Incorporating spatial uncertainty maps into soil sampling improves digital soil mapping classification accuracy in Ontario, Canada
Geoderma Regional(2022)
摘要
Digital soil mapping combines soil plot data with environmental datasets to model variation in soil properties across a landscape. The quality of a digital soil map depends on both the quantity and distribution of soil plots within the study extent. Field campaigns to acquire soil data are time intensive and costly to undertake, requiring training and deployment of field crews and soil processing/analytical costs. Therefore, it is important to optimize site selection and sampling intensity to maximize digital soil map accuracy and minimize field costs. In many cases, soil sampling occurs across several years to gather sufficient soil data. Between successive field campaigns, preliminary digital soil maps and their corresponding uncertainty estimates can be generated. We hypothesize that preliminary uncertainty maps can be useful to guide sampling in subsequent field seasons by targeting areas of high uncertainty to significantly improve model accuracy. This hypothesis was tested by simulating a multi-year soil sampling campaign using an extensive soil moisture regime and soil texture dataset from the Hearst Forest in northeastern Ontario, Canada. We quantified how soil maps and soil models changed as new data points were added and how model/map improvement was influenced by performing additional sampling in areas of high uncertainty. We used multiple uncertainty metrics (Ignorance Uncertainty, Exaggeration Uncertainty and Confusion Index) and tested multiple levels of sampling intensity. The results showed modest but statistically significant improvements in model accuracy when subsequent sampling was targeted in high uncertainty areas (treatments) compared to sampling in random areas (controls) (38.7% control accuracy compared to 39.8%/40.4%/40.3% for moisture regime and 23.1% control accuracy compared to 24.3%/25%/24.9% for textural class). There were no significant differences in model performance between the three uncertainty metrics. The most common textural and moisture regime classes in the soil dataset rarely occurred in areas of high uncertainty suggesting that the environmental covariates used in the study tracked real soil variation. As subsequent sampling intensity increased, model performance increased as well (both in the control and treatment groups). There was also a significant treatment × sampling intensity interaction meaning that uncertainty guided sampling was increasingly beneficial as sampling effort increased. This paper demonstrates a proof of concept that generating preliminary uncertainty maps in digital soil mapping can be a useful tool for informing future field soil sample collections to improve model performance.
更多查看译文
关键词
Digital soil mapping,Uncertainty,Adaptive sampling,Spodosols,Luvisols
AI 理解论文
溯源树
样例
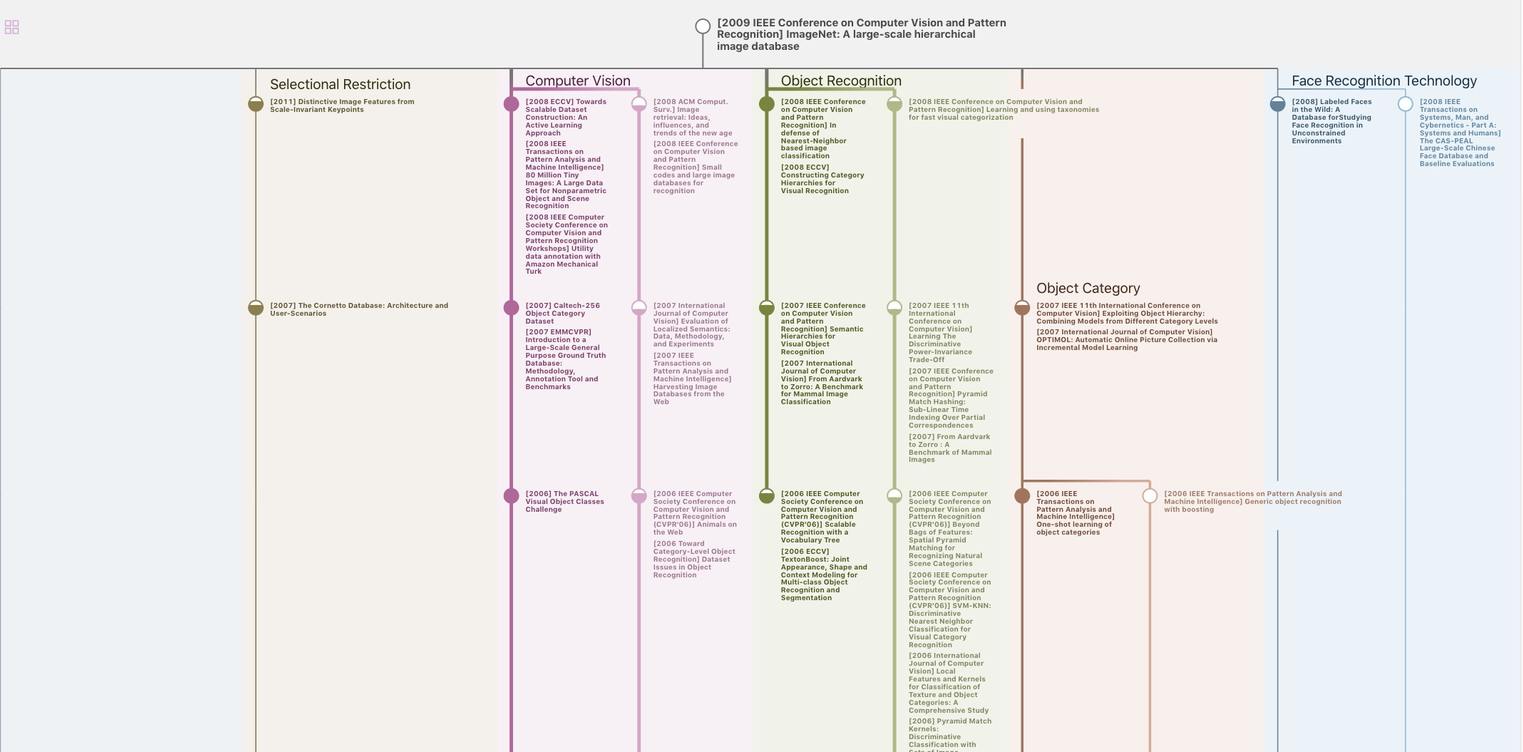
生成溯源树,研究论文发展脉络
Chat Paper
正在生成论文摘要