Single-channel EEG automatic sleep staging based on transition optimized HMM
MULTIMEDIA TOOLS AND APPLICATIONS(2022)
摘要
Sleep staging is a key process for evaluating sleep quality and diagnosing somnipathy-related diseases. Psychologists are required to do the traditional sleep stages identification. The manual work by these experts is often time-consuming and error-prone. In order to improve the performance of such a process, automatic Electroencephalography (EEG) signal analysis using machine learning approaches is often used. In this paper, a transitions-optimized Hidden Markov Model (HMM) model is proposed to improve the accuracy of prediction. Our proposed framework includes 4 key modules: feature extraction, feature selection, classification, and transition optimization. By applying, transition optimization process after a general GMM-HMM classification, our experimental results show a convincing improvement in the accuracy of classification.
更多查看译文
关键词
Sleep staging, Maximum information coefficient, Electroencephalography, Hidden Markov model
AI 理解论文
溯源树
样例
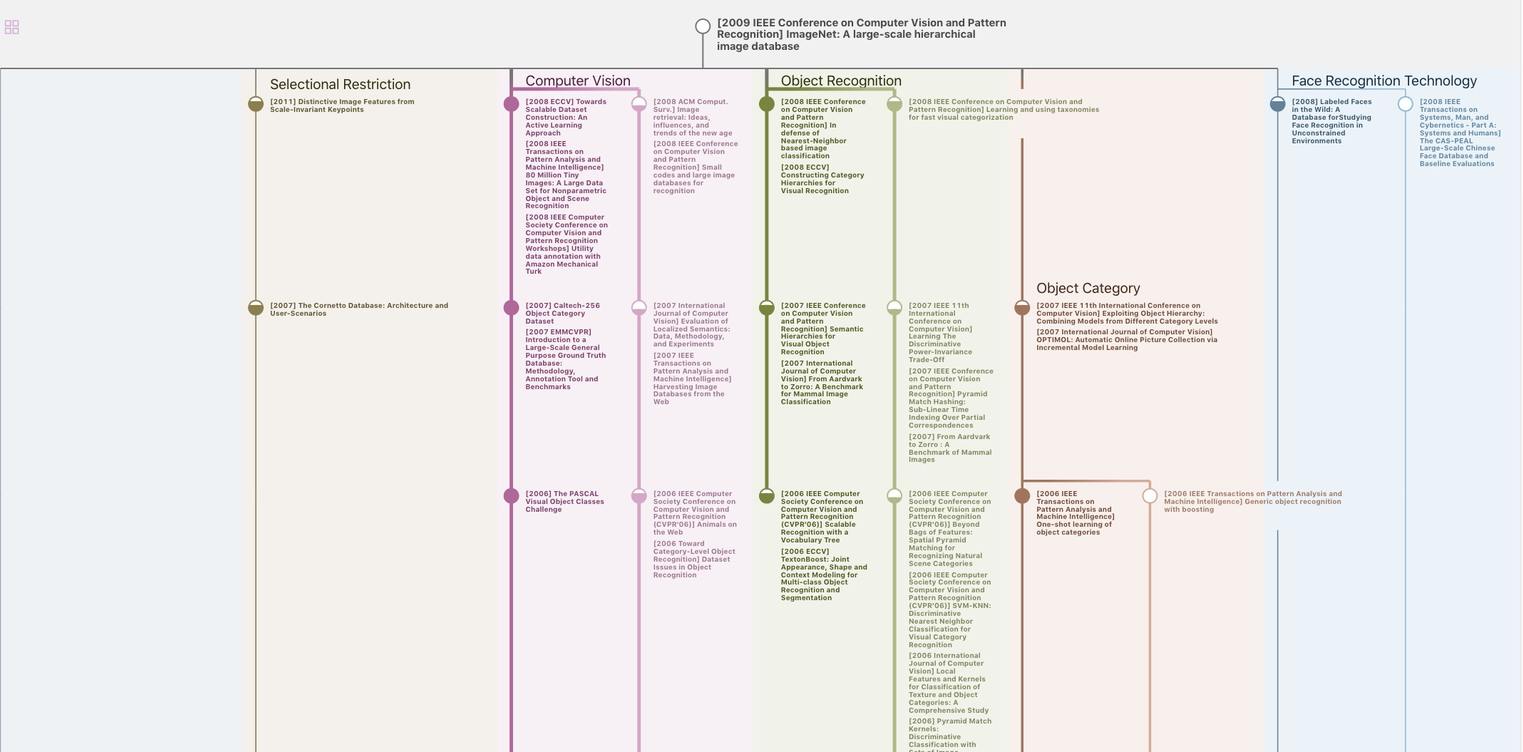
生成溯源树,研究论文发展脉络
Chat Paper
正在生成论文摘要