A Novel Machine Learning-Based Approach for Induction Machine Fault Classifier Development-A Broken Rotor Bar Case Study
ENERGIES(2022)
摘要
Rotor bars are one of the most failure-critical components in induction machines. We present an approach for developing a rotor bar fault identification classifier for induction machines. The developed machine learning-based models are based on simulated electrical current and vibration velocity data and measured vibration acceleration data. We introduce an approach that combines sequential model-based optimization and the nested cross-validation procedure to provide a reliable estimation of the classifiers' generalization performance. These methods have not been combined earlier in this context. Automation of selected parts of the modeling procedure is studied with the measured data. We compare the performance of logistic regression and CatBoost models using the fast Fourier-transformed signals or their extracted statistical features as the input data. We develop a technique to use domain knowledge to extract features from specific frequency ranges of the fast Fourier-transformed signals. While both approaches resulted in similar accuracy with simulated current and measured vibration acceleration data, the feature-based models were faster to develop and run. With measured vibration acceleration data, better accuracy was obtained with the raw fast Fourier-transformed signals. The results demonstrate that an accurate and fast broken rotor bar detection model can be developed with the presented approach.
更多查看译文
关键词
broken rotor bar, condition monitoring, fault classification, feature extraction, induction machine, machine learning, predictive maintenance, supervised learning
AI 理解论文
溯源树
样例
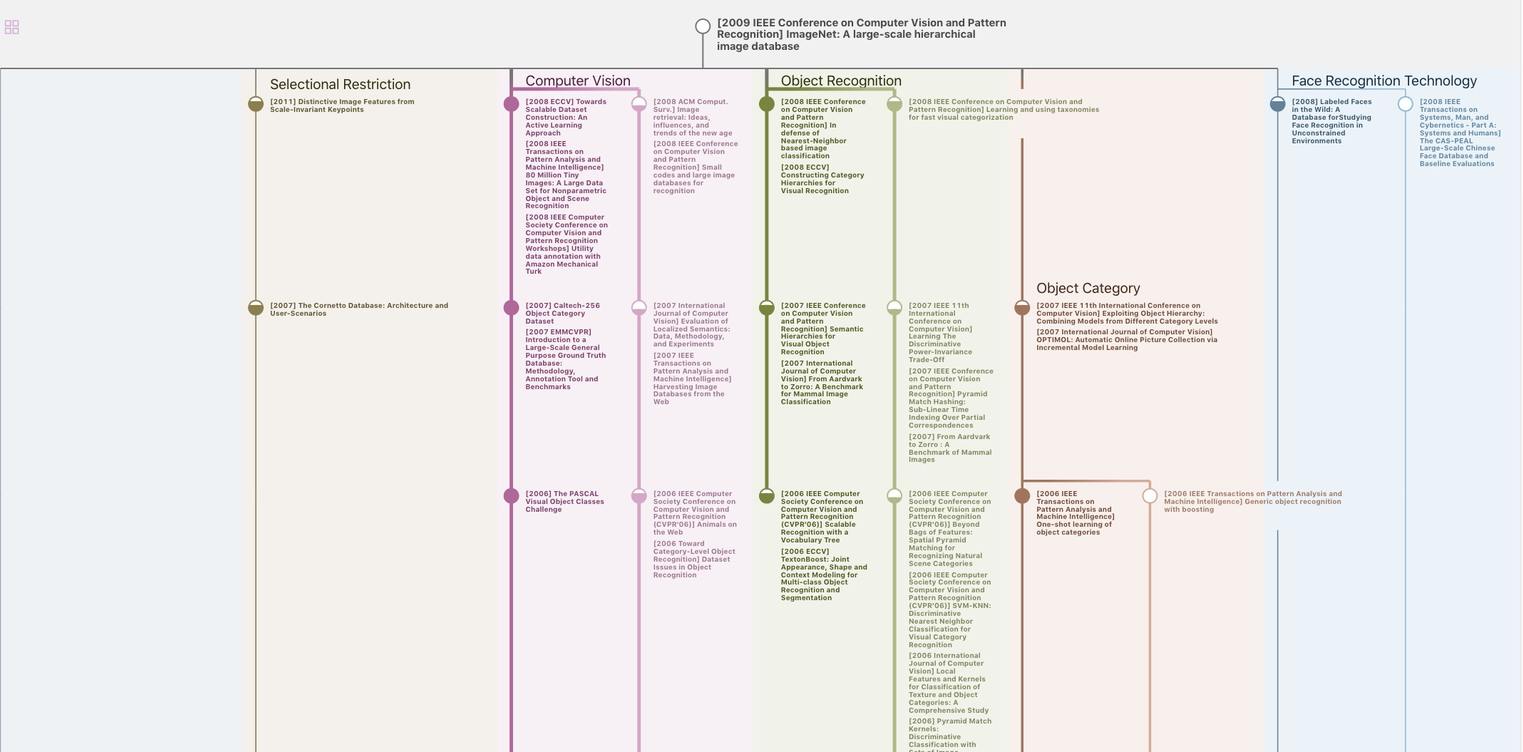
生成溯源树,研究论文发展脉络
Chat Paper
正在生成论文摘要