在生成论文摘要