A multi-head attention network with adaptive meta-transfer learning for RUL prediction of rocket engines
Reliability Engineering & System Safety(2022)
摘要
Accurate prediction of remaining useful life (RUL) is necessary to ensure stable and safe operations for rocket engines. The paper proposed a multi-head attention network coupled with adaptive meta-transfer learning for RUL prediction. By combining the convolution-based branch with an attention-based branch, the multi-head attention network is proposed for accurate RUL prediction of cryogenic bearings in rocket engines under the steady stage. In addition, an adaptive model-agnostic meta-transfer learning strategy is developed to further improve the performance under small sample circumstances with adaptive hyper-parameters. To demonstrate the superiority, the proposed method is compared with typical benchmark algorithms using real monitoring data from a high-precision cryogenic rocket engine experiment platform. Results indicate that the proposed method achieves better performance compared with existing models under multiple evaluation indexes.
更多查看译文
关键词
Remaining useful life,Deep neural network,Rocket engine,Transfer learning
AI 理解论文
溯源树
样例
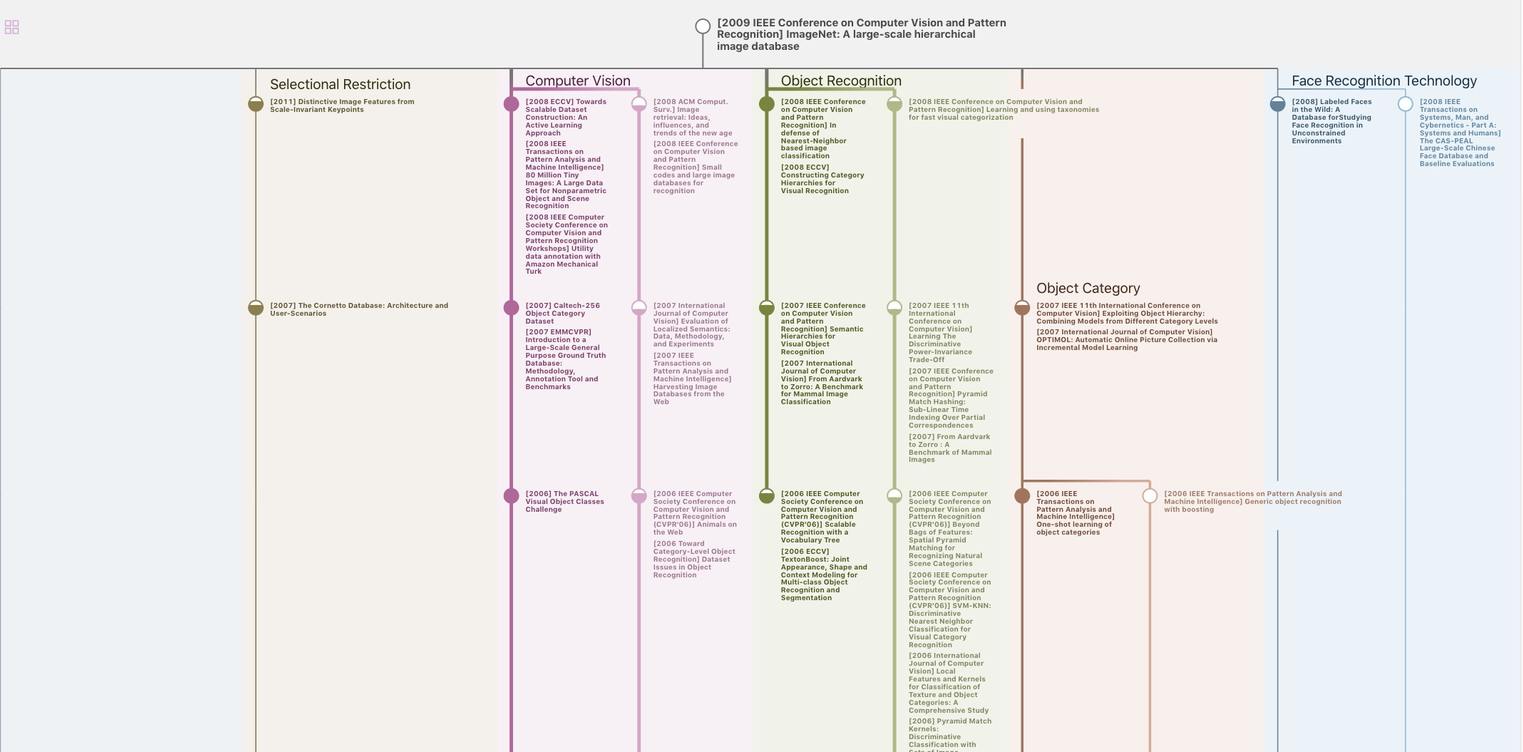
生成溯源树,研究论文发展脉络
Chat Paper
正在生成论文摘要