Graph neural network and multi-agent reinforcement learning for machine-process-system integrated control to optimize production yield
Journal of Manufacturing Systems(2022)
摘要
In this paper, an integrated control framework is proposed for the optimization of the production yield by integrating different levels of a manufacturing system, including system, process, and machine levels. The manufacturing system is modeled as a graph by treating machines as nodes and material flows as links. The graph model enjoys high flexibility and is able to incorporate all relevant real-time information across all levels of the manufacturing system in the dynamic node feature. Since the real-time tool state is essential for decision making, Recursive Bayesian Estimation (RBE) is adopted to reduce the tool state observations through sensors and machine learning models and provide more accurate tool state estimation to be included into the graph node feature. With the graph model, Graph Neural Network (GNN) is applied to process the node features to generate node embedding that reflects both local and global information. For the integrated control purpose, each machine node is then be treated as a distributed agent in Multi-Agent Reinforcement Learning (MARL) that conditions its policy on the node embedding from GNN. State-of-the-art GNN and MARL algorithms, namely Graph Attention Network (GAT) and Value Decomposition Actor Critic (VDAC), are implemented to train learnable parameters in GNN-MARL networks to learn the optimal multi-agent policy. Extensive numerical experiments and analysis proves the effectiveness of the proposed integrated control framework.
更多查看译文
关键词
Integrated control,Manufacturing system graph,Graph neural network,Multi-agent reinforcement learning,Production yield optimization
AI 理解论文
溯源树
样例
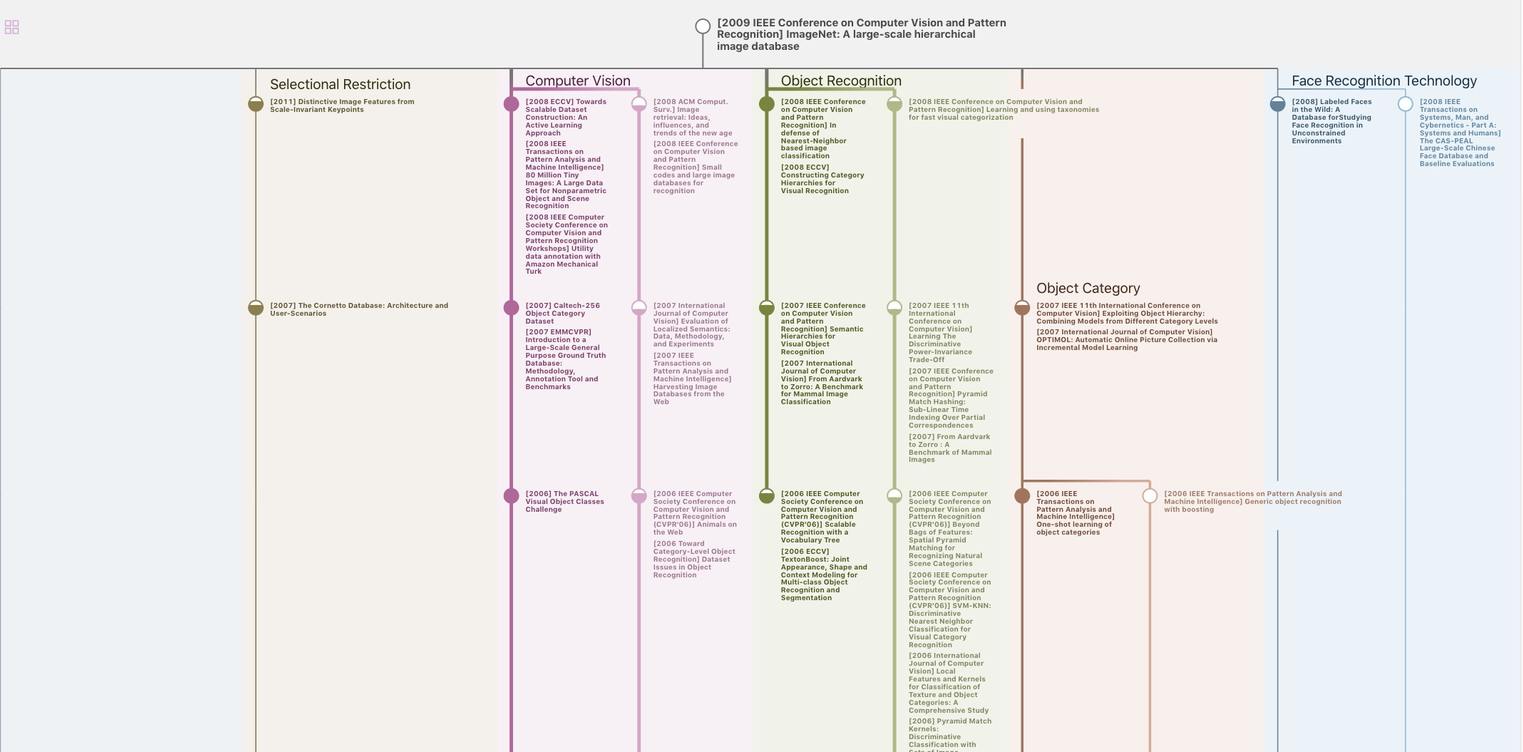
生成溯源树,研究论文发展脉络
Chat Paper
正在生成论文摘要