Novel online discriminant analysis based schemes to deal with observations from known and new classes: Application to industrial systems
Engineering Applications of Artificial Intelligence(2022)
摘要
In industrial systems, continuous monitoring of process conditions is crucial. Existing discriminant analysis methods, including quadratic discriminant analysis (QDA), Fisher discriminant analysis (FDA), exponential discriminant analysis (EDA), orthonormal discriminant vector (ODV) and kernel Fisher discriminant (KFD), have been widely used in fault classification literature. Although efficient in controlled environment, they may fail in practice as they discriminate through a closed space. They cannot recognize observations that do not belong to their training set. In this paper, we propose statistical subspaces to allow these methods to efficiently deal with unseen observations of unknown classes. Traditional discriminant functions are summarized, and then new decision schemes are presented. Continuous stirred tank reactor (CSTR) and Tennessee Eastman process (TEP) evaluated the performance of the proposed approach and applied it on five popular methods. The results show that the new space has superior performances in term of discrimination between known and unknown faults.
更多查看译文
关键词
Open set classification,Discriminant analysis,Statistic test
AI 理解论文
溯源树
样例
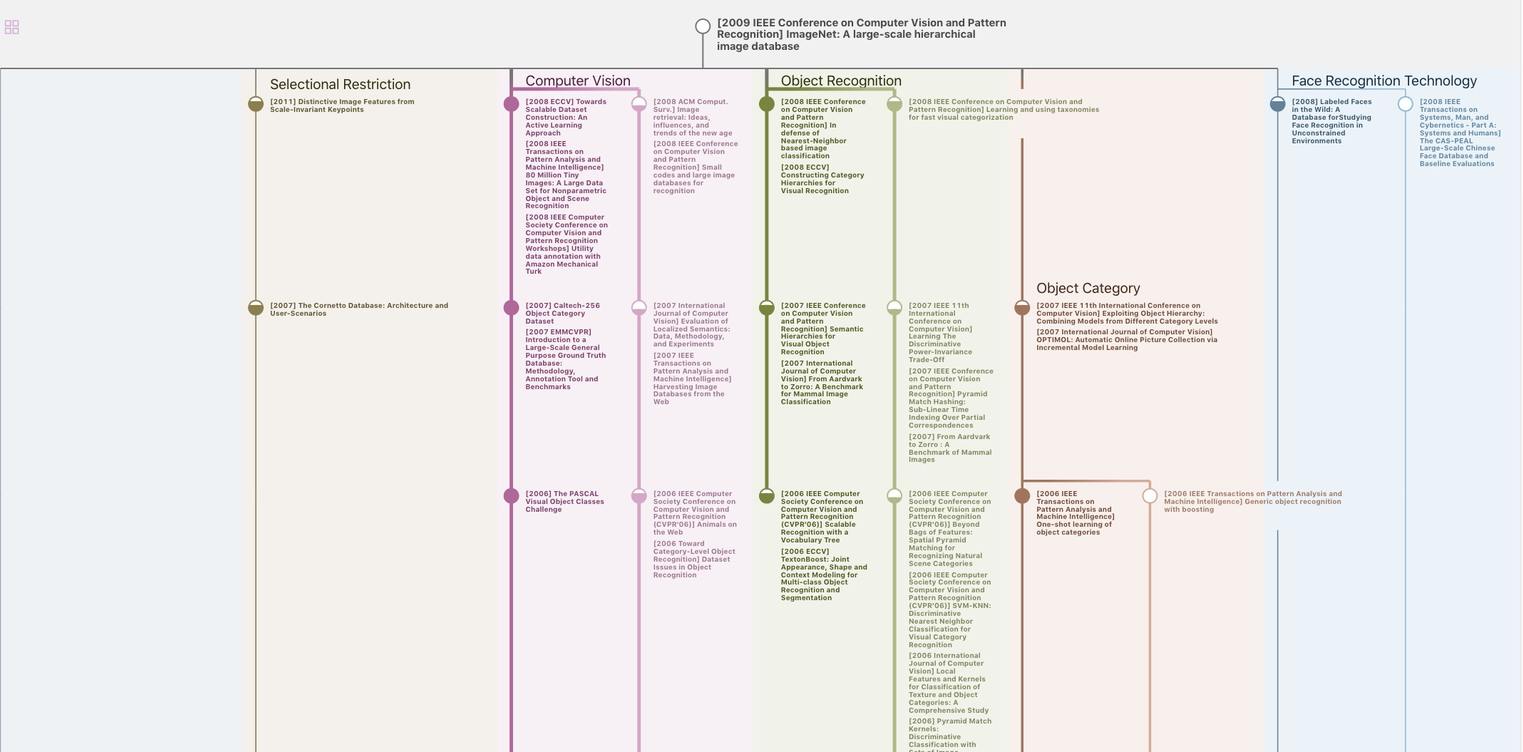
生成溯源树,研究论文发展脉络
Chat Paper
正在生成论文摘要