Non-Technical Losses Detection Using Autoencoder and Bidirectional Gated Recurrent Unit to Secure Smart Grids
IEEE ACCESS(2022)
摘要
Electricity theft is considered one of the most significant reasons of the non technical losses (NTL). It negatively influences the utilities in terms of the power supply quality, grid's safety, and economic loss. Therefore, it is necessary to effectively deal with the electricity theft problem. For detecting electricity theft in smart grids (SGs), an efficient and state-of-the-art approach is designed in the underlying work based on autoencoder and bidirectional gated recurrent unit (AE-BiGRU). The proposed approach consists of six components: (1) data collection, (2) data preparation, (3) data balancing, (4) feature extraction, (5) classification and (6) performance evaluation. Moreover, bidirectional gated recurrent unit (BiGRU) is used for the identification of the anomalies in electricity consumption (EC) patterns caused due to factors like family formation changes, holidays, parties, and so on, which are referred as non-theft factors. The proposed autoencoder-bidirectional gated recurrent unit (AE-BiGRU) model employs the EC data acquired from state grid corporation of China (SGCC) for simulations. Furthermore, it is visualized from the simulation results that 90.1% accuracy and 10.2% false positive rate (FPR) are obtained by the proposed model. The results are better than different existing classifiers, i.e., logistic regression (LR), decision tree (DT), extreme gradient boosting (XGBoost), gated recurrent unit (GRU), etc.
更多查看译文
关键词
Data models, Support vector machines, Logic gates, Hardware, Feature extraction, Convolutional neural networks, Meters, Autoencoder, bidirectional gated recurrent unit, deep learning algorithms, smart grid, electricity theft detection, machine learning algorithms, synthetic theft attacks
AI 理解论文
溯源树
样例
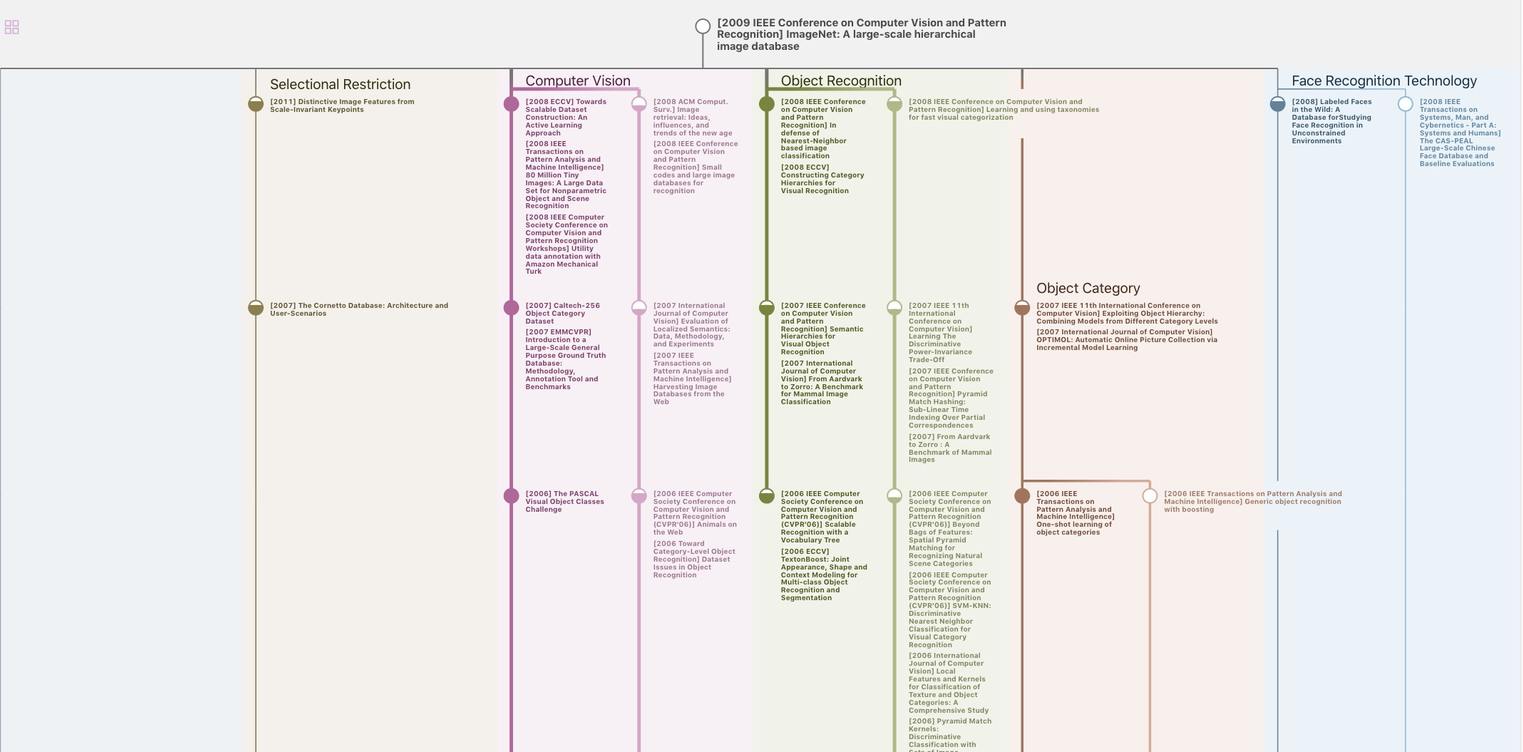
生成溯源树,研究论文发展脉络
Chat Paper
正在生成论文摘要