Sparse Integration Schemes for Chance-Constrained Motion Primitive Path Planning
IEEE Robotics and Automation Letters(2022)
摘要
Expected value computations are a key bottleneck for path planning under uncertainty. Computing an expected value requires integrating over the uncertainty domain, which may be high dimensional. One way to accelerate such calculations is through the use of a “sparse” numerical integration scheme. This work presents a method for obtaining maximally sparse numerical integration schemes for use with hypergraph search algorithms for path planning problems under parametric uncertainty. The approach formulates a mixed-integer linear program that is tailored to the specific structure of the hypergraph on which chance-constrained motion primitive planning problems are solved. The optimization is solved offline, yielding a sparse integration scheme that can then be used for a variety of planning tasks. Results demonstrate that the sparse schemes maintain the estimation accuracy of the original formulation while requiring dramatically less computation time.
更多查看译文
关键词
Collision avoidance,optimization and optimal control,planning under uncertainty
AI 理解论文
溯源树
样例
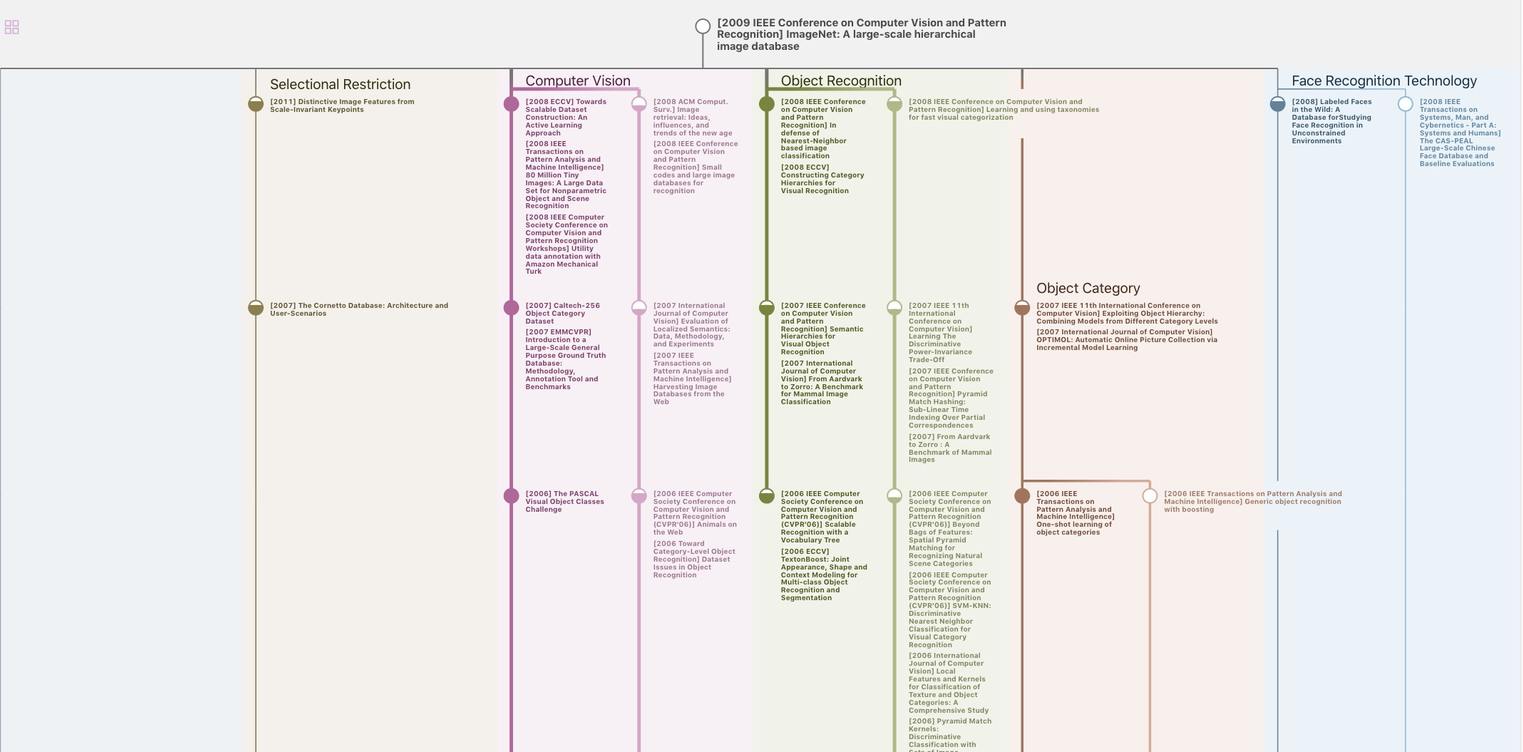
生成溯源树,研究论文发展脉络
Chat Paper
正在生成论文摘要