A Computational Methodology for Estimating the Detected Energy Spectra of the Gamma-Ray Flux From Irradiated Nuclear Fuel
IEEE Transactions on Nuclear Science(2022)
摘要
Gamma-ray spectrometry using collimated detectors is a well-established examination method for irradiated nuclear fuel. However, the feasibility of examining a particular nuclide of interest is subject to constraints; the peak must be statistically determinable with the desired precision, and the total spectrum count rate in the detector should not cause throughput issues. Methods were assembled for gamma spectrum prediction to optimize instruments for gamma emission tomography and to enable
a priori
feasibility evaluation of determination of single peaks of irradiated nuclear fuel. The aim was to find reliable results (~10% accuracy) regarding total spectrum and peak count rates with faster computation time than a full-Monte Carlo approach. For this purpose, the method is based on depletion calculations with SERPENT2, a point-source kernel method for the collimator response, and a rig response matrix and a detector response matrix, both computed with MCNP6. The computational methodology uses the fuel properties (dimensions, materials, power history, and cooling time) and the instrumental setup (collimator and detector dimensions and materials) as an input. The prediction method was validated using the measured data from a high-burnup, short-cooled test fuel rodlet from the Halden reactor. Absolute count rates and ratios of characteristic peaks were compared between predicted and measured spectra, showing a total count rate overestimation of 7% and discrepancies between 2% and 20% for the single peaks (the same order of magnitude of the uncertainty). This level of agreement is deemed sufficient for measurement campaigns planning and the optimization of spectroscopic instruments for use in gamma scanning and tomography of nuclear fuel.
更多查看译文
关键词
Gamma emission tomography (GET),gamma spectroscopy,nuclear fuel inspection,post irradiation examination (PIE)
AI 理解论文
溯源树
样例
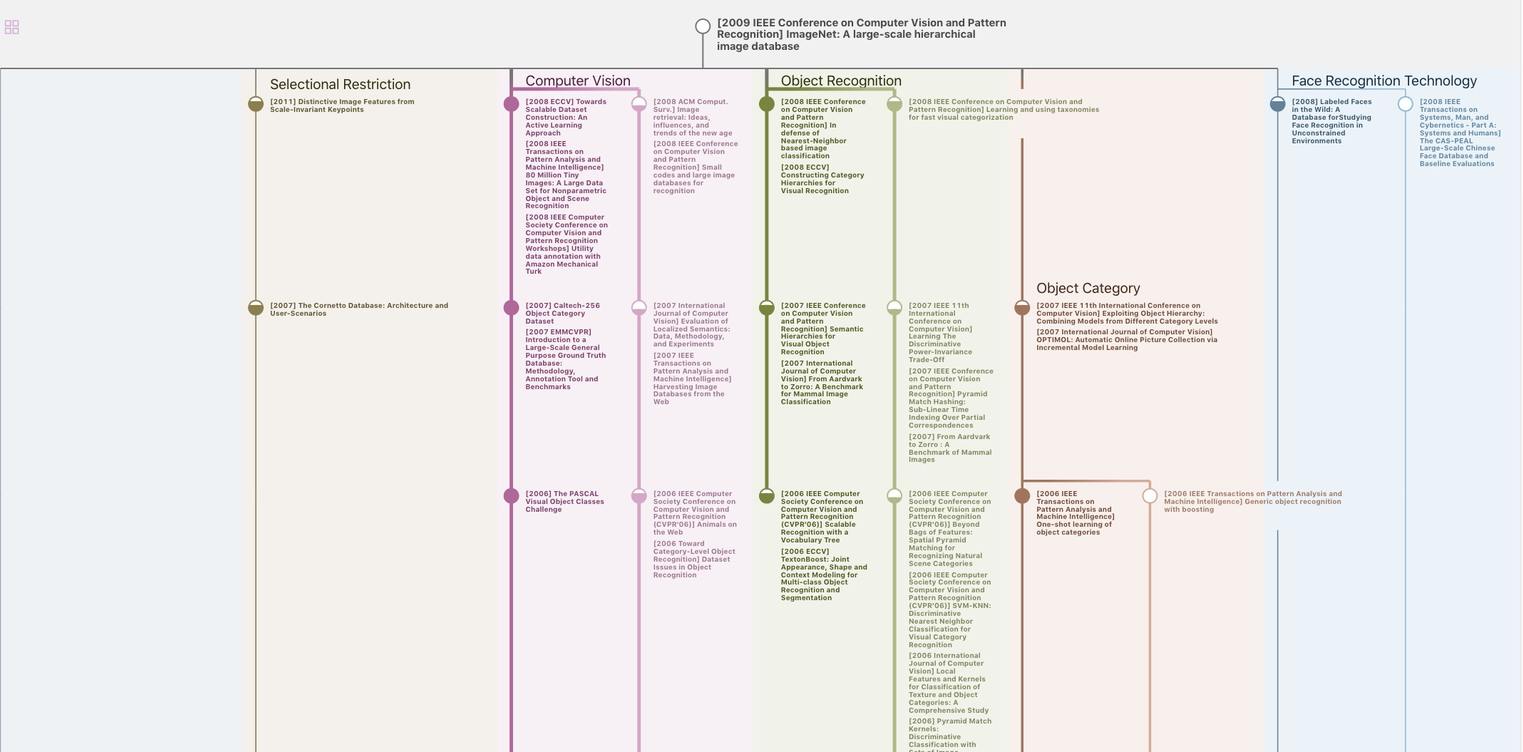
生成溯源树,研究论文发展脉络
Chat Paper
正在生成论文摘要