Electroglottograph-Based Speech Emotion Recognition via Cross-Modal Distillation
APPLIED SCIENCES-BASEL(2022)
摘要
Speech emotion recognition (SER) is an important component of emotion computation and signal processing. Recently, many works have applied abundant acoustic features and complex model architectures to enhance the model's performance, but these works sacrifice the portability of the model. To address this problem, we propose a model utilizing only the fundamental frequency from electroglottograph (EGG) signals. EGG signals are a sort of physiological signal that can directly reflect the movement of the vocal cord. Under the assumption that different acoustic features share similar representations in the internal emotional state, we propose cross-modal emotion distillation (CMED) to train the EGG-based SER model by transferring robust speech emotion representations from the log-Mel-spectrogram-based model. Utilizing the cross-modal emotion distillation, we achieve an increase of recognition accuracy from 58.98% to 66.80% on the S70 subset of the Chinese Dual-mode Emotional Speech Database (CDESD 7-classes) and 32.29% to 42.71% on the EMO-DB (7-classes) dataset, which shows that our proposed method achieves a comparable result with the human subjective experiment and realizes a trade-off between model complexity and performance.
更多查看译文
关键词
speech emotion recognition, knowledge distillation, cross-modal transfer, electroglottograph
AI 理解论文
溯源树
样例
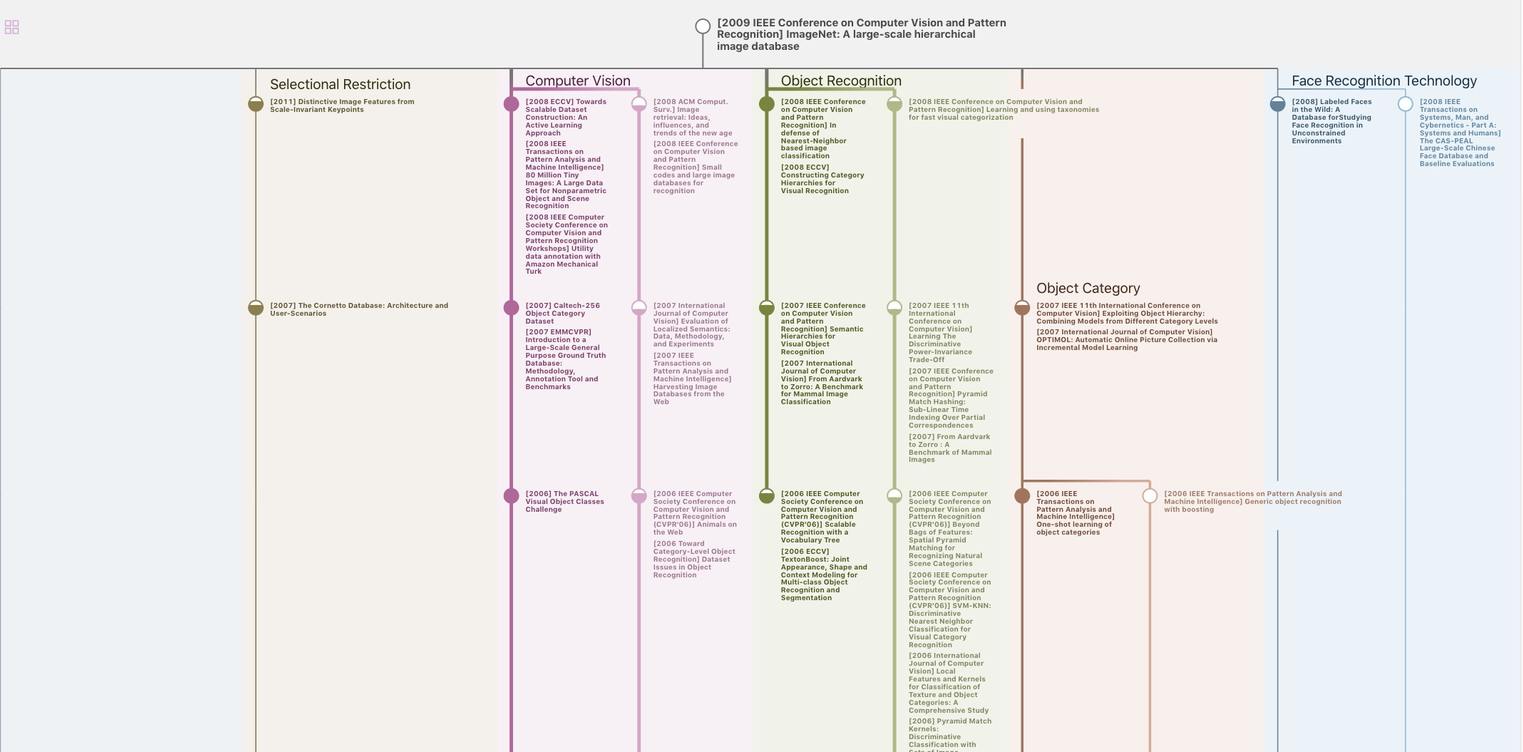
生成溯源树,研究论文发展脉络
Chat Paper
正在生成论文摘要