Lithologic classification of pyroclastic rocks: A case study for the third member of the Huoshiling Formation, Dehui fault depression, Songliao Basin, NE China
Journal of Petroleum Science and Engineering(2022)
摘要
With the ongoing discovery of volcanic oil and gas reservoirs, pyroclastic rocks have begun to attract widespread attention from both the academic and industrial sectors. Because there is more information about the cementation mode and the grain size in the Chinese classification system for pyroclastic rocks than there is in the system used throughout the rest of the world, we use the Chinese classification system to investigate the lithologies of the pyroclastic rocks in our study area. The three cementation modes dictate the three classes of pyroclastic rock: pyroclastic lava, normal pyroclastic rock, and sedimentary pyroclastic rock. Within these three modes, pyroclastic rocks are further classified by their clastic material grain size into agglomerate, breccia, and tuff. If the pyroclastic material is moved by aeolian transport, it tends to follow the positive sedimentation cycle sequence. In this study, we classify the pyroclastic rocks from the third member of the Huoshiling Formation (Fm) in the Dehui fault depression in the southern Songliao Basin. Draw the cross plots of pyroclastic lava, normal pyroclastic rock and sedimentary pyroclastic rock in the study area. Based on core date, fullbore micro-resistivity images (FMIs), and thin section analyses, we determine the mineral composition, particle size, and cementation mode of breccia bearing tuff lava, tuff lava, breccia bearing welded tuff, volcanic breccia, breccia bearing tuff, tuff, sedimentary volcanic breccia, and sedimentary tuff. Our results indicate that the decision tree method does not require all of the input curves to identify a given sample's lithology. We also found that adding more data to a support vector machine (SVM) does not necessarily translate into more accurate classification results. This classification is undertaken using two different techniques: a combination of elemental capture spectroscopy (ECS) cross plots and FMI and a SVM learning algorithm with information derived from node information in a decision tree. Ultimately, the results produced by the SVM algorithm are more accurate (recognition accuracy of 98.6%) than those generated by the ECS/FMI approach. Furthermore, the SVM algorithm requires less data and operates very efficiently. Using data from multiple wells allows for better and more rapid identification of the lithology type in the target layer; large observational or training datasets provide a strong foundation for further reservoir evaluation.
更多查看译文
关键词
Pyroclastic rock,Dehui fault depression,Lithology classification,Machine learning,Cross plots,Logging identification
AI 理解论文
溯源树
样例
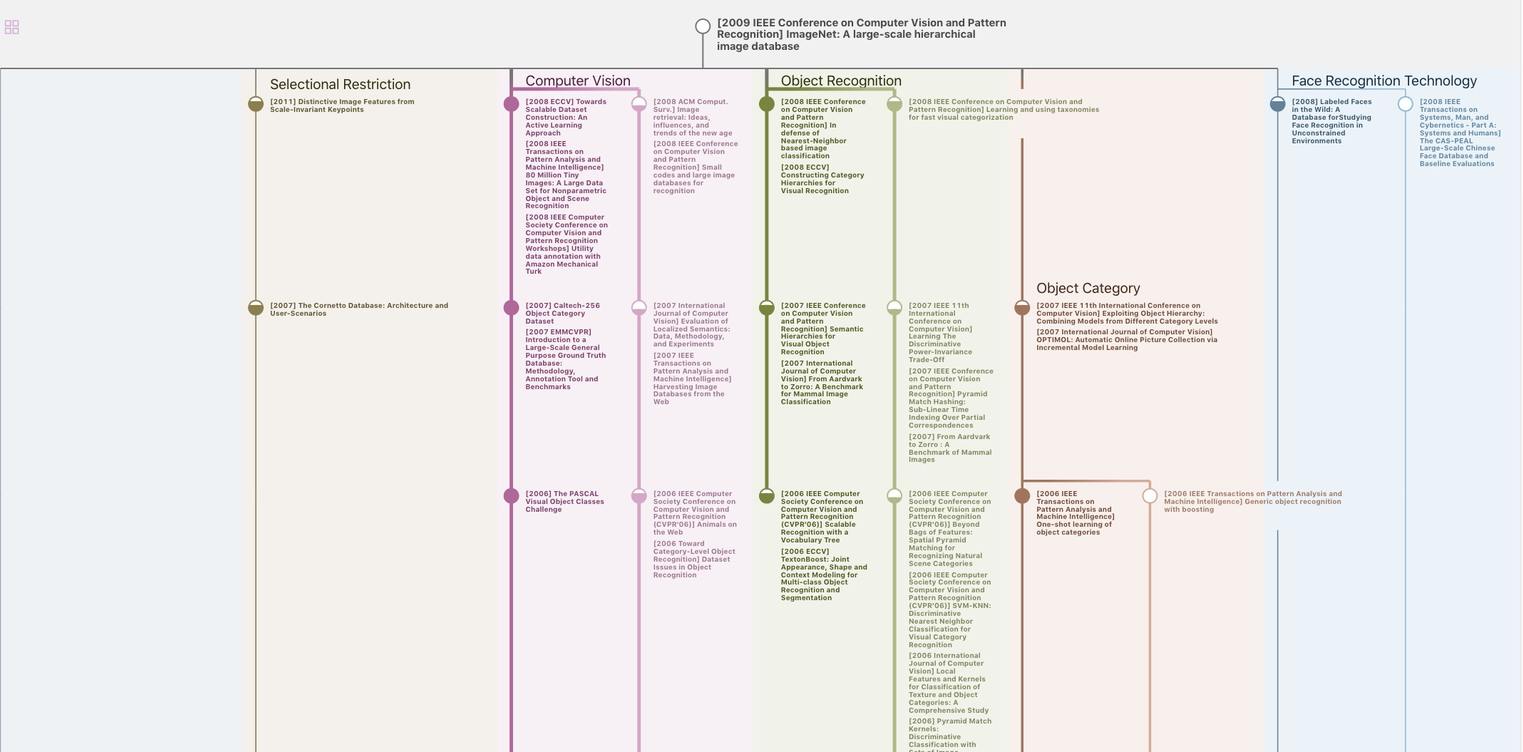
生成溯源树,研究论文发展脉络
Chat Paper
正在生成论文摘要