Learning Scale-Consistent Attention Part Network for Fine-Grained Image Recognition
IEEE TRANSACTIONS ON MULTIMEDIA(2022)
摘要
Discriminative region localization and feature learning are crucial for fine-grained visual recognition. Existing approaches solve this issue by attention mechanism or part based methods while neglecting consistency between attention and local parts, as well as the rich relation information among parts. This paper proposes a Scale-consistent Attention Part Network (SCAPNet) to address that issue, which seamlessly integrates three novel modules: grid gate attention unit (gGAU), scale-consistent attention part selection (SCAPS), and part relation modeling (PRM). The gGAU module represents the grid region at a certain fine-scale with middle layer CNN features and produces hard attention maps with the lightweight Gumbel-Max based gate. The SCAPS module utilizes attention to guide part selection across multi-scales and keep the selection scale-consistent. The PRM module utilizes the self-attention mechanism to build the relationship among parts based on their appearance and relative geo-positions. SCAPNet can be learned in an end-to-end way and demonstrates state-of-the-art accuracy on several publicly available fine-grained recognition datasets (CUB-200-2011, FGVC-Aircraft, Veg200, and Fru92).
更多查看译文
关键词
Image recognition, Task analysis, Logic gates, Location awareness, Visualization, Training, Object detection, Fine-grained image recognition, scale-consistent, attention part
AI 理解论文
溯源树
样例
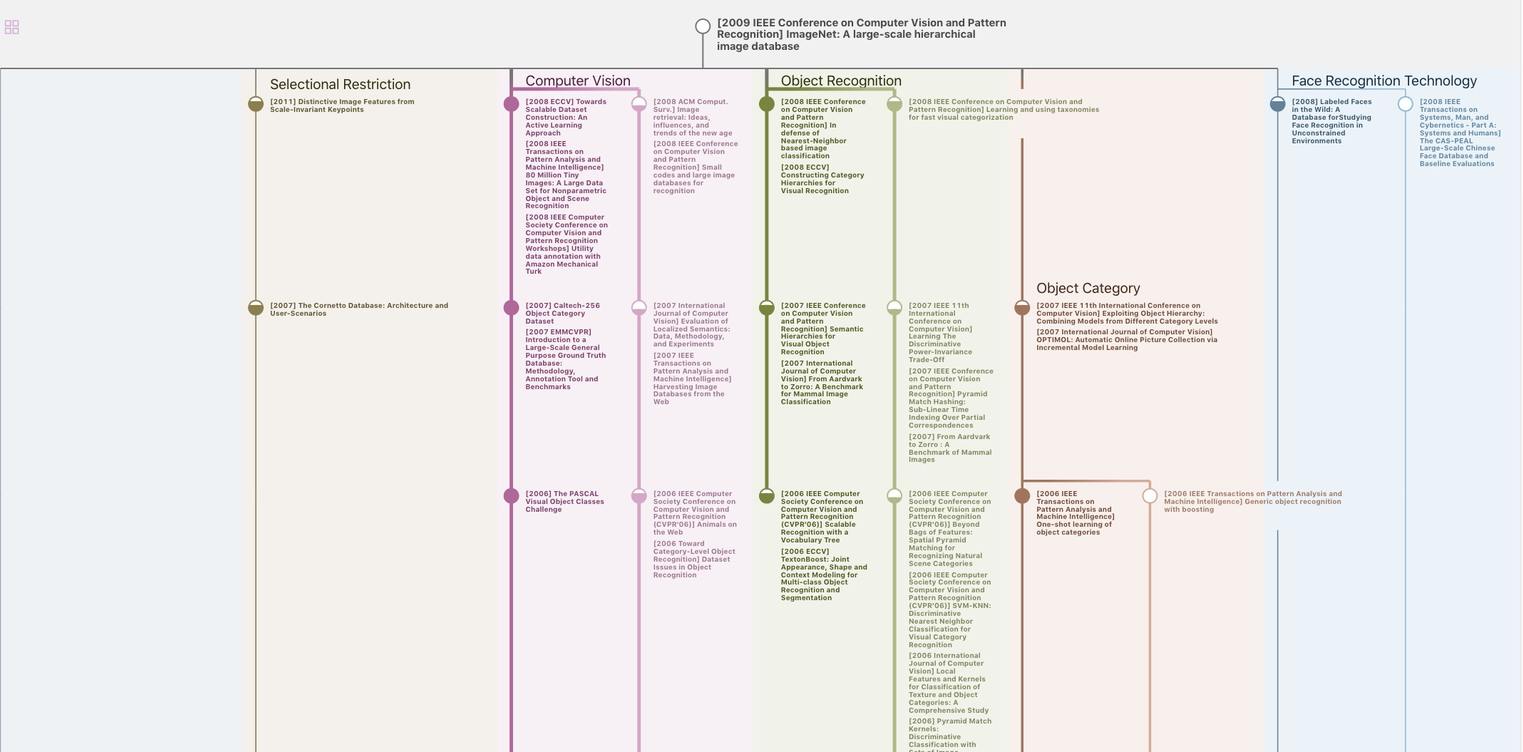
生成溯源树,研究论文发展脉络
Chat Paper
正在生成论文摘要